STNet: An End-to-End Generative Framework for Synthesizing Spatiotemporal Super-Resolution Volumes
Jun Han, Hao Zheng, Danny Chen, Chaoli Wang
External link (DOI)
View presentation:2021-10-27T13:30:00ZGMT-0600Change your timezone on the schedule page
2021-10-27T13:30:00Z
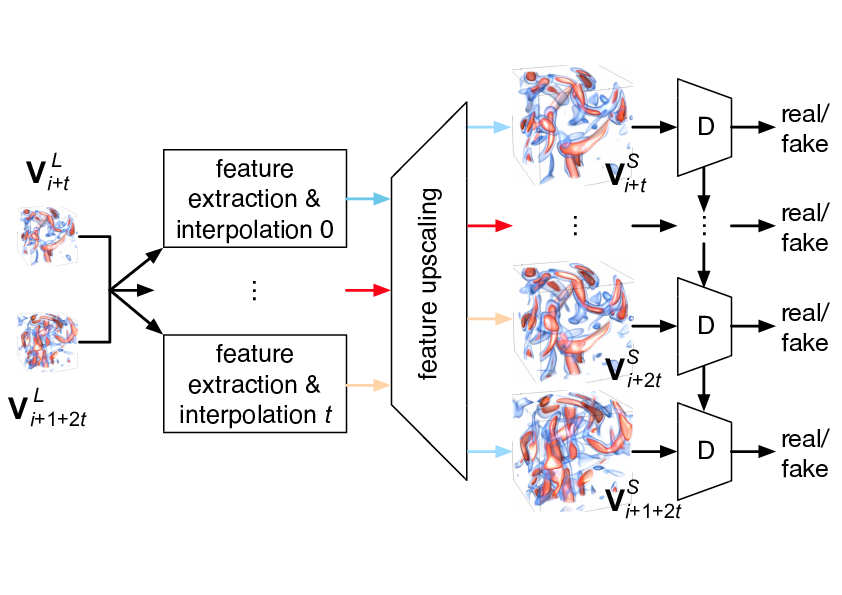
Fast forward
Direct link to video on YouTube: https://youtu.be/AezFUomjfzI
Abstract
We present STNet, an end-to-end generative framework that synthesizes spatiotemporal super-resolution volumes with high fidelity for time-varying data. STNet includes two modules: a generator and a spatiotemporal discriminator. The input to the generator is two low-resolution volumes at both ends, and the output is the intermediate and the two-ending spatiotemporal super- resolution volumes. The spatiotemporal discriminator, leveraging convolutional long short-term memory, accepts a spatiotemporal super-resolution sequence as input and predicts a conditional score for each volume based on its spatial (the volume itself) and temporal (the previous volumes) information. We propose an unsupervised pre-training stage using cycle loss to improve the generalization of STNet. Once trained, STNet can generate spatiotemporal super-resolution volumes from low-resolution ones, offering scientists an option to save data storage (i.e., sparsely sampling the simulation output in both spatial and temporal dimensions). We compare STNet with the baseline bicubic+linear interpolation, two deep learning solutions (SSR+TSR, STD), and a state-of-the-art tensor compression solution (TTHRESH) to show the effectiveness of STNet.