Graph Comparison for Causal Discovery
Joseph Cottam, Maria Glenski, Zhuanyi Huang, Ryan Rabello, Austin Golding, Svitlana Volkova, Dustin L Arendt
View presentation:2021-10-24T14:40:00ZGMT-0600Change your timezone on the schedule page
2021-10-24T14:40:00Z
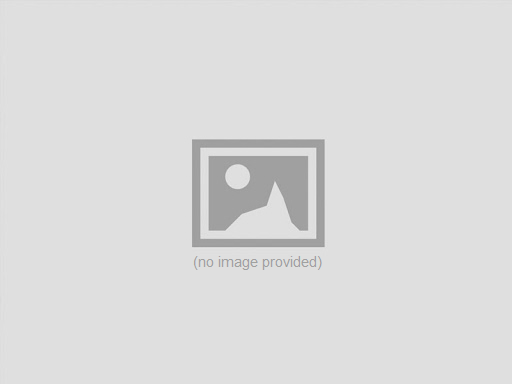
Abstract
Reasoning about cause and effect is one of the frontiers for modern machine learning. Many causality techniques reason over a ``causal graph'' provided as input to the problem. When a causal graph cannot be produced from human expertise, ``causal discovery'' algorithms can be used to generate one from data. Unfortunately, causal discovery algorithms vary wildly in their results due to unrealistic data and modeling assumptions, so the results still need to be manually validated and adjusted. This paper presents a graph comparison tool designed to help analysts curate causal discovery results. This tool facilitates feedback loops whereby an analyst compares proposed graphs from multiple algorithms (or ensembles) and then uses insights from the comparison to refine parameters and inputs to the algorithms. We illustrate different types of comparisons and show how the interplay of causal discovery and graph comparison improves causal discovery.