HealthPrism: A Visual Analytics System for Exploring Children's Physical and Mental Health Profiles with Multimodal Data
Zhihan Jiang, Handi Chen, Rui Zhou, Jing Deng, Xinchen Zhang, Running Zhao, Cong Xie, Yifang Wang, Edith Ngai
DOI: 10.1109/TVCG.2023.3326943
Room: 105
2023-10-26T04:45:00ZGMT-0600Change your timezone on the schedule page
2023-10-26T04:45:00Z
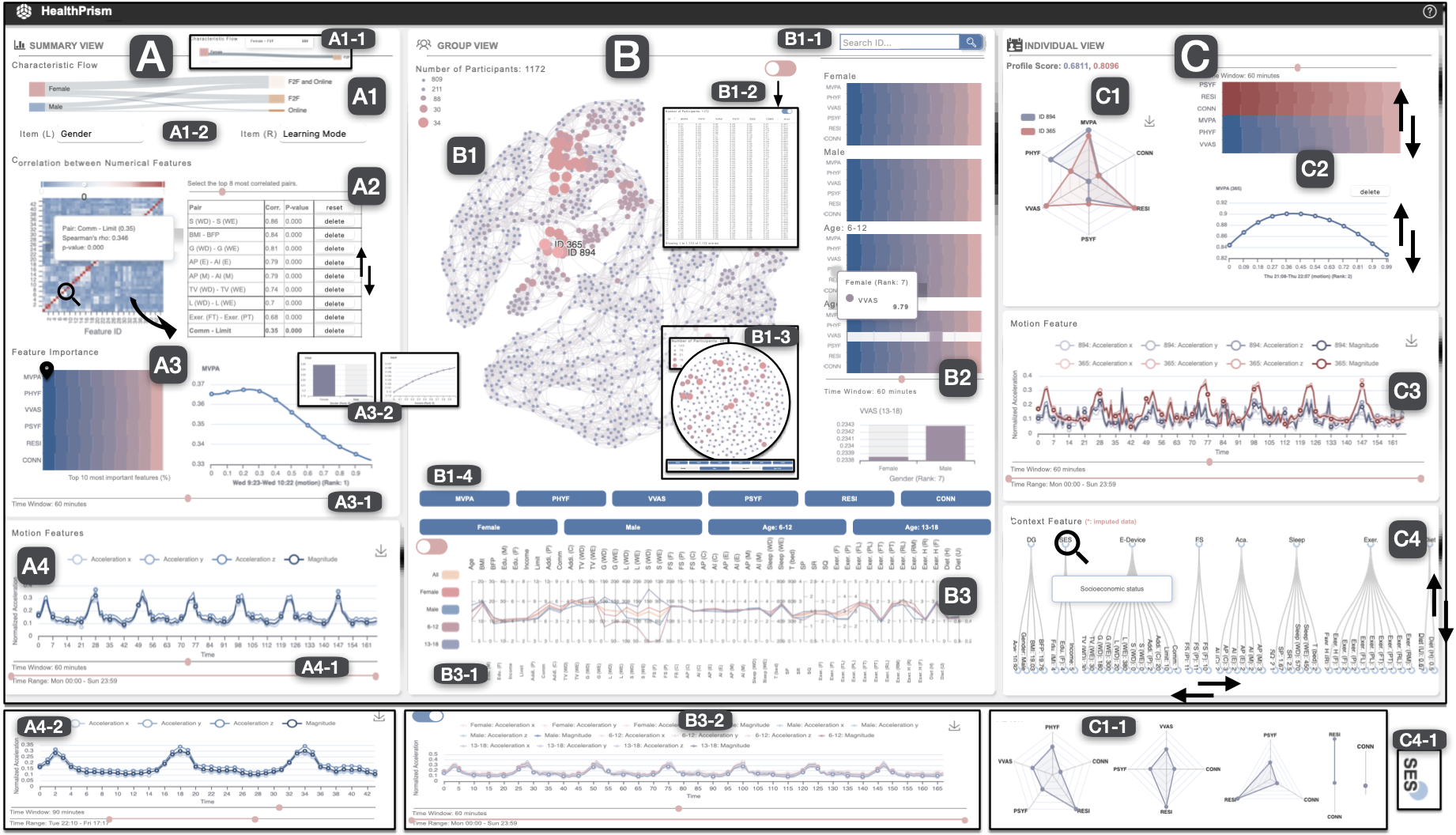
Fast forward
Full Video
Keywords
Visual Analytics, Health Profiling, Multimodal Learning, Context Data, Motion Data
Abstract
The correlation between children’s personal and family characteristics (e.g., demographics and socioeconomic status) and their physical and mental health status has been extensively studied across various research domains, such as public health, medicine, and data science. Such studies can provide insights into the underlying factors affecting children’s health and aid in the development of targeted interventions to improve their health outcomes. However, with the availability of multiple data sources, including context data (i.e., the background information of children) and motion data (i.e., sensor data measuring activities of children), new challenges have arisen due to the large-scale, heterogeneous, and multimodal nature of the data. Existing statistical hypothesis-based and learning model-based approaches have been inadequate for comprehensively analyzing the complex correlation between multimodal features and multi-dimensional health outcomes due to the limited information revealed. In this work, we first distill a set of design requirements from multiple levels through conducting a literature review and iteratively interviewing 11 experts from multiple domains (e.g., public health and medicine). Then, we propose HealthPrism, an interactive visual and analytics system for assisting researchers in exploring the importance and influence of various context and motion features on children’s health status from multi-level perspectives. Within HealthPrism, a multimodal learning model with a gate mechanism is proposed for health profiling and cross-modality feature importance comparison. A set of visualization components is designed for experts to explore and understand multimodal data freely. We demonstrate the effectiveness and usability of HealthPrism through quantitative evaluation of the model performance, case studies, and expert interviews in associated domains.