Imma Sort by Two or More Attributes With Interpretable Monotonic Multi-Attribute Sorting
Yan Lyu, Fan Gao, I-Shuen Wu, Brian Lim
External link (DOI)
View presentation:2021-10-27T17:00:00ZGMT-0600Change your timezone on the schedule page
2021-10-27T17:00:00Z
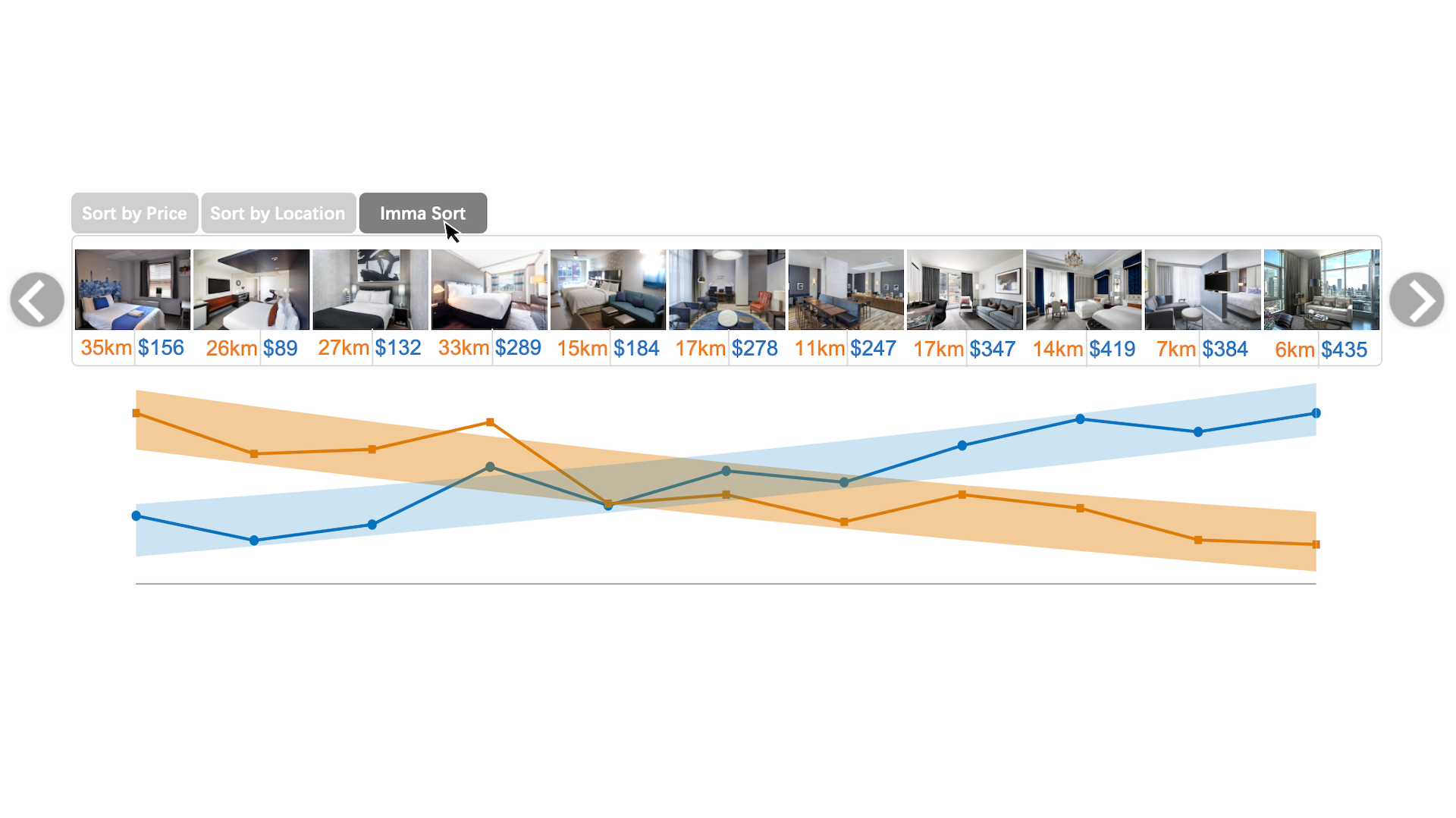
Fast forward
Direct link to video on YouTube: https://youtu.be/m3ojNqQbScI
Keywords
Multi-attribute sorting, decision making, interpretability, human predictability, predictive interpretability.
Abstract
Many choice problems often involve multiple attributes which are mentally challenging, because only one attribute is neatly sorted while others could be randomly arranged. We hypothesize that perceiving approximately monotonic trends across multiple attributes is key to the overall interpretability of sorted results, because users can easily predict the attribute values of the next items. We extend a ranking principal curve model to tune monotonic trends in attributes and present Imma Sort to sort items by multiple attributes simultaneously by trading-off the monotonicity in the primary sorted attribute to increase the human predictability for other attributes. We characterize how it performs for varying attribute correlations, attribute preferences, list lengths and number of attributes. We further extend Imma Sort with ImmaAnchor and ImmaCenter to improve the learnability and efficiency to search sorted items with conflicting attributes. We demonstrate usage scenarios for two applications and evaluate its learnability, usability, interpretability and user performance in prediction and search tasks. We find that Imma Sort improves the interpretability and satisfaction of sorting by ≥ 2 attributes. We discuss why, when, where, and how to deploy Imma Sort for real-world applications.