TransforLearn: Interactive Visual Tutorial for the Transformer Model
Lin Gao, Zekai Shao, Ziqin LUO, Haibo Hu, Cagatay Turkay, Siming Chen
DOI: 10.1109/TVCG.2023.3327353
Room: 109
2023-10-25T22:00:00ZGMT-0600Change your timezone on the schedule page
2023-10-25T22:00:00Z
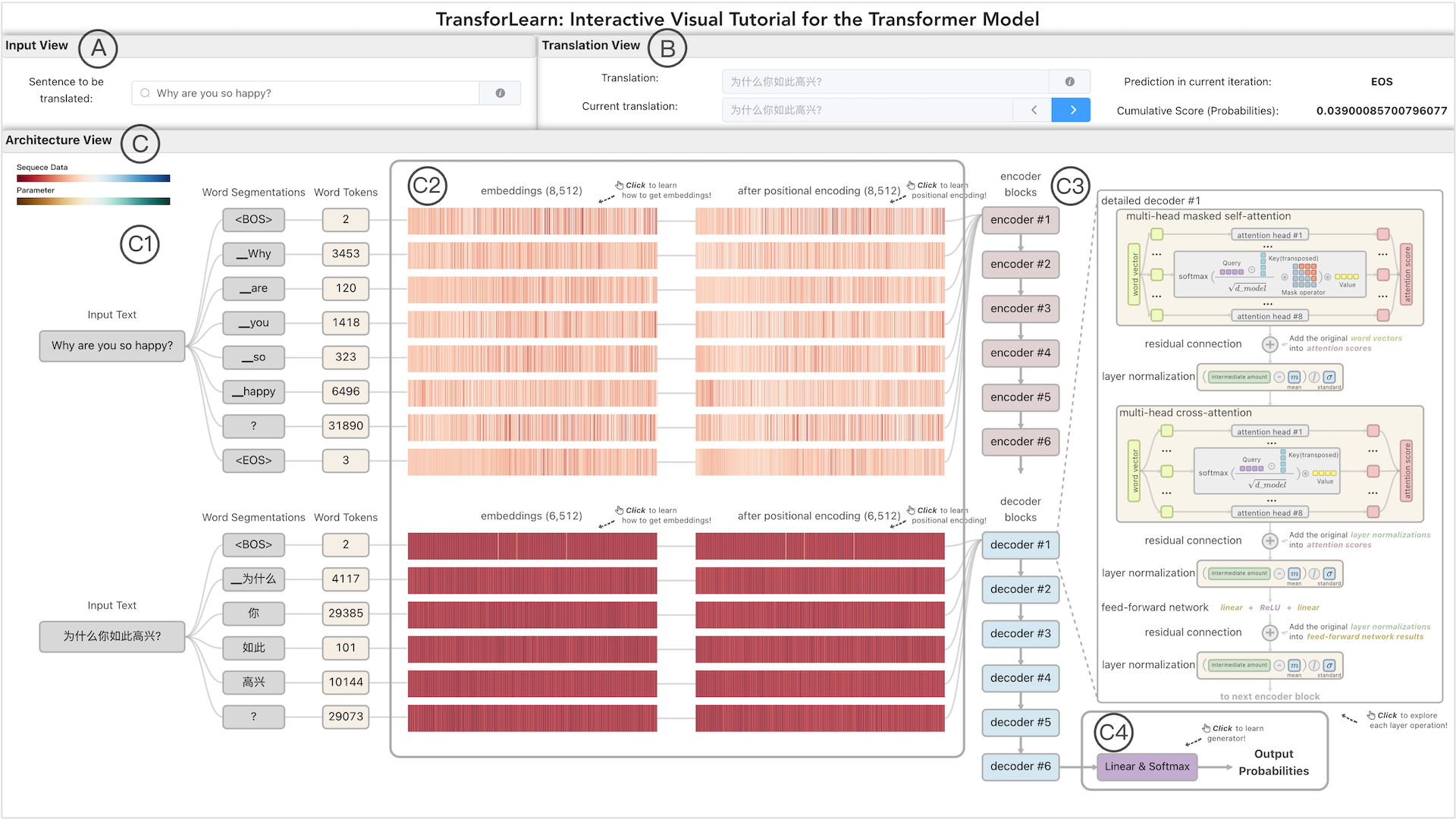
Fast forward
Full Video
Keywords
Deep learning, Transformer, Visual tutorial, Explorable explanations
Abstract
The widespread adoption of Transformers in deep learning, serving as the core framework for numerous large-scale language models, has sparked significant interest in understanding their underlying mechanisms. However, beginners face difficulties in comprehending and learning Transformers due to its complex structure and abstract data representation. We present TransforLearn, the first interactive visual tutorial designed for deep learning beginners and non-experts to comprehensively learn about Transformers. TransforLearn supports interactions for architecture-driven exploration and task-driven exploration, providing insight into different levels of model details and their working processes. It accommodates interactive views of each layer's operation and mathematical formula, helping users to understand the data flow of long text sequences. By altering the current decoder-based recursive prediction results and combining the downstream task abstractions, users can deeply explore model processes. Our user study revealed that the interactions of TransforLearn are positively received. We observe that TransforLearn facilitates users' accomplishment of study tasks and a grasp of key concepts in Transformer effectively.