Revisiting Dimensionality Reduction Techniques for Visual Cluster Analysis: An Empirical Study
Jiazhi Xia, Yuchen Zhang, Jie Song, Yang Chen, Yunhai Wang, Shixia Liu
External link (DOI)
View presentation:2021-10-27T17:30:00ZGMT-0600Change your timezone on the schedule page
2021-10-27T17:30:00Z
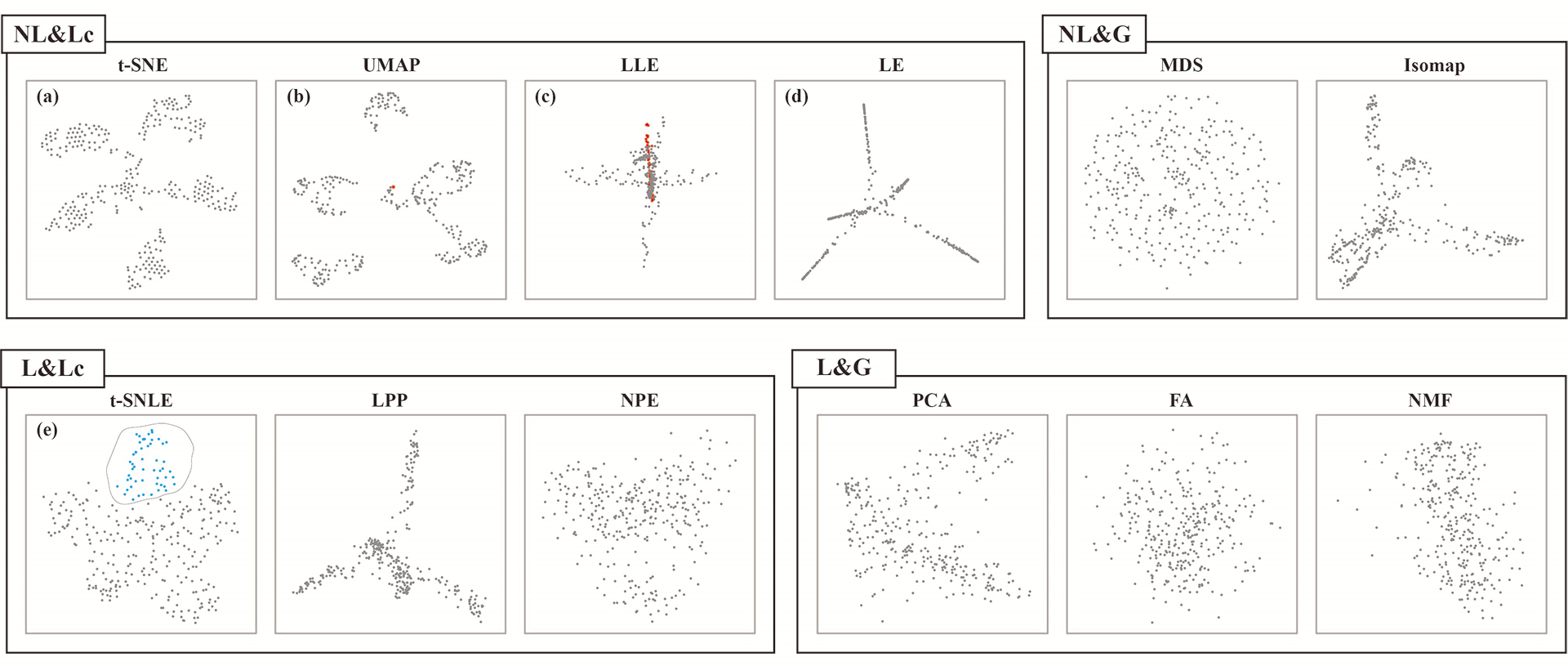
Fast forward
Direct link to video on YouTube: https://youtu.be/aNjcy5KLfd8
Abstract
Dimensionality Reduction (DR) techniques can generate 2D projections and enable visual exploration of cluster structures of high-dimensional datasets. However, different DR techniques would yield various patterns, which significantly affect the performance of visual cluster analysis tasks. We present the results of a user study that investigates the influence of different DR techniques on visual cluster analysis. Our study focuses on the most concerned property types, namely the linearity and locality, and evaluates twelve representative DR techniques that cover the concerned properties. Four controlled experiments were conducted to evaluate how the DR techniques facilitate the tasks of 1) identifying clusters, 2) associating cluster members, 3) comparing distances among clusters, and 4) comparing cluster densities, respectively. We also evaluated users’ subjective preference of the DR techniques regarding the quality of projected clusters. The results show that: 1) Non-linear and Local techniques are preferred in identifying clusters and associating cluster members; 2) Linear techniques perform better than non-linear techniques in comparing cluster densities; 3) UMAP (Uniform Manifold Approximation and Projection) and t-SNE (t-Distributed Stochastic Neighbor Embedding) perform the best in identifying clusters and associating cluster members; 4) NMF(Nonnegative Matrix Factorization) has competitive performance in comparing distances among clusters; 5) t-SNLE(t-Distributed Stochastic Neighbor Linear Embedding) has competitive performance in comparing cluster densities.