Knowledge Graphs in Practice: Characterizing their Users, Challenges, and Visualization Opportunities
Harry Li, Gabriel Appleby, Camelia D. Brumar, Remco Chang, Ashley Suh
Room: 105
2023-10-25T05:33:00ZGMT-0600Change your timezone on the schedule page
2023-10-25T05:33:00Z
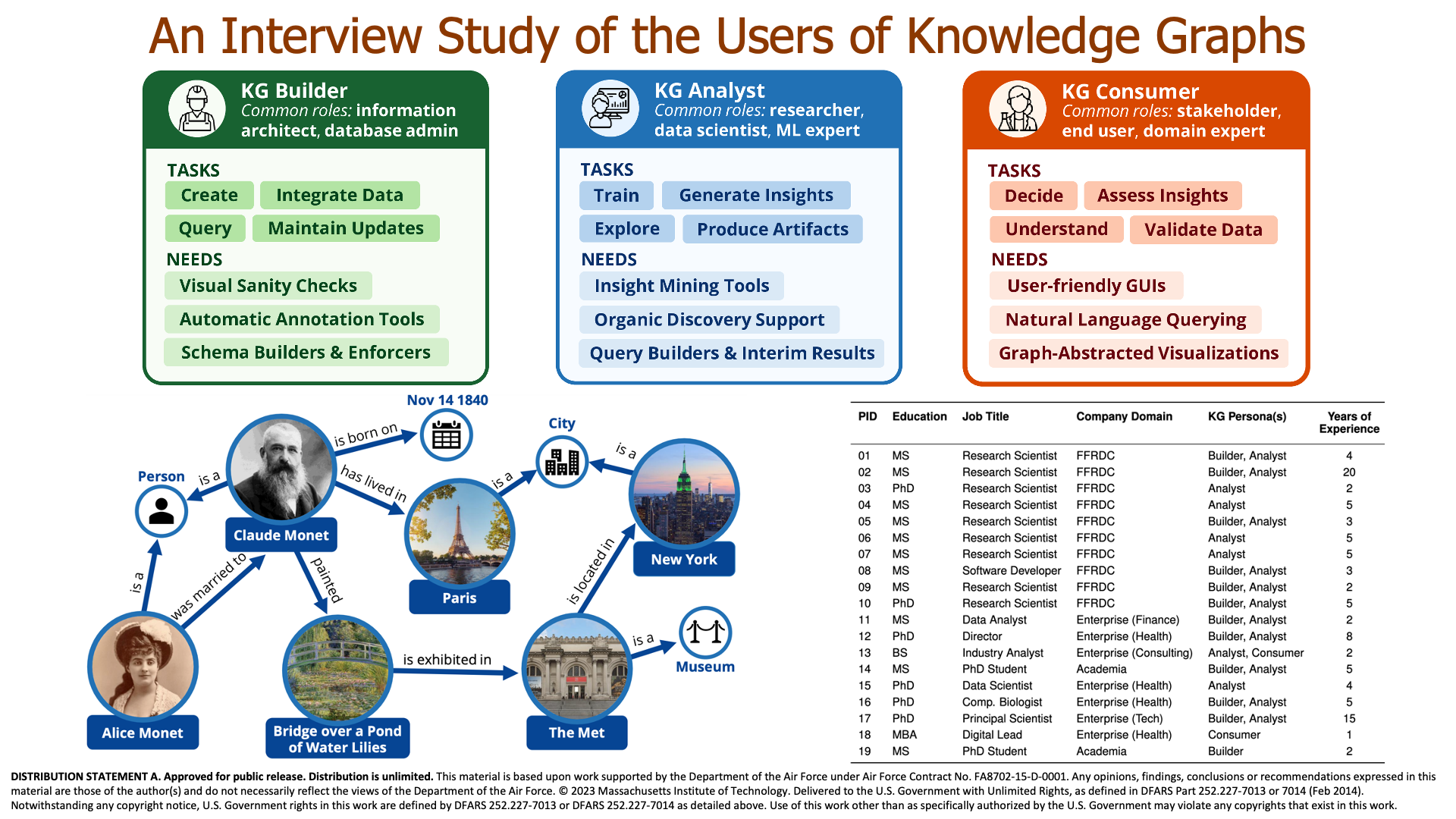
Fast forward
Full Video
Keywords
Knowledge graphs, visualization techniques and methodologies, human factors, visual communication
Abstract
This study presents insights from interviews with nineteen Knowledge Graph (KG) practitioners who work in both enterprise and academic settings on a wide variety of use cases. Through this study, we identify critical challenges experienced by KG practitioners when creating, exploring, and analyzing KGs that could be alleviated through visualization design. Our findings reveal three major personas among KG practitioners -- KG Builders, Analysts, and Consumers -- each of whom have their own distinct expertise and needs. We discover that KG Builders would benefit from schema enforcers, while KG Analysts need customizable query builders that provide interim query results. For KG Consumers, we identify a lack of efficacy for node-link diagrams, and the need for tailored domain-specific visualizations to promote KG adoption and comprehension. Lastly, we find that implementing KGs effectively in practice requires both technical and social solutions that are not addressed with current tools, technologies, and collaborative workflows. From the analysis of our interviews, we distill several visualization research directions to improve KG usability, including knowledge cards that balance digestibility and discoverability, timeline views to track temporal changes, interfaces that support organic discovery, and semantic explanations for AI and machine learning predictions.