COVID-view: Diagnosis of COVID-19 using Chest CT
Shreeraj Jadhav, Gaofeng Deng, Marlene Zawin, Arie Kaufman
External link (DOI)
View presentation:2021-10-29T15:00:00ZGMT-0600Change your timezone on the schedule page
2021-10-29T15:00:00Z
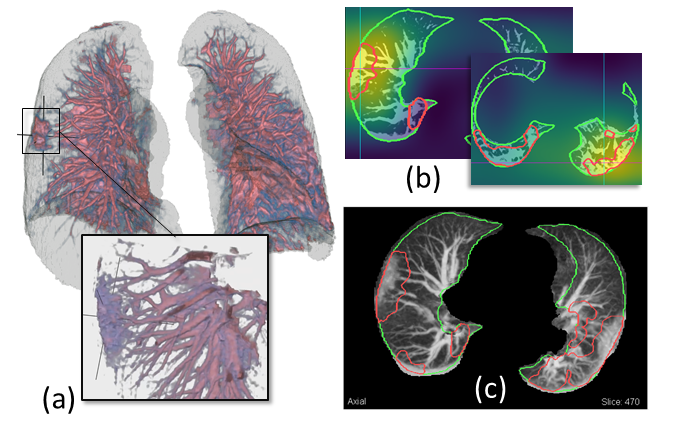
Fast forward
Direct link to video on YouTube: https://youtu.be/q9PAQZU8bb0
Abstract
Significant work has been done towards deep learning (DL) models for automatic lung and lesion segmentation and classification of COVID-19 on chest CT data. However, comprehensive visualization systems focused on supporting the dual visual+DL diagnosis of COVID-19 are non-existent. We present COVID-view, a visualization application specially tailored for radiologists to diagnose COVID-19 from chest CT data. The system incorporates a complete pipeline of automatic lungs segmentation, localization/isolation of lung abnormalities, followed by visualization, visual and DL analysis, and measurement/quantification tools. Our system combines the traditional 2D workflow of radiologists with newer 2D and 3D visualization techniques with DL support for a more comprehensive diagnosis. COVID-view incorporates a novel DL model for classifying the patients into positive/negative COVID-19 cases, which acts as a reading aid for the radiologist using COVID-view, and provides the attention heatmap as an explainable DL for the model output. We designed and evaluated COVID-view through suggestions, close feedback and conducting case studies of real-world patient data by expert radiologists who have substantial experience diagnosing chest CT scans for COVID-19, pulmonary embolism, and other forms of lung infections. We present requirements and task analysis for the diagnosis of COVID-19 that motivate our design choices and results in a practical system which is capable of handling real-world patient cases.