Attribute-based Explanation of Non-Linear Embeddings of High-Dimensional Data
Jan-Tobias Sohns, Michaela Schmitt, Fabian Jirasek, Hans Hasse, Heike Leitte
External link (DOI)
View presentation:2021-10-27T18:00:00ZGMT-0600Change your timezone on the schedule page
2021-10-27T18:00:00Z
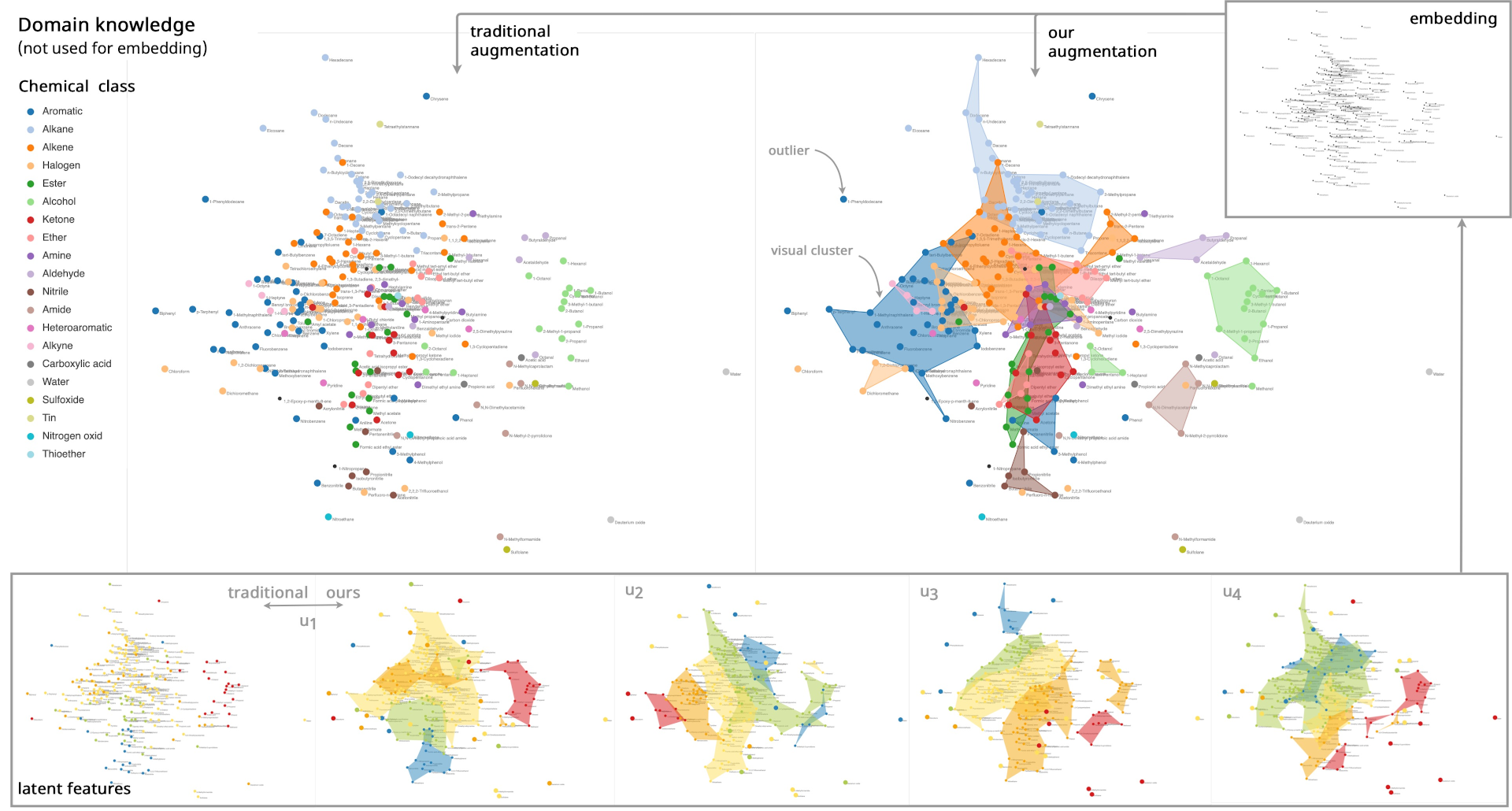
Abstract
Embeddings of high-dimensional data are widely used to explore data, to verify analysis results, and to communicate information. Their explanation, in particular with respect to the input attributes, is often difficult. With linear projects like PCA the axes can still be annotated meaningfully. With non-linear projections this is no longer possible and alternative strategies such as attribute-based color coding are required. In this paper, we review existing augmentation techniques and discuss their limitations. We present the Non-Linear Embeddings Surveyor (NoLiES) that combines a novel augmentation strategy for projected data (rangesets) with interactive analysis in a small multiples setting. Rangesets use a set-based visualization approach for binned attribute values that enable the user to quickly observe structure and detect outliers. We detail the link between algebraic topology and rangesets and demonstrate the utility of NoLiES in case studies with various challenges (complex attribute value distribution, many attributes, many data points) and a real-world application to understand latent features of matrix completion in thermodynamics.