DRIL: Descriptive Rules by Interactive Learning
Fang Cao, Eli T Brown
External link (DOI)
View presentation:2020-10-30T14:10:00ZGMT-0600Change your timezone on the schedule page
2020-10-30T14:10:00Z
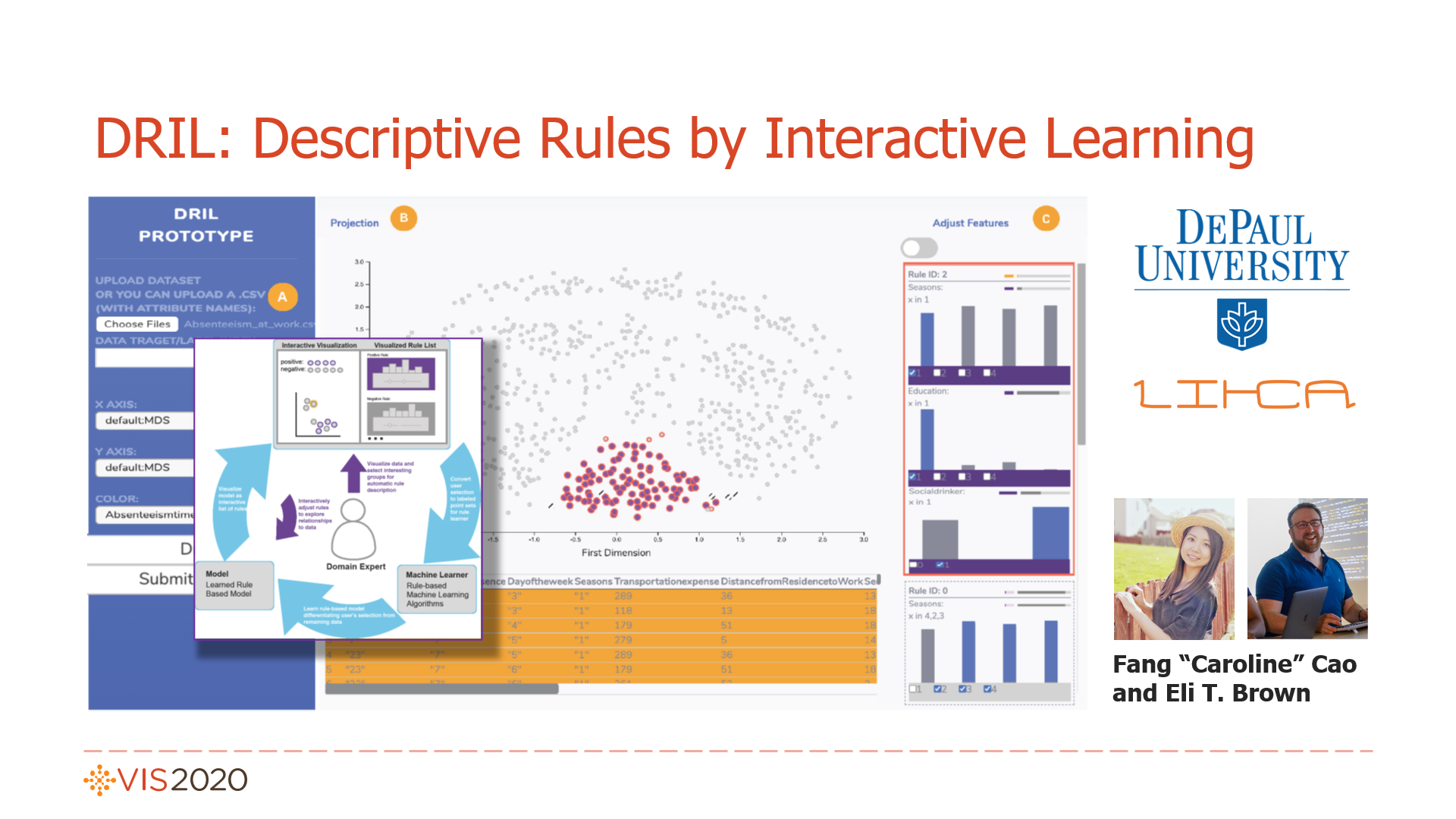
Fast forward
Direct link to video on YouTube: https://youtu.be/88f-JssKCSw
Keywords
human-in-the-loop, data characterization, rule learning
Abstract
Analyzing data is increasingly a part of jobs across industry, science and government, but data stakeholders are not necessarily experts in analytics. The human-in-the-loop (HIL) approach includes semantic interaction tools, which leverage machine learning behind the scenes to assist users with their tasks without engaging them directly with algorithms. One widely applicable model for how humans understand data is descriptive rules, which can characterize important attributes and simultaneously their crucial values or ranges. In this paper, we introduce an approach to help with data understanding via interactively and automatically generated rules. Our approach makes discerning the behavior of groups of interesting data efficient and simple by bridging the gap between machine learning methods for rule learning and the user experience of sensemaking through visual exploration. We have evaluated our approach with machine learning experiments to confirm an existing rule learning algorithm performs well in this interactive context even with a small amount of user input, and created a prototype system, DRIL (Descriptive Rules by Interactive Learning), to demonstrate its capability through a case study.