The Rational Agent Benchmark for Data Visualization
Yifan Wu, Ziyang Guo, Michalis Mamakos, Jason Hartline, Jessica Hullman
DOI: 10.1109/TVCG.2023.3326513
Room: 109
2023-10-25T00:33:00ZGMT-0600Change your timezone on the schedule page
2023-10-25T00:33:00Z
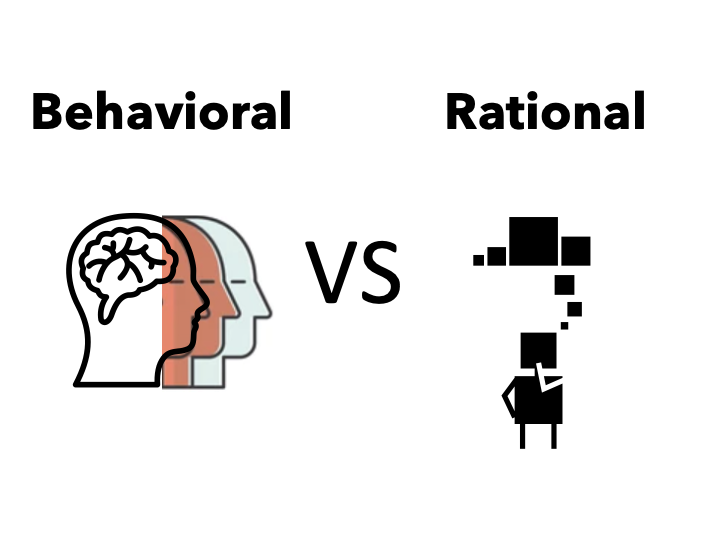
Fast forward
Full Video
Keywords
Evaluation, decision-making, rational agent, scoring rule
Abstract
Understanding how helpful a visualization is from experimental results is difficult because the observed performance is confounded with aspects of the study design, such as how useful the information that is visualized is for the task. We develop a rational agent framework for designing and interpreting visualization experiments. Our framework conceives two experiments with the same setup: one with behavioral agents (human subjects), and the other one with a hypothetical rational agent. A visualization is evaluated by comparing the expected performance of behavioral agents to that of a rational agent under different assumptions. Using recent visualization decision studies from the literature, we demonstrate how the framework can be used to pre-experimentally evaluate the experiment design by bounding the expected improvement in performance from having access to visualizations, and post-experimentally to deconfound errors of information extraction from errors of optimization, among other analyses.