MultiVision: Designing Analytical Dashboards with Deep Learning Based Recommendation
Aoyu Wu, Yun Wang, Mengyu Zhou, Xinyi He, Haidong Zhang, Huamin Qu, Dongmei Zhang
External link (DOI)
View presentation:2021-10-28T16:00:00ZGMT-0600Change your timezone on the schedule page
2021-10-28T16:00:00Z
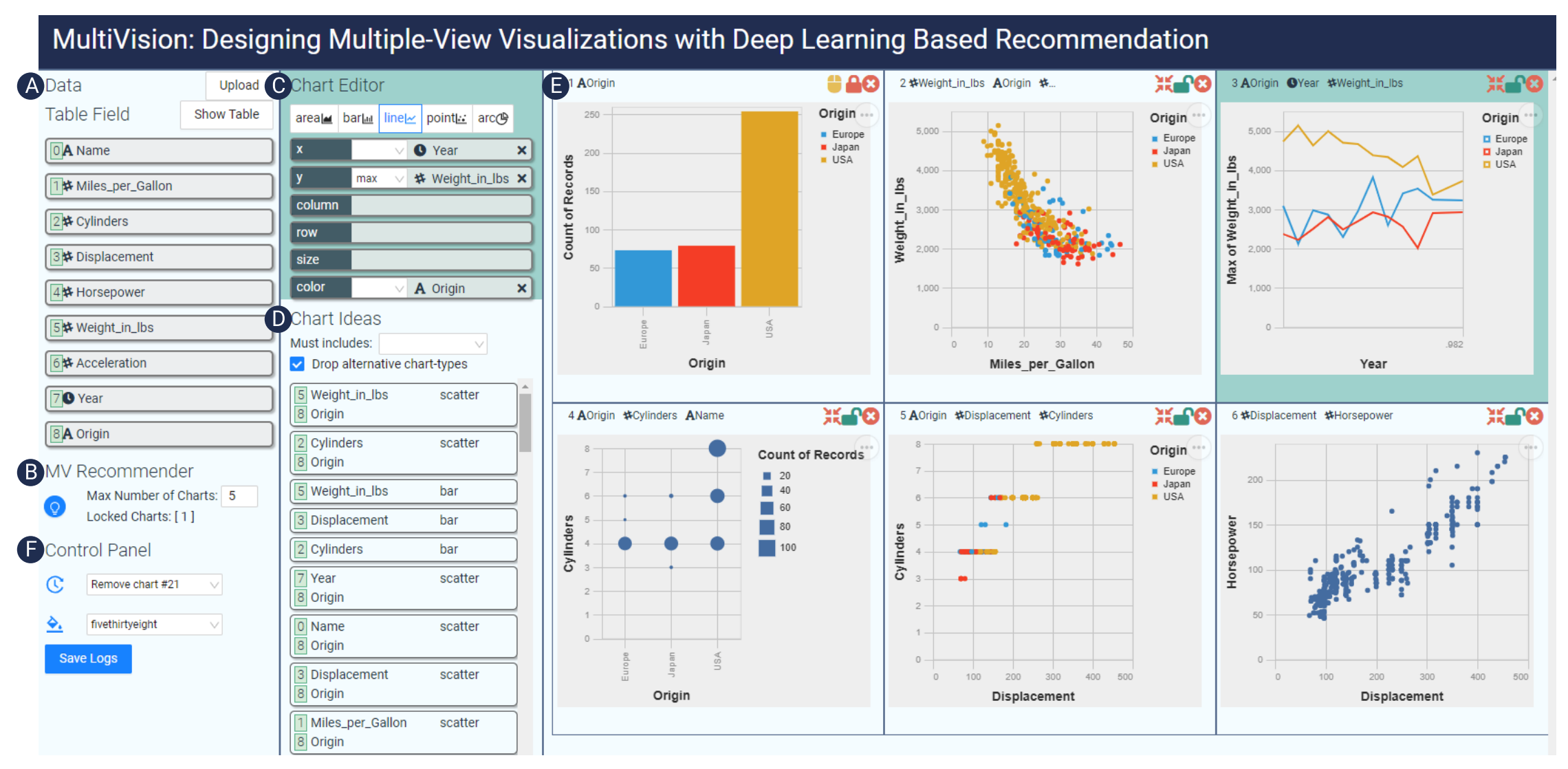
Abstract
We contribute a deep-learning-based method that assists in designing analytical dashboards for analyzing a data table. Given a data table, data workers usually need to experience a tedious and time-consuming process to select meaningful combinations of data columns for creating charts. This process is further complicated by the needs of creating dashboards composed of multiple views that unveil different perspectives of data. Existing automated approaches for recommending multiple-view visualizations mainly build on manually crafted design rules, producing sub-optimal or irrelevant suggestions. To address this gap, we present a deep learning approach for selecting data columns and recommending multiple charts. More importantly, we integrate the deep learning models into a mixed-initiative system. Our model could make recommendations given optional user-input selections of data columns. The model, in turn, learns from provenance data of authoring logs in an offline manner. We compare our deep learning model with existing methods for visualization recommendation and conduct a user study to evaluate the usefulness of the system.