A Unified Interactive Model Evaluation for Classification, Object Detection, and Instance Segmentation in Computer Vision
Changjian Chen, Yukai Guo, Fengyuan Tian, Shilong Liu, Weikai Yang, Zhaowei Wang, Jing Wu, Hang Su, Hanspeter Pfister, Shixia Liu
DOI: 10.1109/TVCG.2023.3326588
Room: 103
2023-10-24T22:48:00ZGMT-0600Change your timezone on the schedule page
2023-10-24T22:48:00Z
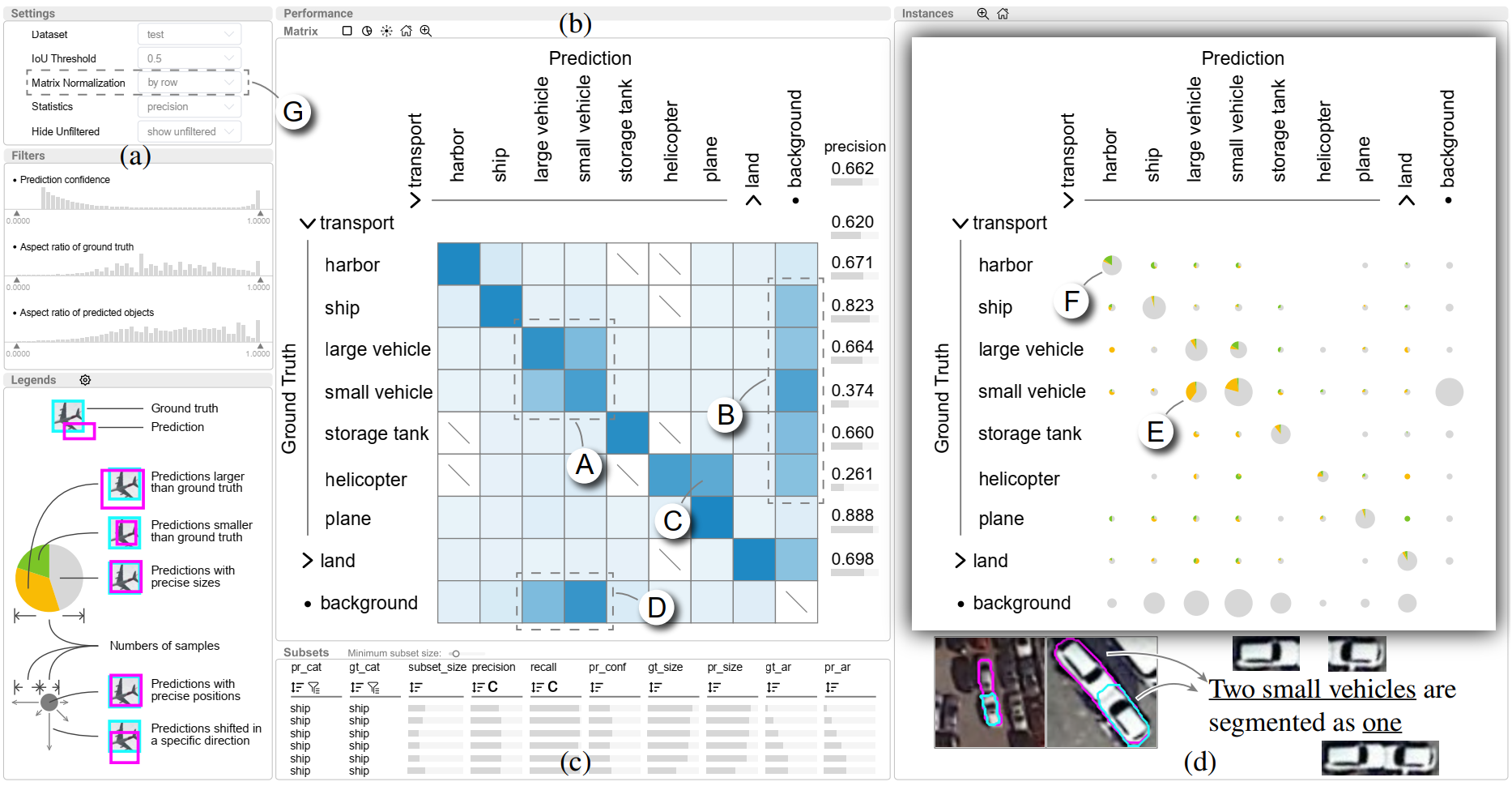
Fast forward
Full Video
Keywords
Model evaluation, computer vision, classification, object detection, instance segmentation
Abstract
Existing model evaluation tools mainly focus on evaluating classification models, leaving a gap in evaluating more complex models, such as object detection. In this paper, we develop an open-source visual analysis tool, Uni-Evaluator, to support a unified model evaluation for classification, object detection, and instance segmentation in computer vision. The key idea behind our method is to formulate both discrete and continuous predictions in different tasks as unified probability distributions. Based on these distributions, we develop 1) a matrix-based visualization to provide an overview of model performance; 2) a table visualization to identify the problematic data subsets where the model performs poorly; 3) a grid visualization to display the samples of interest. These visualizations work together to facilitate the model evaluation from a global overview to individual samples. Two case studies demonstrate the effectiveness of Uni-Evaluator in evaluating model performance and making informed improvements.