TROPHY: A Topologically Robust Physics-Informed Tracking Framework for Tropical Cyclone
Lin Yan, Hanqi Guo, Tom Peterka, Bei Wang Phillips, Jiali Wang
DOI: 10.1109/TVCG.2023.3326905
Room: 106
2023-10-26T04:45:00ZGMT-0600Change your timezone on the schedule page
2023-10-26T04:45:00Z
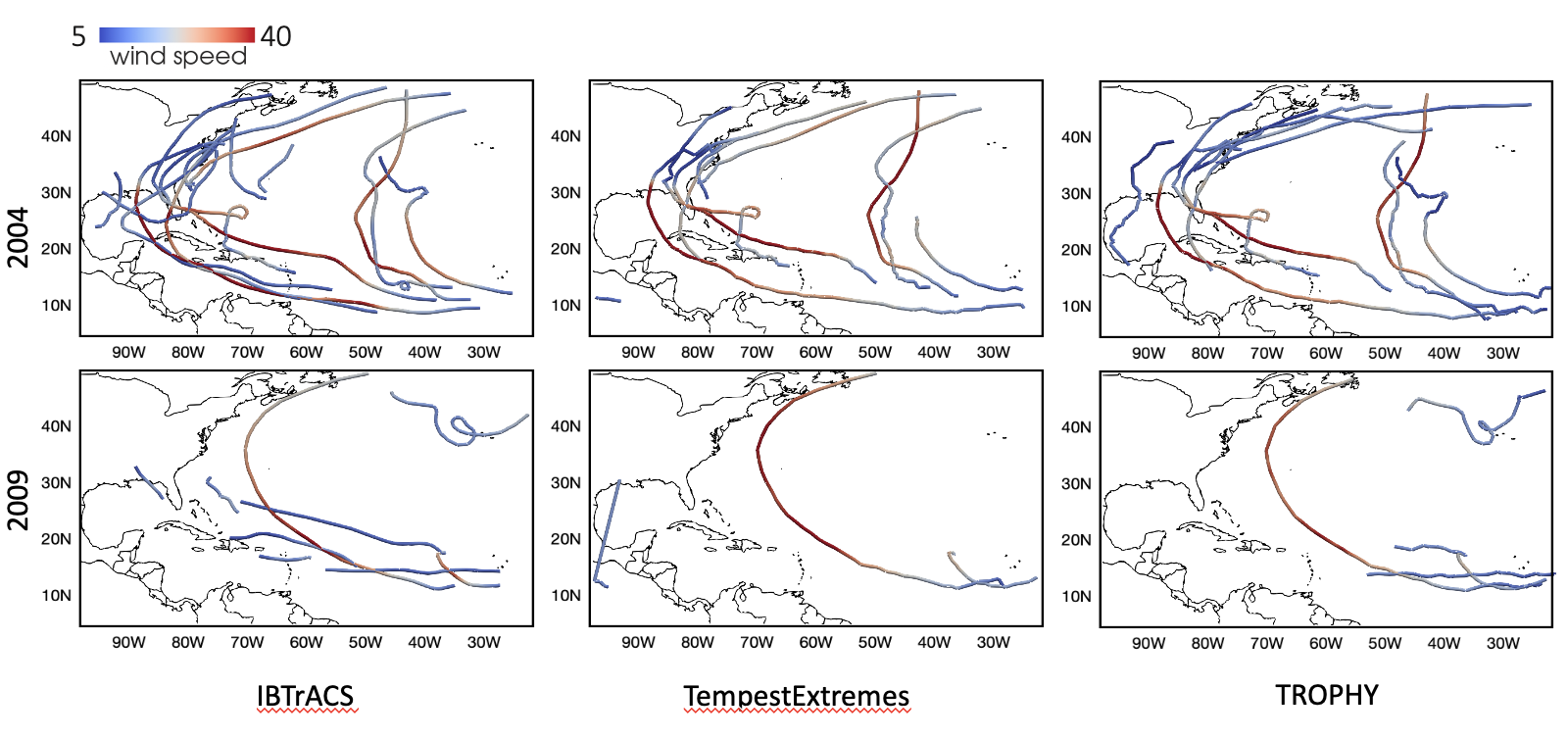
Fast forward
Full Video
Keywords
Feature tracking, robustness, topology-based methods in visualization, applications, climate science, tropical cyclones
Abstract
Tropical cyclones (TCs) are among the most destructive weather systems. Realistically and efficiently detecting and tracking TCs are critical for assessing their impacts and risks. In particular, the eye is a signature feature of a mature TC. Therefore, knowing the eyes' locations and movements is crucial for both operational weather forecasts and climate risk assessments. Recently, a multilevel robustness framework has been introduced to study the critical points of time-varying vector fields. The framework quantifies the robustness (i.e., structural stability) of critical points across varying neighborhoods. By relating the multilevel robustness with critical point tracking, the framework has demonstrated its potential in cyclone tracking. An advantage is that it identifies cyclonic features using only 2D wind vector fields, which is encouraging as most tracking algorithms require multiple dynamic and thermodynamic variables at different altitudes. A disadvantage is that the framework does not scale well computationally for datasets containing a large number of cyclones. This paper introduces a topologically robust physics-informed tracking framework (TROPHY) for TC tracking. The main idea is to integrate physical knowledge of TC to drastically improve the computational efficiency of multilevel robustness framework for large-scale climate datasets. First, during preprocessing, we propose a physics-informed feature selection strategy to filter 90% of critical points that are short-lived and have low stability, thus preserving good candidates for TC tracking. Second, during in-processing, we impose constraints during the multilevel robustness computation to focus only on physics-informed neighborhoods of TCs. We apply TROPHY to 30 years of 2D wind fields from reanalysis data in ERA5 and generate a number of TC tracks. In comparison with the observed tracks, we demonstrate that TROPHY can capture TC characteristics (e.g., frequency, intensity, duration, latitudes with maximum intensity, and genesis) that are comparable to and sometimes even better than a well-validated TC tracking algorithm that requires multiple dynamic and thermodynamic scalar fields.