Sequence Braiding: Visual Overviews of Temporal Event Sequences and Attributes
Sara Di Bartolomeo, Yixuan Zhang, Fangfang Sheng, Cody Dunne
External link (DOI)
View presentation:2020-10-29T15:15:00ZGMT-0600Change your timezone on the schedule page
2020-10-29T15:15:00Z
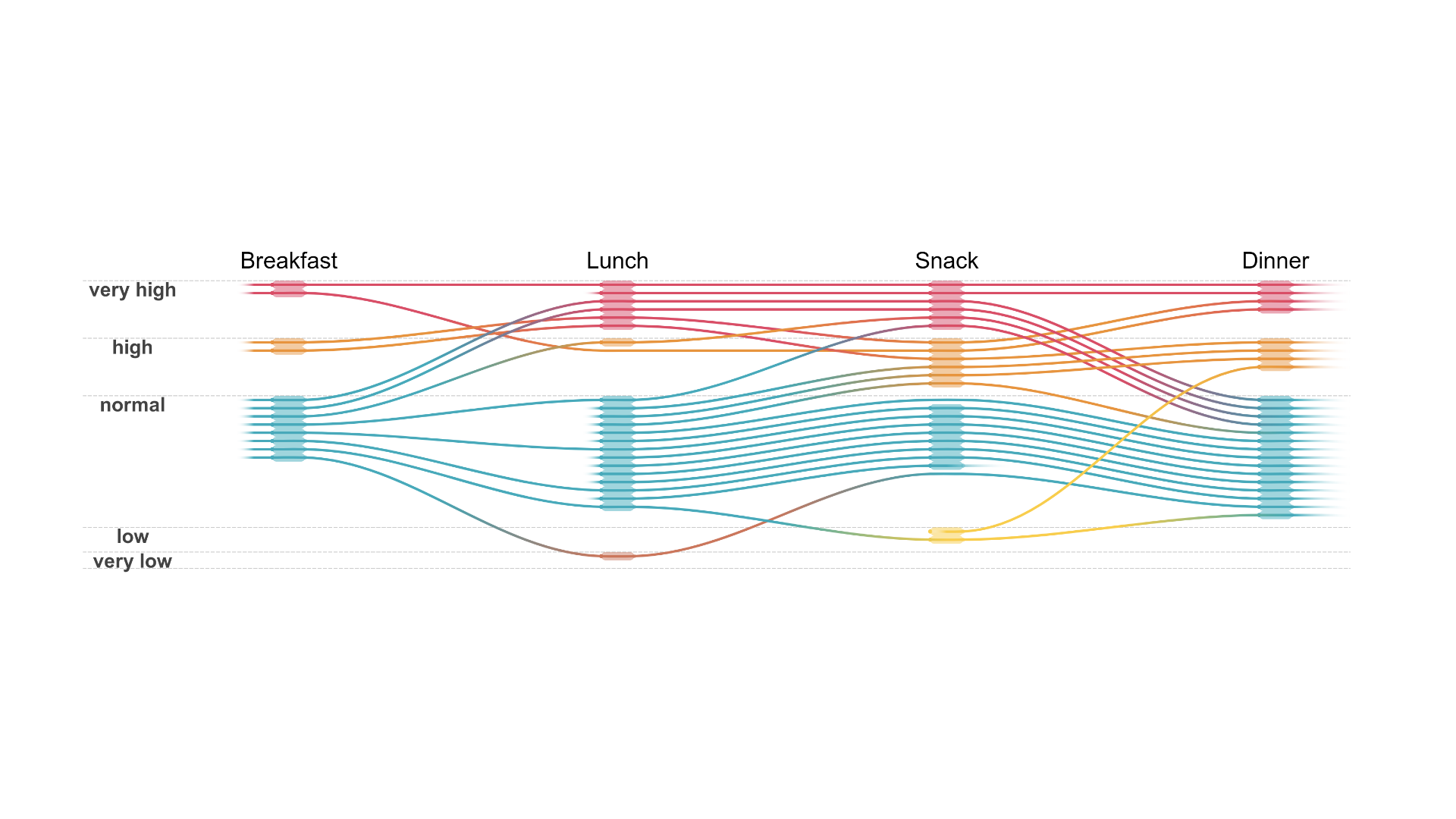
Fast forward
Direct link to video on YouTube: https://youtu.be/dPBnoCoGdq4
Keywords
Temporal Data, Algorithms, Application Motivated Visualization, Software Prototype, Life Sciences, Health, Medicine, Biology, Bioinformatics, Genomics, Domain Agnostic, Charts, Diagrams, and Plots
Abstract
Temporal event sequence alignment has been used in many domains to visualize nuanced changes and interactions over time. Existing approaches align one or two sentinel events. Overview tasks require examining all alignments of interest using interaction and time or juxtaposition of many visualizations. Furthermore, any event attribute overviews are not closely tied to sequence visualizations. We present Sequence Braiding, a novel overview visualization for temporal event sequences and attributes using a layered directed acyclic network. Sequence Braiding visually aligns many temporal events and attribute groups simultaneously and supports arbitrary ordering, absence, and duplication of events. In a controlled experiment we compare Sequence Braiding and IDMVis on user task completion time, correctness, error, and confidence. Our results provide good evidence that users of Sequence Braiding can understand high-level patterns and trends faster and with similar error.