Honorable Mention
VBridge: Connecting the Dots Between Features and Data to Explain Healthcare Models
Furui Cheng, Dongyu Liu, Fan Du, Yanna Lin, Alexandra Zytek, Haomin Li, Huamin Qu, Kalyan Veeramachaneni
External link (DOI)
View presentation:2021-10-29T16:15:00ZGMT-0600Change your timezone on the schedule page
2021-10-29T16:15:00Z
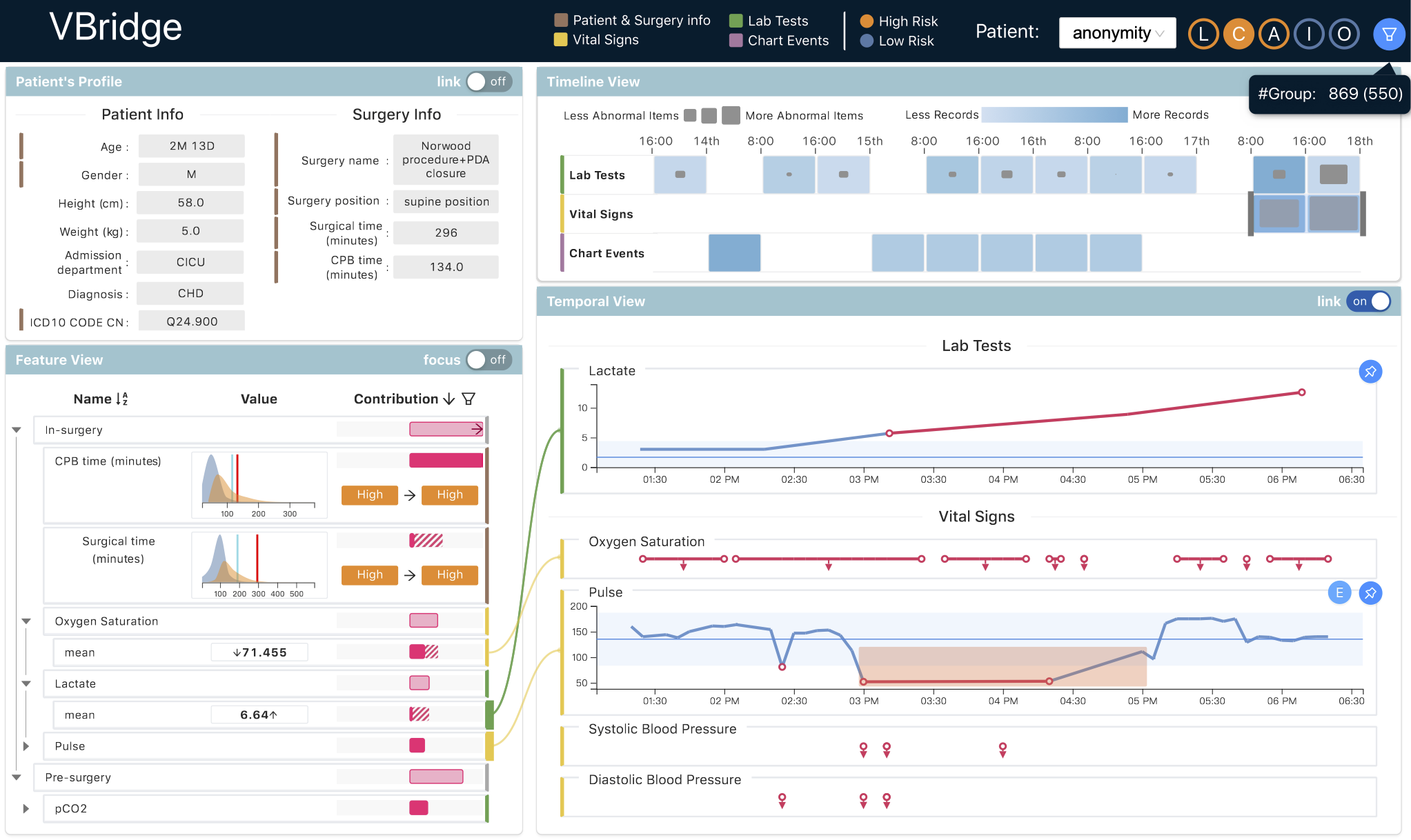
Fast forward
Direct link to video on YouTube: https://youtu.be/PnAxWRLKgFY
Abstract
Machine learning (ML) is increasingly applied to Electronic Health Records (EHRs) to solve clinical prediction tasks. Although many ML models perform promisingly, issues with model transparency and interpretability limit their adoption in clinical practice. Directly using existing explainable ML techniques in clinical settings can be challenging. Through literature surveys and collaborations with six clinicians with an average of 17 years of clinical experience, we identified three key challenges, including clinicians' unfamiliarity with ML features, lack of contextual information, and the need for cohort-level evidence. Following an iterative design process, we further designed and developed VBridge, a visual analytics tool that seamlessly incorporates ML explanations into clinicians' decision-making workflow. The system includes a novel hierarchical display of contribution-based feature explanations and enriched interactions that connect the dots between ML features, explanations, and data. We demonstrated the effectiveness of VBridge through two case studies and expert interviews with four clinicians, showing that visually associating model explanations with patients' situational records can help clinicians better interpret and use model predictions when making clinician decisions. We further derived a list of design implications for developing future explainable ML tools to support clinical decision-making.