SSR-TVD: Spatial Super-Resolution for Time-Varying Data Analysis and Visualization
Jun Han, Chaoli Wang
External link (DOI)
View presentation:2021-10-28T18:15:00ZGMT-0600Change your timezone on the schedule page
2021-10-28T18:15:00Z
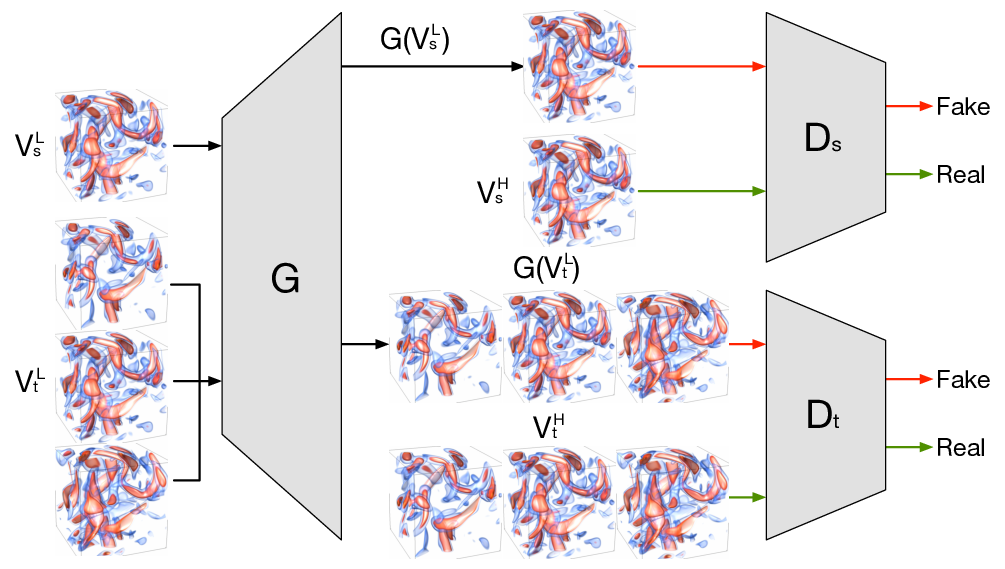
Fast forward
Direct link to video on YouTube: https://youtu.be/LajFBGu5Ch4
Keywords
Time-varying data visualization, deep learning, super-resolution, generative adversarial network
Abstract
We present SSR-TVD, a novel deep learning framework that produces coherent spatial super-resolution (SSR) of time-varying data (TVD) using adversarial learning. In scientific visualization, SSR-TVD is the first work that applies the generative adversarial network (GAN) to generate high-resolution volumes for three-dimensional time-varying data sets. The design of SSR-TVD includes a generator and two discriminators (spatial and temporal discriminators). The generator takes a low-resolution volume as input and outputs a synthesized high-resolution volume. To capture spatial and temporal coherence in the volume sequence, the two discriminators take the synthesized high-resolution volume(s) as input and produce a score indicating the realness of the volume(s). Our method can work in the in situ visualization setting by downscaling volumetric data from selected time steps as the simulation runs and upscaling downsampled volumes to their original resolution during postprocessing. To demonstrate the effectiveness of SSR-TVD, we show quantitative and qualitative results with several time-varying data sets of different characteristics and compare our method against volume upscaling using bicubic interpolation and a solution solely based on CNN.