Roses Have Thorns: Understanding the Downside of Oncological Care Delivery Through Visual Analytics and Sequential Rule Mining
Carla Gabriela Floricel, Andrew Wentzel, Abdallah Mohamed, Clifton David Fuller, Guadalupe Canahuate, G. Elisabeta Marai
DOI: 10.1109/TVCG.2023.3326939
Room: 105
2023-10-26T05:09:00ZGMT-0600Change your timezone on the schedule page
2023-10-26T05:09:00Z
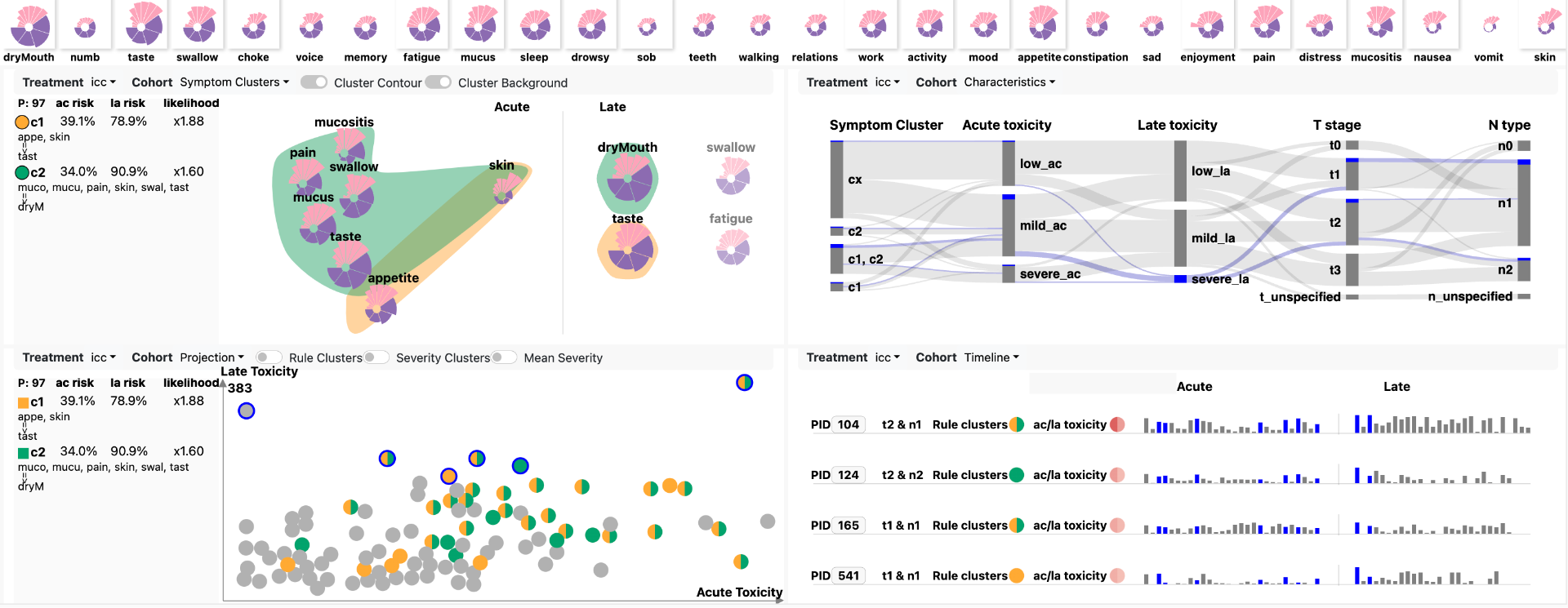
Fast forward
Full Video
Keywords
Temporal Data; Life Sciences; Mixed Initiative Human-Machine Analysis; Data Clustering and Aggregation
Abstract
Personalized head and neck cancer therapeutics have greatly improved survival rates for patients, but are often leading to understudied long-lasting symptoms which affect quality of life. Sequential rule mining (SRM) is a promising unsupervised machine learning method for predicting longitudinal patterns in temporal data which, however, can output many repetitive patterns that are difficult to interpret without the assistance of visual analytics. We present a data-driven, human-machine analysis visual system developed in collaboration with SRM model builders in cancer symptom research, which facilitates mechanistic knowledge discovery in large scale, multivariate cohort symptom data. Our system supports multivariate predictive modeling of post-treatment symptoms based on during-treatment symptoms. It supports this goal through an SRM, clustering, and aggregation back end, and a custom front end to help develop and tune the predictive models. The system also explains the resulting predictions in the context of therapeutic decisions typical in personalized care delivery. We evaluate the resulting models and system with an interdisciplinary group of modelers and head and neck oncology researchers. The results demonstrate that our system effectively supports clinical and symptom research.