HypoML: Visual Analysis for Hypothesis-based Evaluation of Machine Learning Models
Qianwen Wang, William Alexander, Jack Pegg, Huamin Qu, Min Chen
External link (DOI)
View presentation:2020-10-28T14:15:00ZGMT-0600Change your timezone on the schedule page
2020-10-28T14:15:00Z
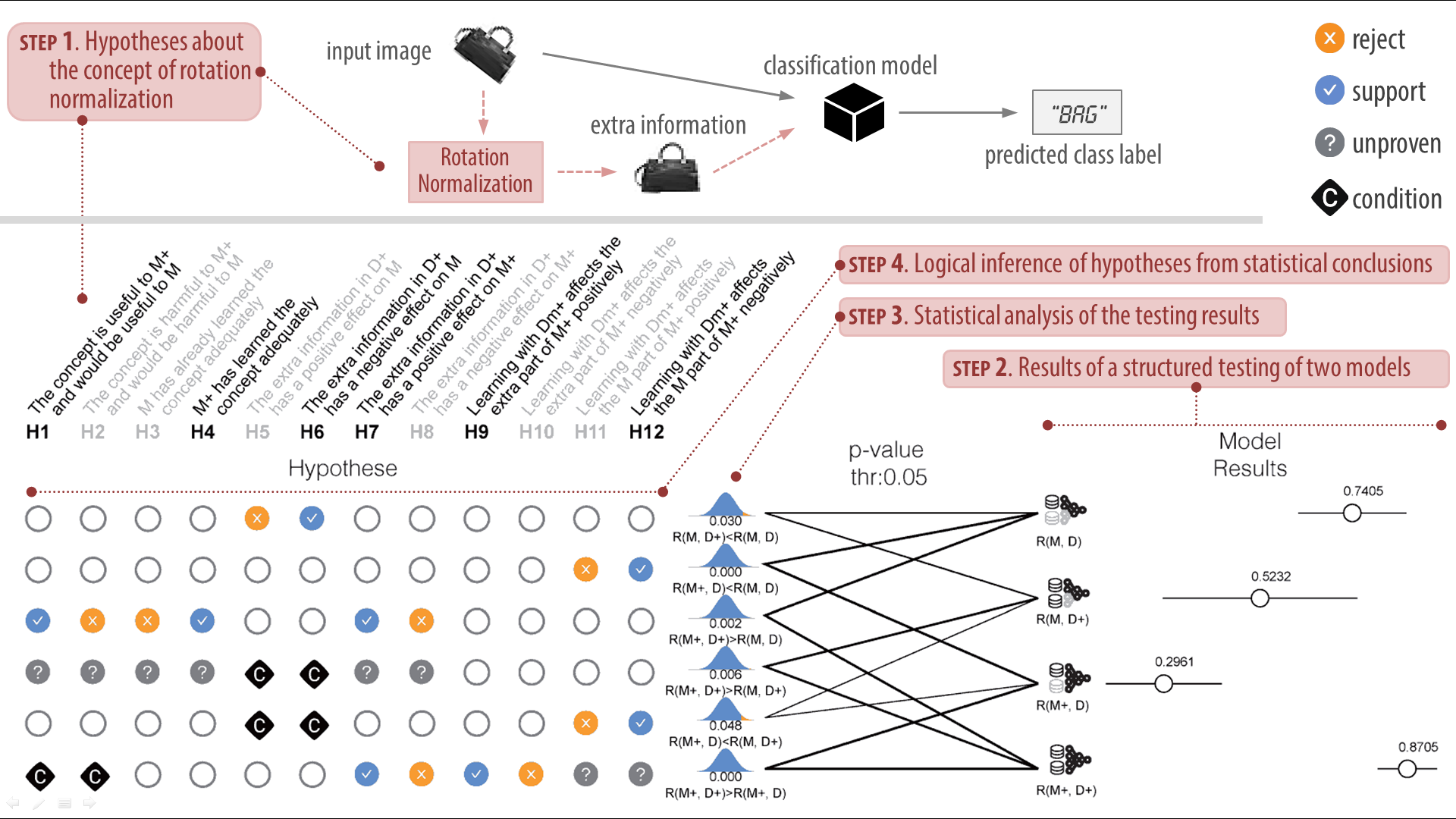
Fast forward
Direct link to video on YouTube: https://youtu.be/rf-amrd2Goc
Keywords
Visual analytics, model-developmental visualization, machine learning, neural network, hypothesis test, HypoML
Abstract
In this paper, we present a visual analytics tool for enabling hypothesis-based evaluation of machine learning (ML) models. We describe a novel ML-testing framework that combines the traditional statistical hypothesis testing (commonly used in empirical research) with logical reasoning about the conclusions of multiple hypotheses. The framework defines a controlled configuration for testing a number of hypotheses as to whether and how some extra information about a “concept” or “feature” may benefit or hinder an ML model. Because reasoning multiple hypotheses is not always straightforward, we provide HypoML as a visual analysis tool, with which, the multi-thread testing results are first transformed to analytical results using statistical and logical inferences, and then to a visual representation for rapid observation of the conclusions and the logical flow between the testing results and hypotheses. We have applied HypoML to a number of hypothesized concepts, demonstrating the intuitive and explainable nature of the visual analysis.