Beyond Visual Analytics: Human-Machine Teaming for AI-Driven Data Sensemaking
John Wenskovitch, Corey Fallon, Kate Miller, Aritra Dasgupta
External link (DOI)
View presentation:2021-10-24T13:45:00ZGMT-0600Change your timezone on the schedule page
2021-10-24T13:45:00Z
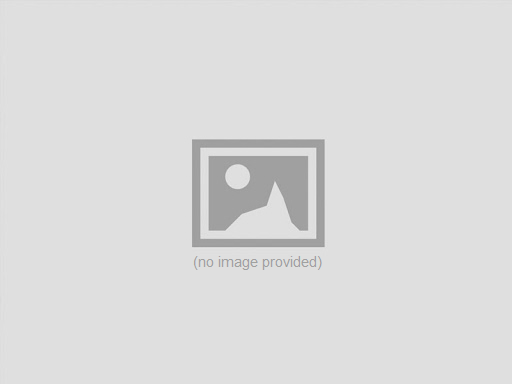
Fast forward
Direct link to video on YouTube: https://youtu.be/sPesrLfBz0A
Abstract
“Detect the expected, discover the unexpected” was the founding principle of the field of visual analytics. This mantra implies human stakeholders, like a domain expert or data analyst, could leverage visual analytics techniques to seek answers to known unknowns and discover unknown unknowns in the course of the data sensemaking process. We argue that in the era of AI-driven automation, the roles of humans and machines (e.g., a machine learning model) need to be recalibrated in terms of a team. We posit that by realizing human-machine teams as a stakeholder unit, we can better achieve the best of both worlds: automation transparency and human reasoning efficacy. However, this also increases the burden on analysts and domain experts towards performing more cognitively demanding tasks than they are used to. In this paper, we reflect on the complementary roles in a human-machine team through the lens of cognitive psychology and map them to existing and emerging research in the visual analytics community. We discuss open questions around the nature of human agency and analyze the shared responsibilities in human-machine teams.