Uncertainty Visualization of the Marching Squares and Marching Cubes Topology Cases
Tushar M. Athawale, Sudhanshu Sane, Chris R. Johnson
External link (DOI)
View presentation:2021-10-29T13:40:00ZGMT-0600Change your timezone on the schedule page
2021-10-29T13:40:00Z
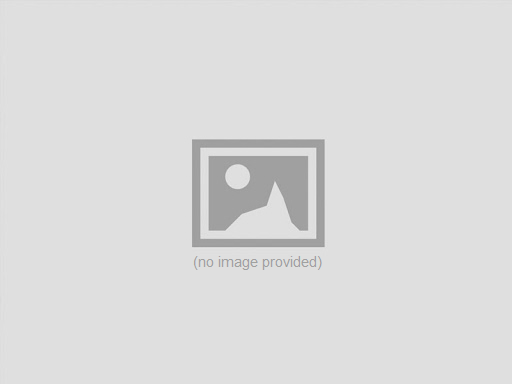
Fast forward
Direct link to video on YouTube: https://youtu.be/Z6jXaJ4uG44
Keywords
Uncertainty Visualization, Scalar Field Data, Computational Topology-based Techniques, Isosurface Techniques
Abstract
Marching squares (MS) and marching cubes (MC) are widely used algorithms for level-set visualization of scientific data. In this paper, we address the challenge of uncertainty visualization of the topology cases of the MS and MC algorithms for uncertain scalar field data sampled on a uniform grid. The visualization of the MS and MC topology cases for uncertain data is challenging due to their exponential nature and a possibility of multiple topology cases per cell of a grid. We propose the topology case count and entropy-based techniques for quantifying uncertainty in the topology cases of the MS and MC algorithms when noise in data is modeled with probability distributions. We demonstrate the applicability of our techniques for independent and correlated uncertainty assumptions. We visualize the quantified topological uncertainty via color mapping proportional to uncertainty, as well as with interactive probability queries in the MS case and entropy isosurfaces in the MC case. We demonstrate the utility of our uncertainty quantification framework in identifying the isovalues exhibiting relatively high topological uncertainty. We illustrate the effectiveness of our techniques via results on synthetic, simulation, and hixel datasets.