Sequen-C: A Multilevel Overview of Temporal Event Sequences
Jessica Magallanes, Tony Stone, Paul Morris, Suzanne Mason, Steven Wood, Maria-Cruz Villa-Uriol
External link (DOI)
View presentation:2021-10-28T17:30:00ZGMT-0600Change your timezone on the schedule page
2021-10-28T17:30:00Z
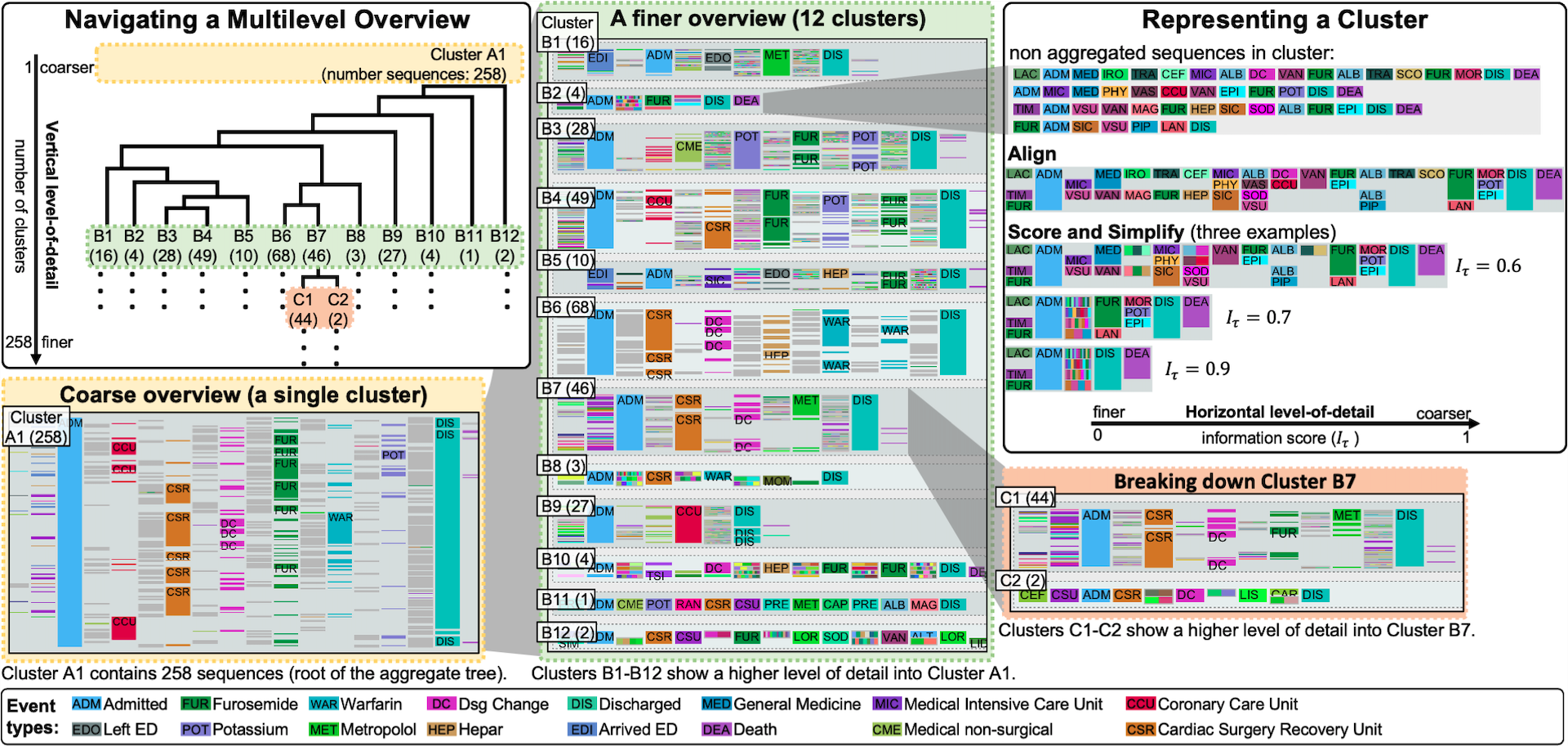
Fast forward
Direct link to video on YouTube: https://youtu.be/cbii6AHG9Oo
Abstract
Building a visual overview of temporal event sequences with an optimal level-of-detail (i.e. simplified but informative) is an ongoing challenge - expecting the user to zoom into every important aspect of the overview can lead to missing insights. We propose a technique to build and explore a multilevel overview of event sequences, from coarse to fine vertical or horizontal level-of-detail, using hierarchical aggregation and a novel cluster data representation Align-Score-Simplify. By default, the overview shows an optimal number of sequence clusters obtained through the average silhouette width metric – then users are able to explore alternative optimal sequence clusterings. The vertical level-of-detail of the overview changes along with the number of clusters, whilst the horizontal level-of-detail refers to the level of summarization applied to each cluster representation. The proposed technique has been implemented into a visualization system called Sequence Cluster Explorer (Sequen-C) that allows multilevel and detail-on-demand exploration through three coordinated views, and the inspection of data attributes at cluster, unique sequence, and individual sequence level. We present two case studies using real-world datasets in the healthcare domain: CUREd and MIMIC-III, which demonstrate how the technique can aid users in exploring and defining a set of distinct pathways that best summarize the dataset, while also being able of identifying deviating pathways and exploring data attributes for selected patterns.