Should I Follow this Model? The Effect of Uncertainty Visualization on the Acceptance of Time Series Forecasts
Dirk Leffrang, Oliver Mueller
External link (DOI)
View presentation:2021-10-24T16:00:00ZGMT-0600Change your timezone on the schedule page
2021-10-24T16:00:00Z
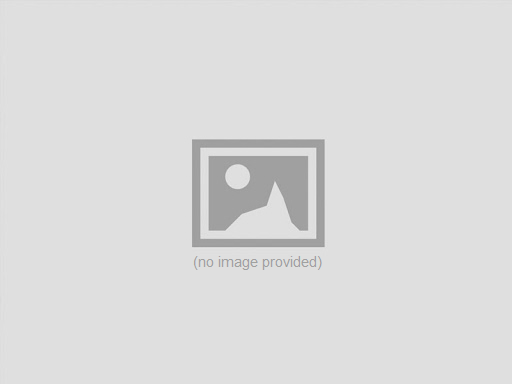
Fast forward
Direct link to video on YouTube: https://youtu.be/BaKlTFM8ZSk
Abstract
Time series forecasts are ubiquitous, ranging from daily weather forecasts to projections of pandemics such as COVID-19. Communicating the uncertainty associated with such forecasts is important, because it may affect users' trust in a forecasting model and, in turn, the decisions made based on the model. Although there exists a growing body of research on visualizing uncertainty in general, the important case of visualizing prediction uncertainty in time series forecasting has not been studied yet. Against this background, we investigated how different visualizations of predictive uncertainty affect the extent to which people follow predictions of a time series forecasting model. More specifically, we conducted an online experiment on forecasting occupied hospital beds due to the COVID-19 pandemic, measuring the influence of uncertainty visualization of algorithmic predictions on participants' own predictions. In contrast to prior studies, our empirical results suggest that more salient visualizations of uncertainty lead to decreased willingness to follow algorithmic forecasts.