InkSight: Leveraging Sketch Interaction for Documenting Chart Findings in Computational Notebooks
Yanna Lin, Haotian Li, Leni Yang, Aoyu Wu, Huamin Qu
DOI: 10.1109/TVCG.2023.3327170
Room: 105
2023-10-26T00:33:00ZGMT-0600Change your timezone on the schedule page
2023-10-26T00:33:00Z
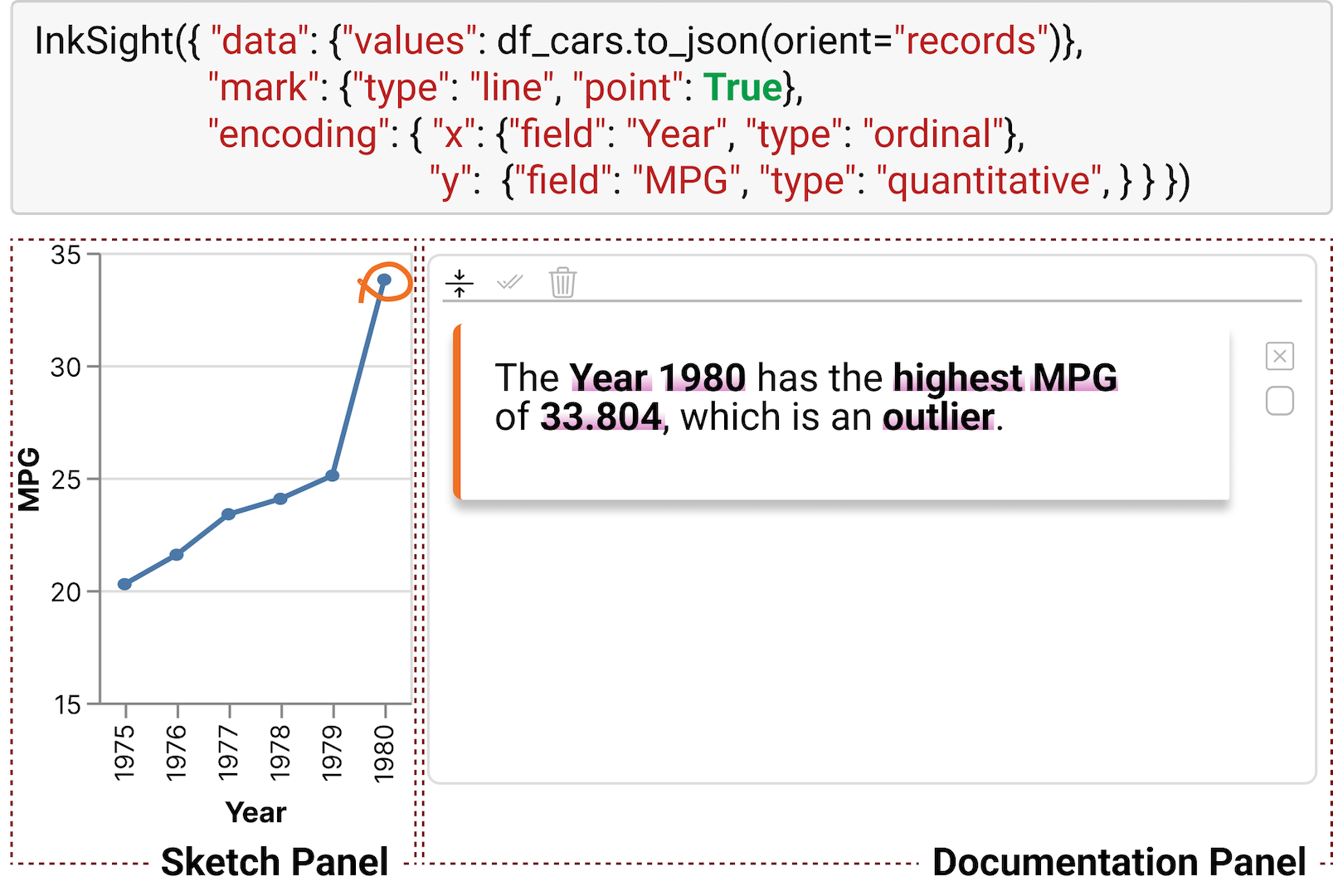
Fast forward
Full Video
Keywords
Computational Notebook, Sketch-based Interaction, Documentation, Visualization, Exploratory Data Analysis
Abstract
Computational notebooks have become increasingly popular for exploratory data analysis due to their ability to support data exploration and explanation within a single document. Effective documentation for explaining chart findings during the exploration process is essential as it helps recall and share data analysis. However, documenting chart findings remains a challenge due to its time-consuming and tedious nature. While existing automatic methods alleviate some of the burden on users, they often fail to cater to users' specific interests. In response to these limitations, we present InkSight, a mixed-initiative computational notebook plugin that generates finding documentation based on the user's intent. InkSight allows users to express their intent in specific data subsets through sketching atop visualizations intuitively. To facilitate this, we designed two types of sketches, i.e., open-path and closed-path sketch. Upon receiving a user's sketch, InkSight identifies the sketch type and corresponding selected data items. Subsequently, it filters data fact types based on the sketch and selected data items before employing existing automatic data fact recommendation algorithms to infer data facts. Using large language models (GPT-3.5), InkSight converts data facts into effective natural language documentation. Users can conveniently fine-tune the generated documentation within InkSight. A user study with 12 participants demonstrated the usability and effectiveness of InkSight in expressing user intent and facilitating chart finding documentation.