My Model is Unfair, Do People Even Care? Visual Design Affects Trust and Perceived Bias in Machine Learning
Aimen Gaba, Zhanna Kaufman, Jason Cheung, Marie Shvakel, Kyle Wm Hall, Yuriy Brun, Cindy Xiong Bearfield
DOI: 10.1109/TVCG.2023.3327192
Room: 109
2023-10-25T00:21:00ZGMT-0600Change your timezone on the schedule page
2023-10-25T00:21:00Z
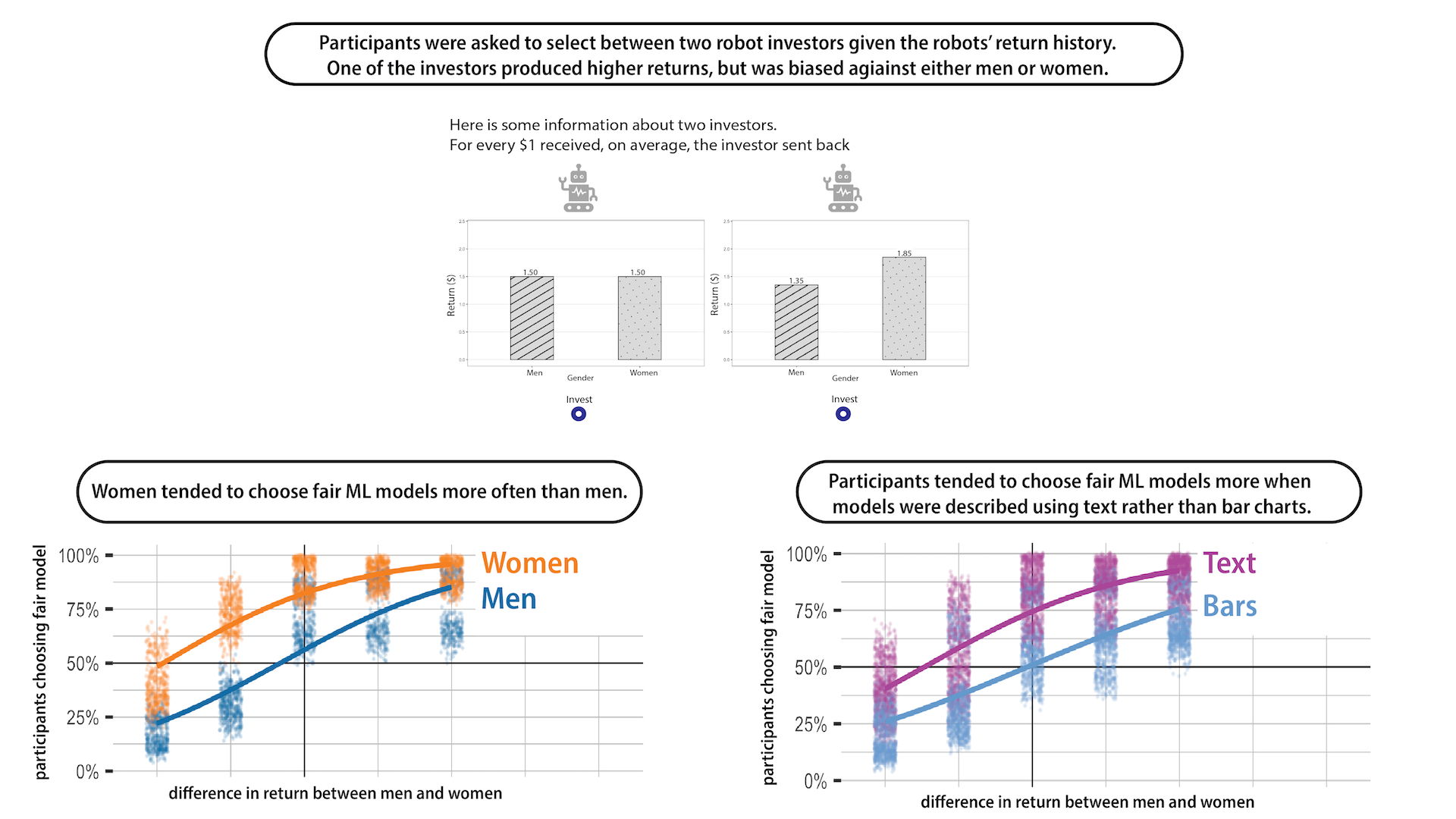
Fast forward
Full Video
Keywords
machine learning, fairness, bias, trust, visual design, gender, human-subjects studies
Abstract
Machine learning technology has become ubiquitous, but, unfortunately, often exhibits bias. As a consequence, disparate stakeholders need to interact with and make informed decisions about using machine learning models in everyday systems. Visualization technology can support stakeholders in understanding and evaluating trade-offs between, for example, accuracy and fairness of models. This paper aims to empirically answer "Can visualization design choices affect a stakeholder's perception of model bias, trust in a model, and willingness to adopt a model?'" Through a series of controlled, crowd-sourced experiments with more than 1,500 participants, we identify a set of strategies people follow in deciding which models to trust. Our results show that men and women prioritize fairness and performance differently and that visual design choices significantly affect that prioritization. For example, women trust fairer models more often than men do, participants value fairness more when it is explained using text than as a bar chart, and being explicitly told a model is biased has a bigger impact than showing past biased performance. We test the generalizability of our results by comparing the effect of multiple textual and visual design choices and offer potential explanations of the cognitive mechanisms behind the difference in fairness perception and trust. Our research guides design considerations to support future work developing visualization systems for machine learning.