Visual Cascade Analytics of Large-scale Spatiotemporal Data
Zikun Deng, Di Weng, Yuxuan Liang, Jie Bao, Yu Zheng, Tobias Schreck, Mingliang Xu, Yingcai Wu
External link (DOI)
View presentation:2021-10-28T16:00:00ZGMT-0600Change your timezone on the schedule page
2021-10-28T16:00:00Z
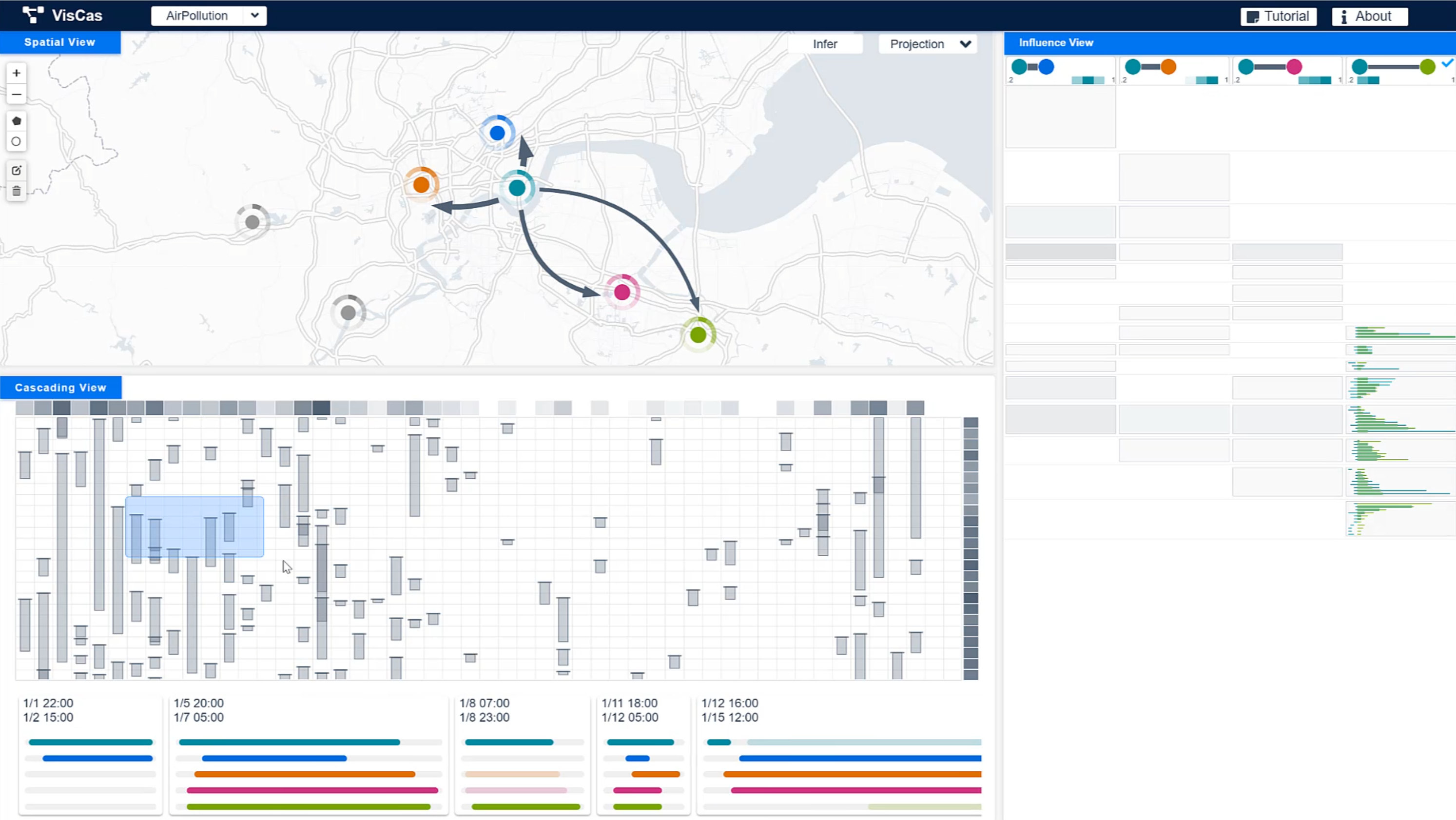
Fast forward
Direct link to video on YouTube: https://youtu.be/vlTkBlKQSt8
Keywords
Spatial cascade, pattern mining, spatiotemporal data
Abstract
Many spatiotemporal events can be viewed as contagions. These events implicitly propagate across space and time by following cascading patterns, expanding their influence, and generating event cascades that involve multiple locations. Analyzing such cascading processes presents valuable implications in various urban applications, such as traffic planning and pollution diagnostics. Motivated by the limited capability of the existing approaches in mining and interpreting cascading patterns, we propose a visual analytics system called VisCas. VisCas combines an inference model with interactive visualizations and empowers analysts to infer and interpret the latent cascading patterns in the spatiotemporal context. To develop VisCas, we address three major challenges, 1) generalized pattern inference, 2) implicit influence visualization, and 3) multifaceted cascade analysis. For the first challenge, we adapt the state-of-the-art cascading network inference technique to general urban scenarios, where cascading patterns can be reliably inferred from large-scale spatiotemporal data. For the second and third challenges, we assemble a set of effective visualizations to support location navigation, influence inspection, and cascading exploration, and facilitate the in-depth cascade analysis. We design a novel influence view based on a three-fold optimization strategy for analyzing the implicit influences of the inferred patterns. We demonstrate the capability and effectiveness of VisCas with two case studies conducted on real-world traffic congestion and air pollution datasets with domain experts.