Sibyl: Understanding and Addressing the Usability Challenges of Machine Learning In High-Stakes Decision Making
Alexandra Zytek, Dongyu Liu, Rhema Vaithianathan, Kalyan Veeramachaneni
External link (DOI)
View presentation:2021-10-27T16:15:00ZGMT-0600Change your timezone on the schedule page
2021-10-27T16:15:00Z
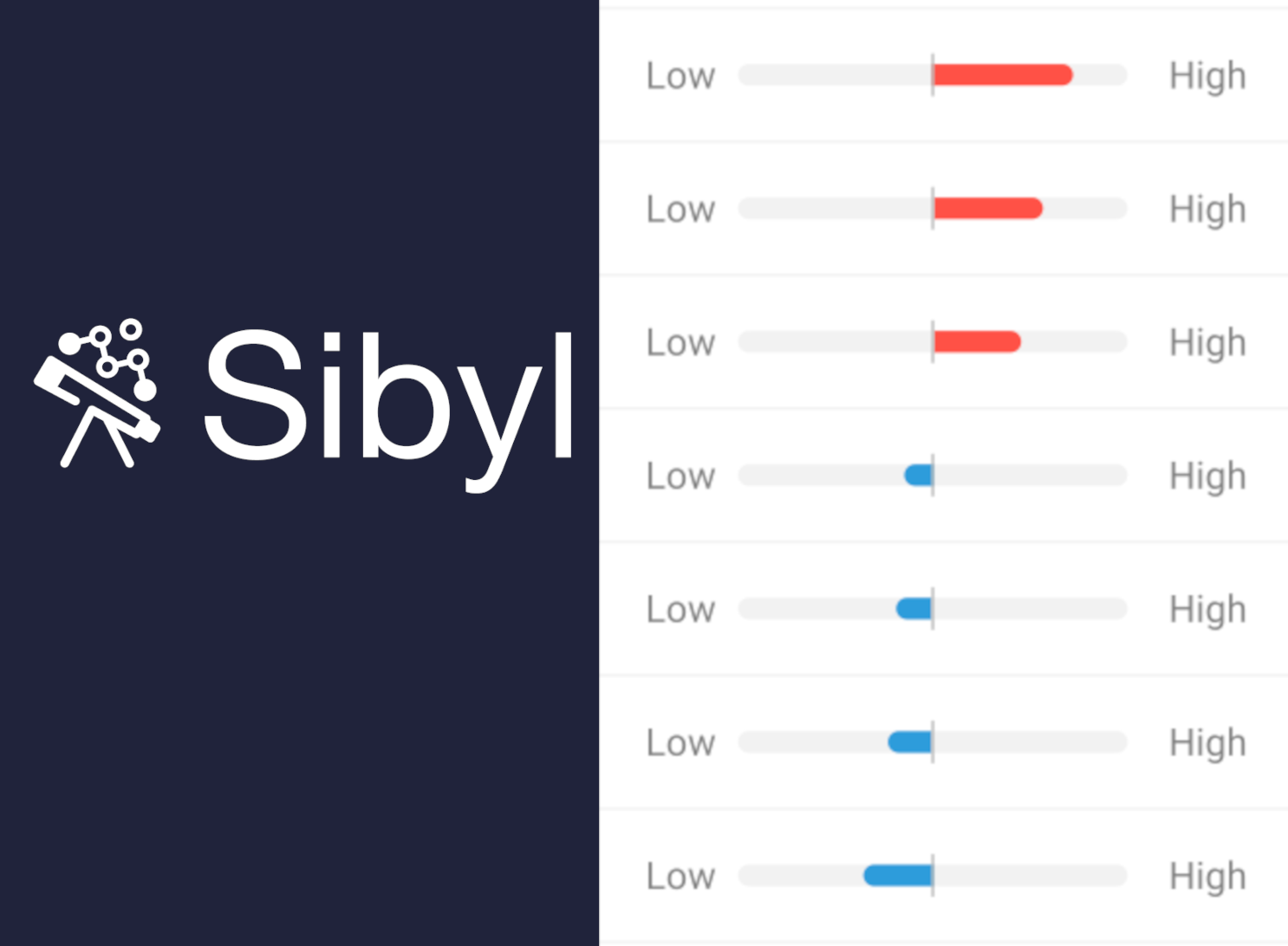
Fast forward
Direct link to video on YouTube: https://youtu.be/ClATgmKwVCs
Abstract
Machine learning (ML) is being applied to a diverse and ever-growing set of domains. In many cases, domain experts --- who often have no expertise in ML or data science --- are asked to use ML predictions to make high-stakes decisions. Multiple ML usability challenges can appear as result, such as lack of user trust in the model, inability to reconcile human-ML disagreement, and ethical concerns about oversimplification of complex problems to a single algorithm output. In this paper, we investigate the ML usability challenges that present in the domain of child welfare screening through a series of collaborations with child welfare screeners. Following the iterative design process between the ML scientists, visualization researchers, and domain experts (child screeners), we first identified four key ML challenges and honed in on one promising explainable ML technique to address them (local factor contributions). Then we designed, implemented, and evaluated our visual analytics tool, Sibyl, to increase the interpretability and interactivity of local factor contributions. The effectiveness of our tool is demonstrated by two formal user studies with 12 non-expert participants and 13 expert participants respectively. Valuable feedback is collected, from which we also composed a list of design implications as a useful guideline for researchers that aim to develop an interpretable and interactive visualization tool for ML prediction models deployed for child welfare screeners and other similar domain experts.