Deconstructing Categorization in Visualization Recommendation: A Taxonomy and Comparative Study
Doris Lee, Vidya Setlur, Melanie Tory, Karrie Karahalios, Aditya Parameswaran
External link (DOI)
View presentation:2021-10-27T17:45:00ZGMT-0600Change your timezone on the schedule page
2021-10-27T17:45:00Z
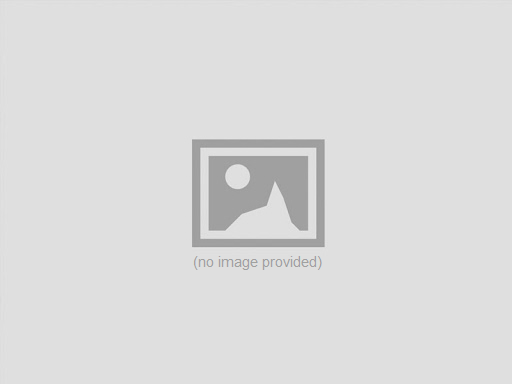
Fast forward
Direct link to video on YouTube: https://youtu.be/21ArbHmwTiI
Keywords
visual analysis, analytical workflow, discovery-driven analysis, visualization recommendations
Abstract
Visualization recommendation (VisRec) systems provide users with suggestions for potentially interesting and useful next steps during exploratory data analysis. These recommendations are typically organized into categories based on their analytical actions, i.e., operations employed to transition from the current exploration state to a recommended visualization. However, despite the emergence of a plethora of VisRec systems in recent work, the utility of the categories employed by these systems in analytical workflows has not been systematically investigated. Our paper explores the efficacy of recommendation categories by formalizing a taxonomy of common categories and developing a system, Frontier, that implements these categories. Using Frontier, we evaluate workflow strategies adopted by users and how categories influence those strategies. Participants found recommendations that add attributes to enhance the current visualization and recommendations that filter to sub-populations to be comparatively most useful during data exploration. Our findings pave the way for next-generation VisRec systems that are adaptive and personalized via carefully chosen, effective recommendation categories.