Why did my AI Agent Lose? Visual Analytics for Scaling Up After-Action Review
Delyar Tabatabai, Anita Ruangrotsakun, Jed Irvine, Jonathan Dodge, Zeyad Shureih, Kin-Ho Lam, Margaret Burnett, Alan Paul Fern, Minsuk Kahng
External link (DOI)
View presentation:2021-10-27T15:30:00ZGMT-0600Change your timezone on the schedule page
2021-10-27T15:30:00Z
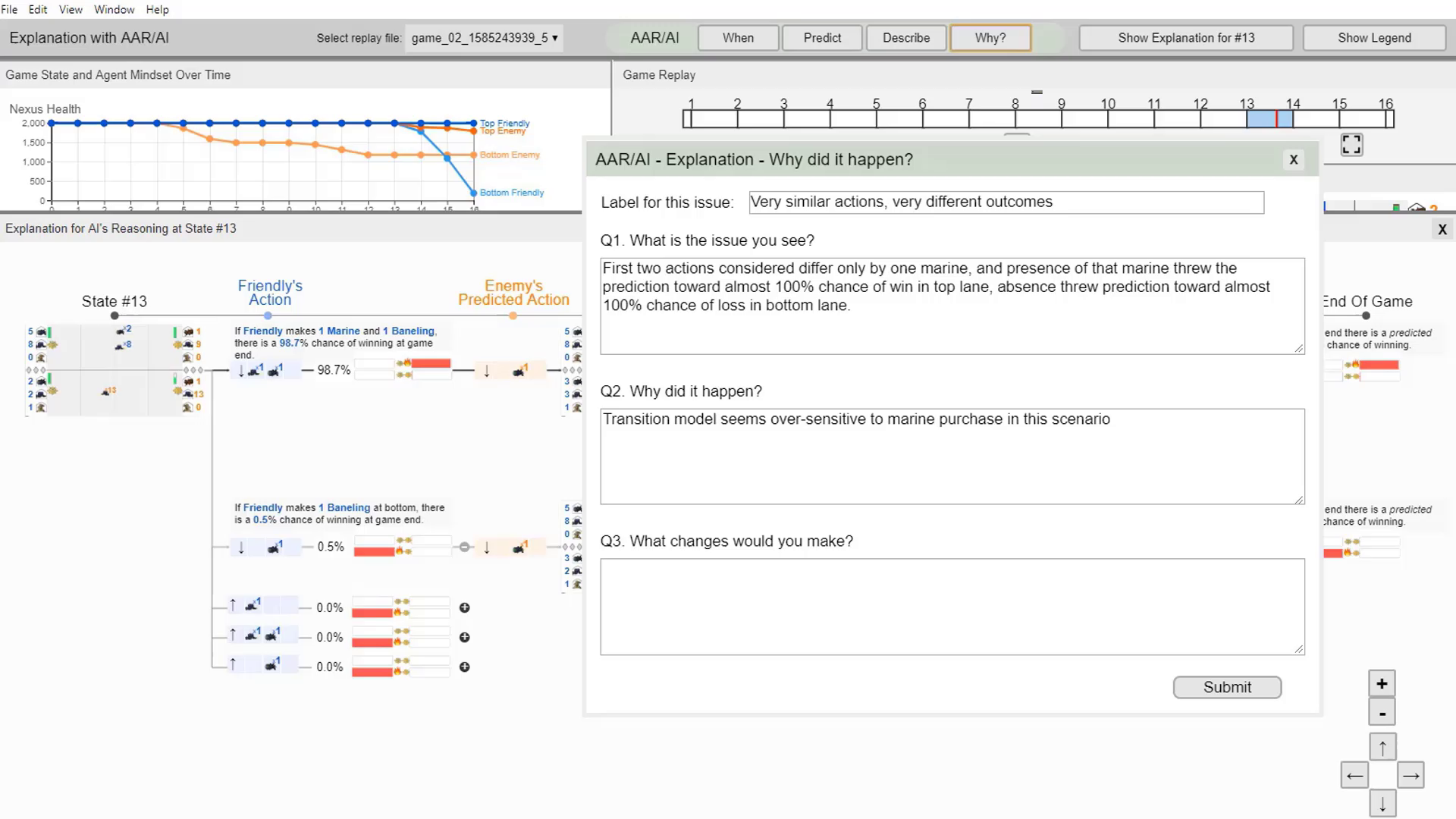
Fast forward
Direct link to video on YouTube: https://youtu.be/KcD7MuFJT9g
Keywords
Machine Learning, Statistics, Modelling, and Simulation Applications, Data Analysis, Reasoning, Problem Solving, and Decision Making, Process/Workflow Design
Abstract
How can we help domain-knowledgeable users who do not have expertise in AI analyze why an AI agent failed? Our research team previously developed a new structured process for such users to assess AI, called After-Action Review for AI (AAR/AI), consisting of a series of steps a human takes to assess an AI agent and formalize their understanding. In this paper, we investigate how the AAR/AI process can scale up to support reinforcement learning (RL) agents that operate in complex environments. We augment the AAR/AI process to be performed at three levels--episode-level, decision-level, and explanation-level--and integrate it into our redesigned visual analytics interface. We illustrate our approach through a usage scenario of analyzing why a RL agent lost in a complex real-time strategy game built with the StarCraft 2 engine. We believe integrating structured processes like AAR/AI into visualization tools can help visualization play a more critical role in AI interpretability.