CloudFindr: A Deep Learning Cloud Artifact Masker for Satellite DEM Data
Kalina Borkiewicz, Viraj Shah, J.P. Naiman, Chuanyue Shen, Stuart Levy, Jeffrey D Carpenter
External link (DOI)
View presentation:2021-10-27T15:00:00ZGMT-0600Change your timezone on the schedule page
2021-10-27T15:00:00Z
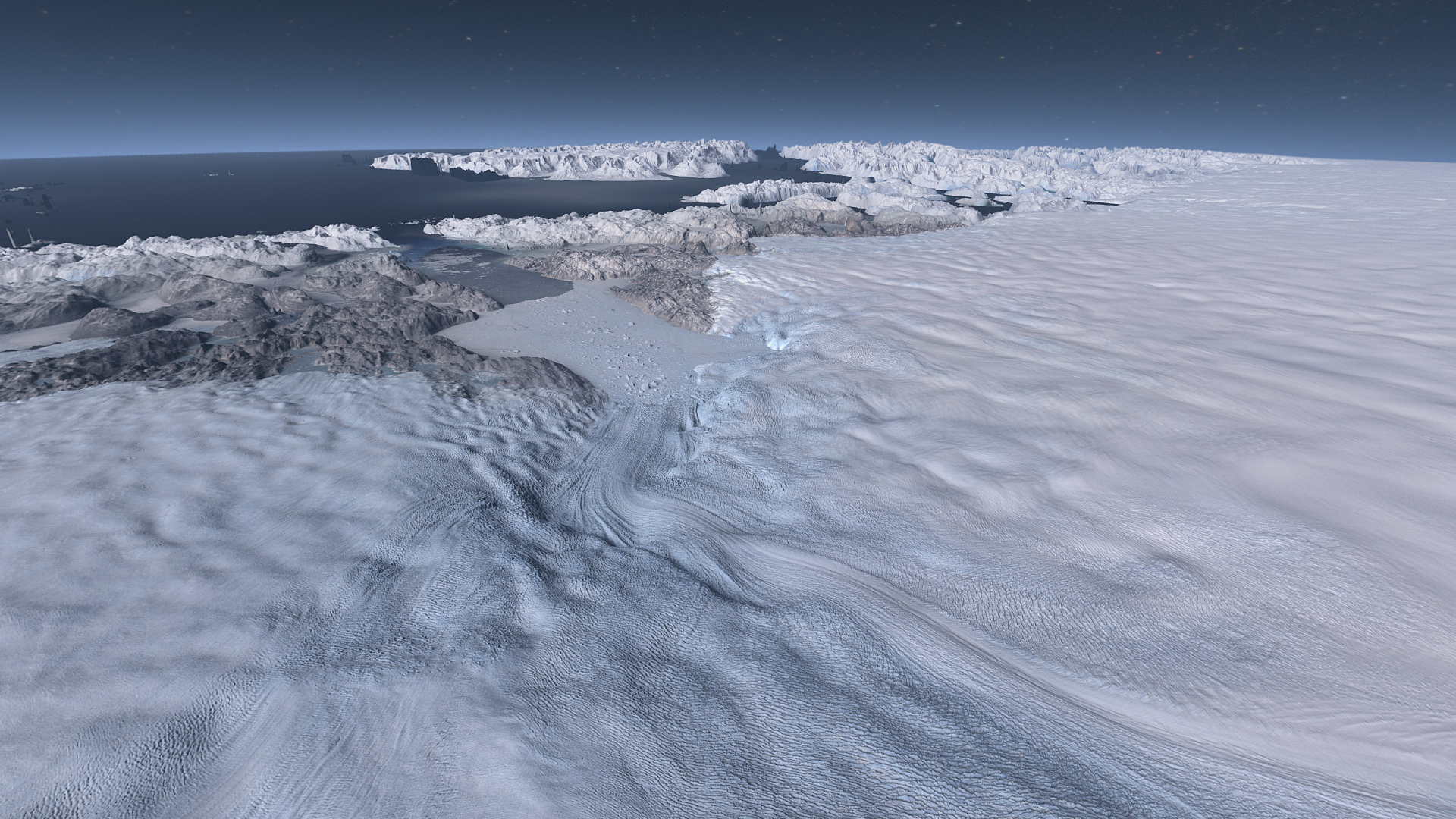
Fast forward
Direct link to video on YouTube: https://youtu.be/-Xox4RsgoVw
Keywords
Data Management, Processing, Wrangling, Feature Detection, Extraction, Tracking & Transformation, Machine Learning Techniques, Cartography, Maps, Image and Signal Processing, General Public, Application Motivated Visualization, Geospatial Data, Image and Video Data
Abstract
Artifact removal is an integral component of cinematic scientific visualization, and is especially challenging with big datasets in which artifacts are difficult to define. In this paper, we describe a method for creating cloud artifact masks which can be used to remove artifacts from satellite imagery using a combination of traditional image processing together with deep learning based on U-Net. Compared to previous methods, our approach does not require multi-channel spectral imagery but performs successfully on single-channel Digital Elevation Models (DEMs). DEMs are a representation of the topography of the Earth and have a variety applications including planetary science, geology, flood modeling, and city planning.