Geo-Context Aware Study of Vision-Based Autonomous Driving Models and Spatial Video Data
Suphanut Jamonnak, Ye Zhao, Xinyi Huang, Md Amiruzzaman
External link (DOI)
View presentation:2021-10-28T15:00:00ZGMT-0600Change your timezone on the schedule page
2021-10-28T15:00:00Z
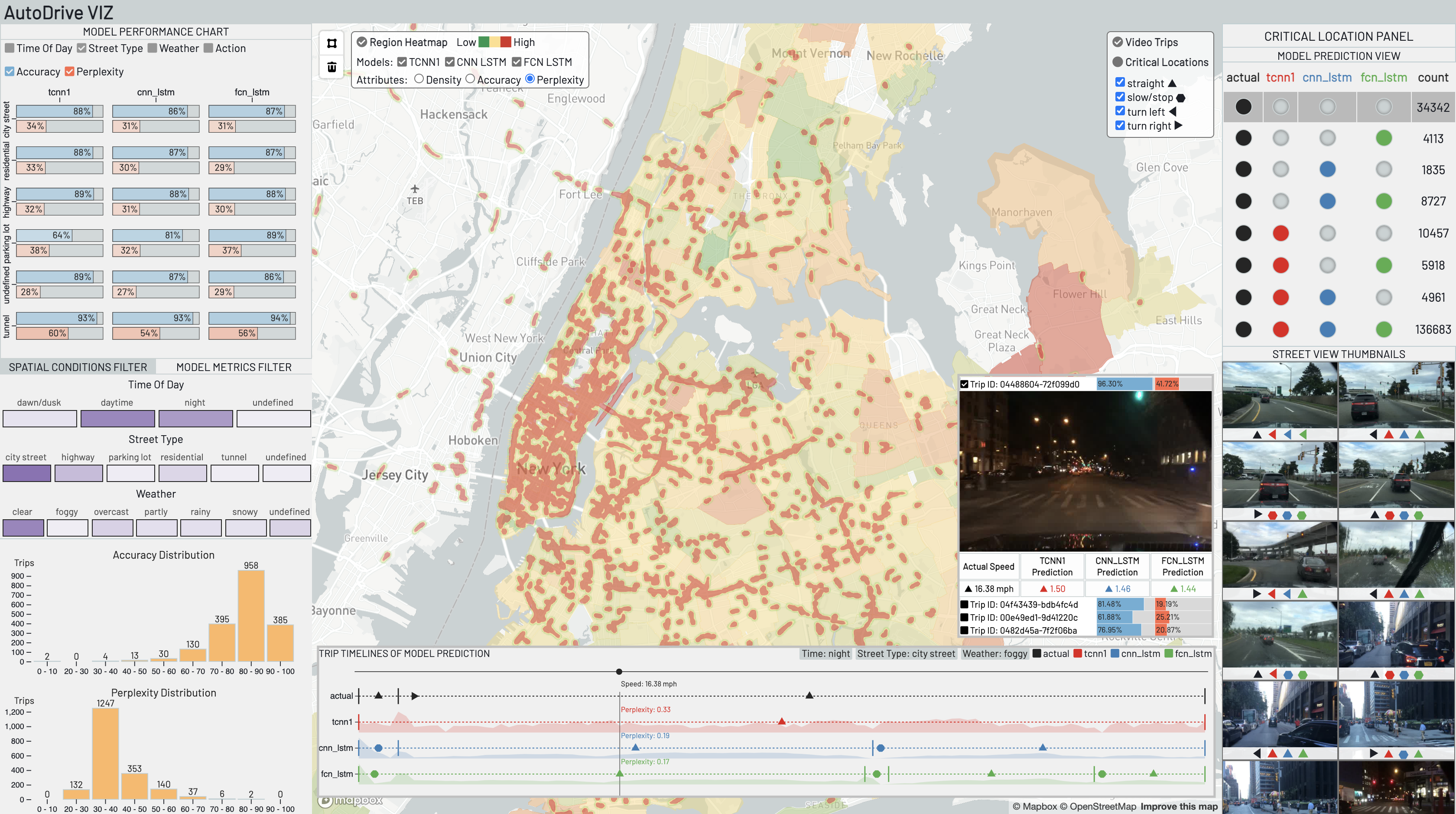
Fast forward
Direct link to video on YouTube: https://youtu.be/Y8bN-6G3Vko
Abstract
Vision-based deep learning (DL) methods have achieved success in learning autonomous driving models from large scale crowd-sourced video datasets. They are trained to predict instantaneous driving behaviors from video data captured by on-vehicle cameras. In this paper, we develop a geo-context aware visualization system for the study of Autonomous Driving Model (ADM) predictions together with large scale ADM video data. The visual study is seamlessly integrated with the geographical environment by combining DL model performance with geospatial visualization techniques. Model performance measures can be studied together with a set of geo-spatial attributes over map views. Users can also discover and compare prediction behaviors of multiple DL models in both city-wide and street-level analysis, together with road images and video contents. Therefore, the system provides a new visual exploration platform for DL model designers in autonomous driving. Use cases and domain expert evaluation show the utility and effectiveness of the visualization system.