Paper 2: Uncertainty-aware Topic Modeling Visualization
Valerie Mü̈ller, Christian Sieg, Lars Linsen
View presentation:2021-10-24T15:40:00ZGMT-0600Change your timezone on the schedule page
2021-10-24T15:40:00Z
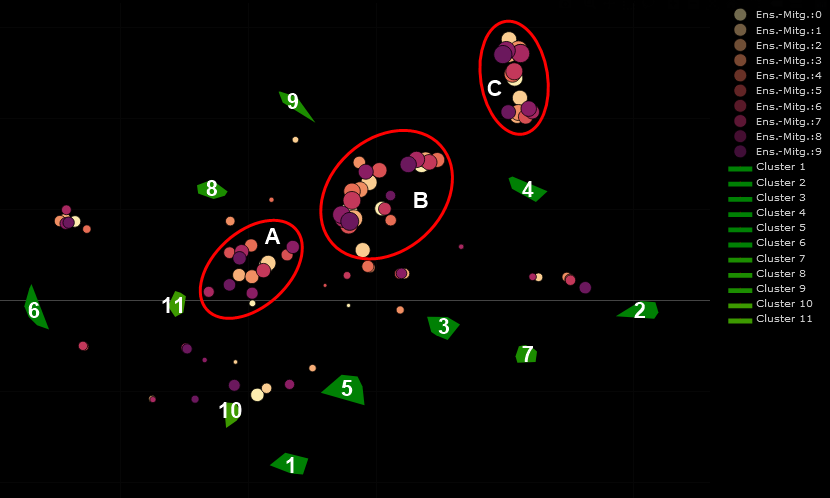
Abstract
Topic modeling is a state-of-the-art technique for analyzing text corpora. It uses a statistical model, most commonly Latent Dirichlet Allocation (LDA), to discover abstract topics that occur in the document collection. However, the LDA-based topic modeling procedure is based on a randomly selected initial configuration as well as a number of parameter values than need to be chosen. This induces uncertainties on the topic modeling results, and visualization methods should convey these uncertainties during the analysis process. We propose a visual uncertainty-aware topic modeling analysis. We capture the uncertainty by computing topic modeling ensembles and propose measures for estimating topic modeling uncertainty from the ensemble. Then, we propose to enhance state-of-the-art topic modeling visualization methods to convey the uncertainty in the topic modeling process. We visualize the entire ensemble of topic modeling results at different levels for topic and document analysis. We apply our visualization methods to a text corpus to document the impact of uncertainty on the analysis.