Best Paper Award
Feature Curves and Surfaces of 3D Asymmetric Tensor Fields
Shih-Hsuan Hung, Yue Zhang, Harry Yeh, Eugene Zhang
External link (DOI)
View presentation:2021-10-26T15:45:00ZGMT-0600Change your timezone on the schedule page
2021-10-26T15:45:00Z
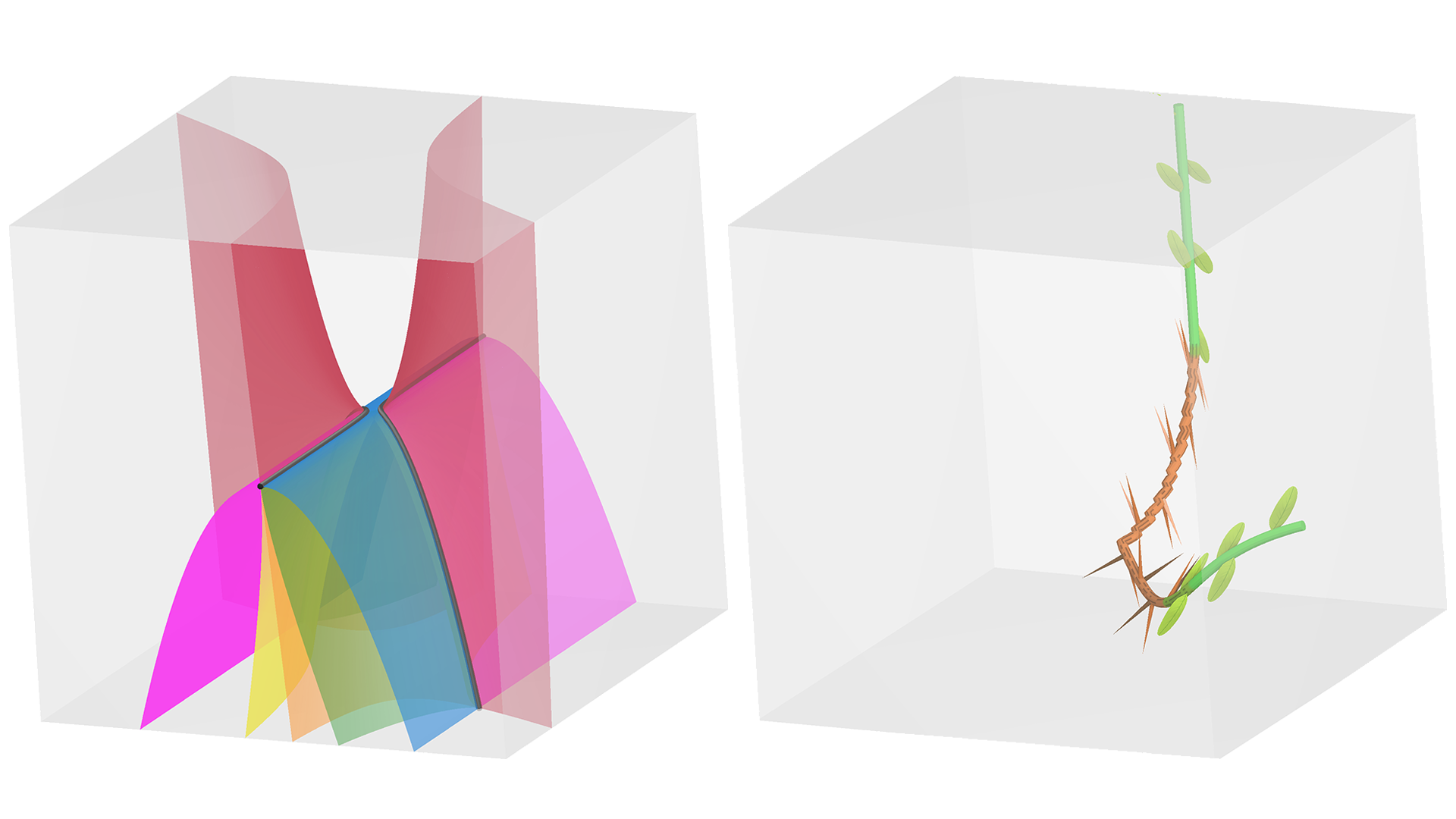
Fast forward
Direct link to video on YouTube: https://youtu.be/kIYX3lWuIew
Abstract
3D asymmetric tensor fields have found many applications in science and engineering domains, such as fluid dynamics and solid mechanics. 3D asymmetric tensors can have complex eigenvalues, which makes their analysis and visualization more challenging than 3D symmetric tensors. Existing research in tensor field visualization focuses on 2D asymmetric tensor fields and 3D symmetric tensor fields. In this paper, we address the analysis and visualization of 3D asymmetric tensor fields. We introduce six topological surfaces and one topological curve, which lead to an eigenvalue space based on the tensor mode that we define. In addition, we identify several non-topological feature surfaces that are nonetheless physically important. Included in our analysis are the realizations that triple degenerate tensors are structurally stable and form curves, unlike the case for 3D symmetric tensors fields. Furthermore, there are two different ways of measuring the relative strengths of rotation and angular deformation in the tensor fields, unlike the case for 2D asymmetric tensor fields. We extract these feature surfaces using the A-patches algorithm. However, since three of our feature surfaces are quadratic, we develop a method to extract quadratic surfaces at any given accuracy. To facilitate the analysis of eigenvector fields, we visualize a hyperstreamline as a tree stem with the other two eigenvectors represented as thorns in the real domain or the dual-eigenvectors as leaves in the complex domain. To demonstrate the effectiveness of our analysis and visualization, we apply our approach to datasets from solid mechanics and fluid dynamics.