HPCClusterScape: Increasing Transparency and Efficiency of Shared High-Performance Computing Clusters for Large-scale AI Models
Heungseok Park, Aeree Cho, Hyojun Jeon, Hayoung Lee, Youngil Yang, Sungjae Lee, Heungsub Lee, Jaegul Choo
Room: 106
2023-10-23T03:00:00ZGMT-0600Change your timezone on the schedule page
2023-10-23T03:00:00Z
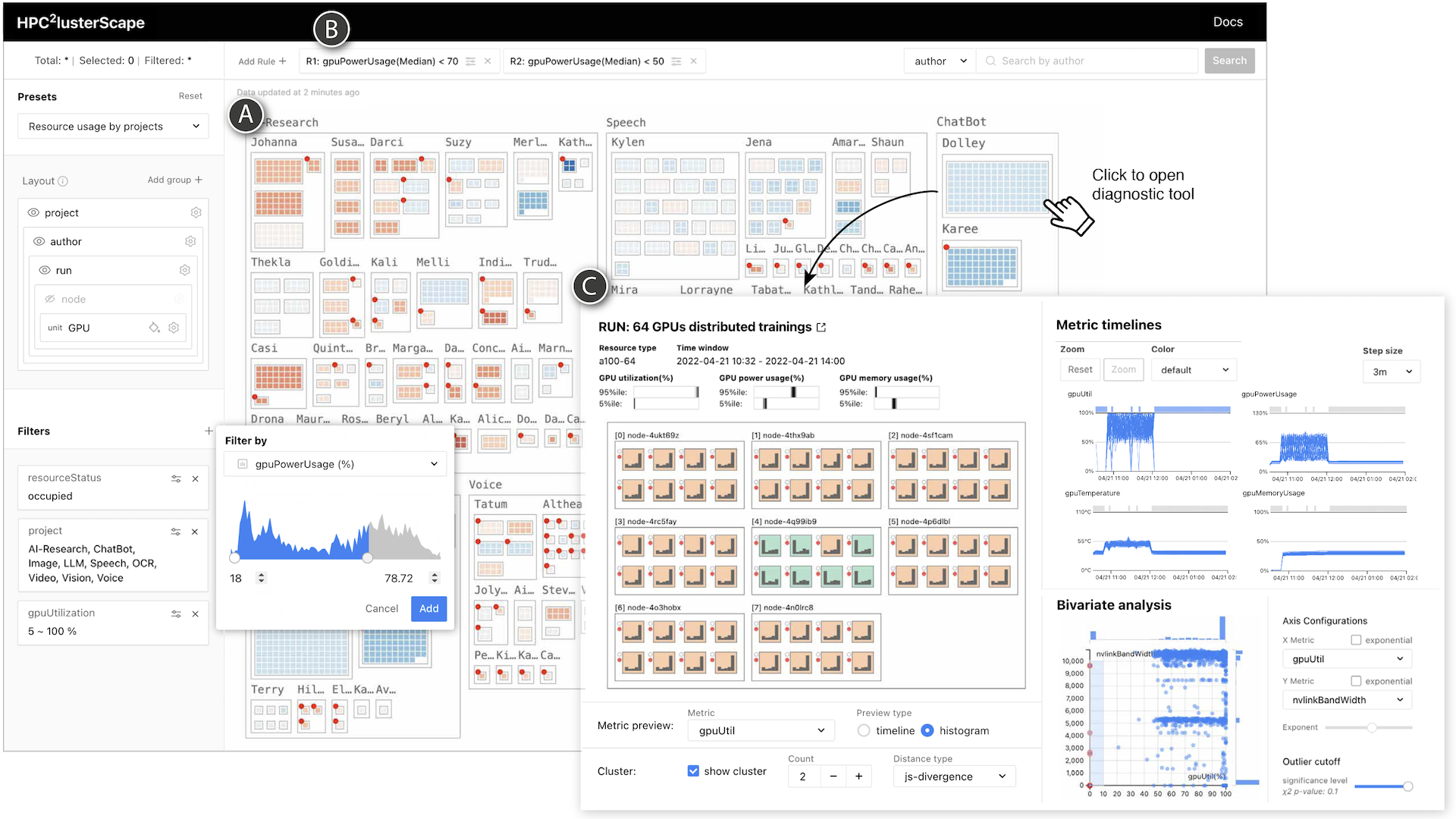
Fast forward
Full Video
Abstract
The emergence of large-scale AI models, like GPT-4, has significantly impacted academia and industry, driving the demand for high-performance computing (HPC) to accelerate workloads. To address this, we present HPCClusterScape, a visualization system that enhances the efficiency and transparency of shared HPC clusters for large-scale AI models. HPCClusterScape provides a comprehensive overview of system-level (e.g., partitions, hosts, and workload status) and application-level (e.g., identification of experiments and researchers) information, allowing HPC operators and machine learning researchers to monitor resource utilization and identify issues through customizable violation rules. The system includes diagnostic tools to investigate workload imbalances and synchronization bottlenecks in large-scale distributed deep learning experiments. Deployed in industrial-scale HPC clusters, HPCClusterScape incorporates user feedback and meets specific requirements. This paper outlines the challenges and prerequisites for efficient HPC operation, introduces the interactive visualization system, and highlights its contributions in addressing pain points and optimizing resource utilization in shared HPC clusters.