Towards Visual Explainable Active Learning for Zero-Shot Classification
Shichao Jia, zeyu li, Nuo Chen, Jiawan Zhang
External link (DOI)
View presentation:2021-10-27T13:30:00ZGMT-0600Change your timezone on the schedule page
2021-10-27T13:30:00Z
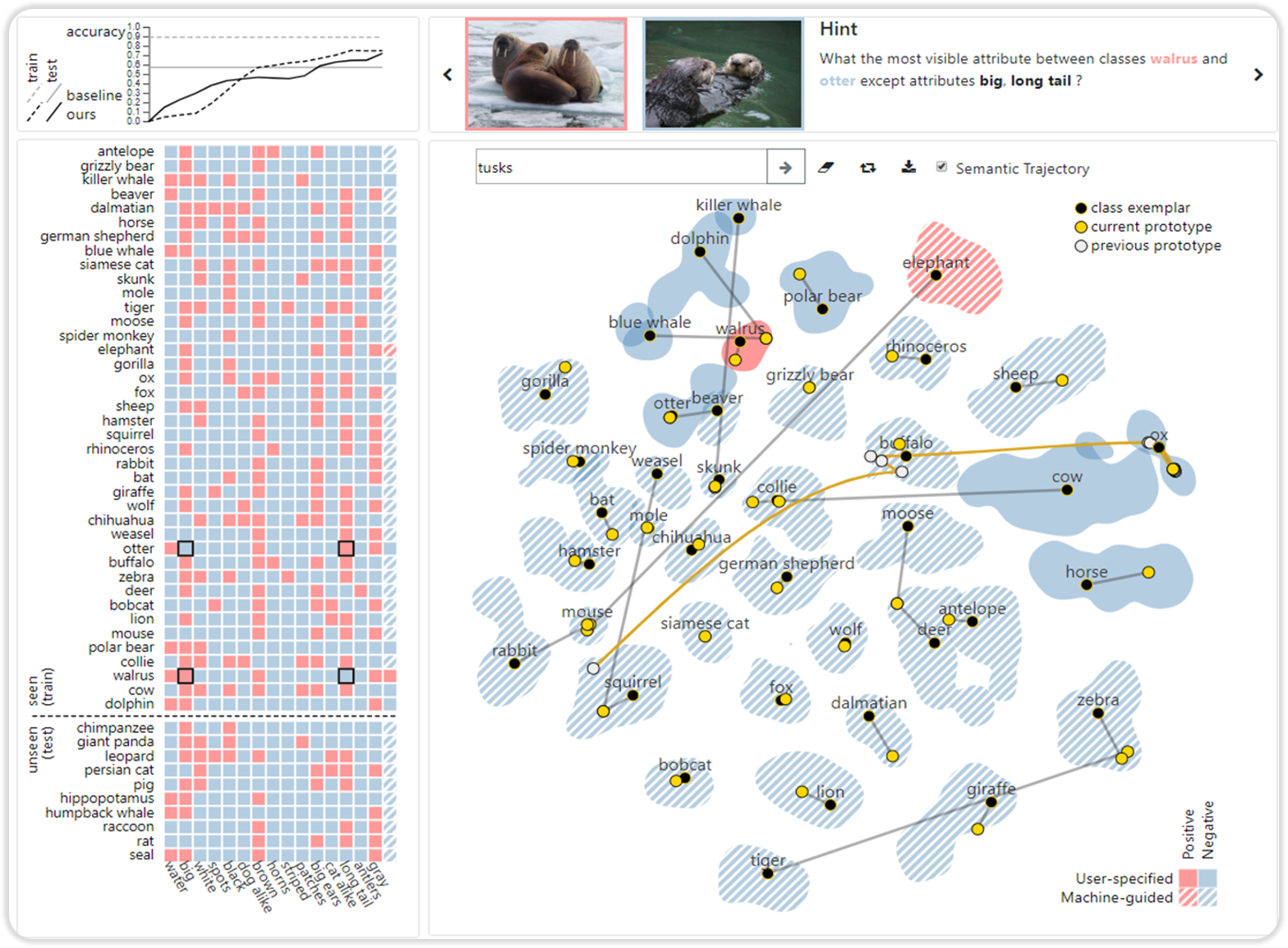
Fast forward
Direct link to video on YouTube: https://youtu.be/uDq00Plsct4
Abstract
Zero-shot classification is a promising paradigm to solve an applicable problem when the training classes and test classes are disjoint. Achieving this usually needs experts to externalize their domain knowledge by manually specifying a class-attribute matrix to define which classes have which attributes. Designing a suitable class-attribute matrix is the key to the subsequent procedure, but this design process is tedious and trial-and-error with no guidance. This paper proposes a visual explainable active learning approach with its design and implementation called semantic navigator to solve the above problems. This approach promotes human-AI teaming with four actions (ask, explain, recommend, respond) in each interaction loop. The machine asks contrastive questions to guide humans in the thinking process of attributes. A novel visualization called semantic map explains the current status of the machine. Therefore analysts can better understand why the machine misclassifies objects. Moreover, the machine recommends the labels of classes for each attribute to ease the labeling burden. Finally, humans can steer the model by modifying the labels interactively, and the machine adjusts its recommendations. The visual explainable active learning approach improves humans' efficiency of building zero-shot classification models interactively, compared with the method without guidance. We justify our results with user studies using the standard benchmarks for zero-shot classification.