THALIS: Human-Machine Analysis of Longitudinal Symptoms in Cancer Therapy
Carla Floricel, Md Nafiul Nipu, Mikayla Biggs, Andrew Wentzel, Guadalupe Canahuate, Lisanne van Dijk, Abdallah Mohamed, Clifton David Fuller, G. Elisabeta Marai
External link (DOI)
View presentation:2021-10-28T15:45:00ZGMT-0600Change your timezone on the schedule page
2021-10-28T15:45:00Z
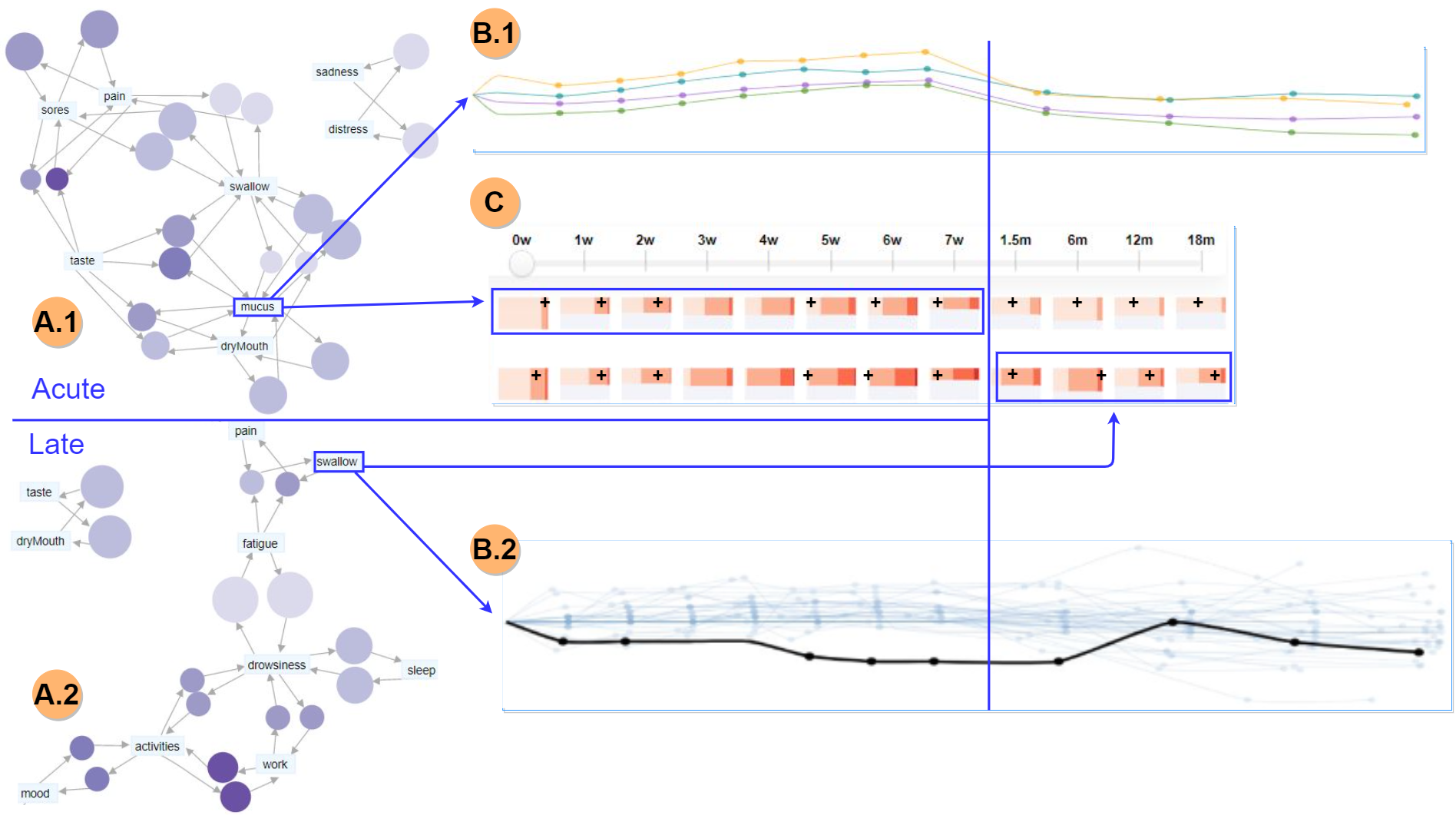
Fast forward
Direct link to video on YouTube: https://youtu.be/HXuYC6NimSg
Abstract
Although cancer patients survive years after oncologic therapy, they are plagued with long-lasting or permanent residual symptoms, whose severity, rate of development, and resolution after treatment vary largely between survivors. The analysis and interpretation of symptoms is complicated by their partial co-occurrence, variability across populations and across time, and, in the case of cancers that use radiation, by further symptom dependency on the prescribed therapy. We describe an integrated environment for visual analysis and knowledge discovery from cancer therapy symptom data, developed in close collaboration with oncology experts. Our visual environment leverages unsupervised machine learning methodology over cohorts of patients, and, in conjunction with custom visual encodings and interactions, provides context for new patients based on patients with similar diagnostic features and symptom evolution. We evaluate this environment on data collected from a cohort of head and neck cancer patients. Feedback from our clinician collaborators indicates that this environment supports knowledge discovery beyond the limits of machines or humans alone, and that it serves as a valuable tool in both the clinic and symptom research.