ScatterUQ: Interactive Uncertainty Visualizations for Multiclass Deep Learning Problems
Harry Li, Steven Jorgensen, John Holodnak, Allan Wollaber
Room: 104
2023-10-26T22:54:00ZGMT-0600Change your timezone on the schedule page
2023-10-26T22:54:00Z
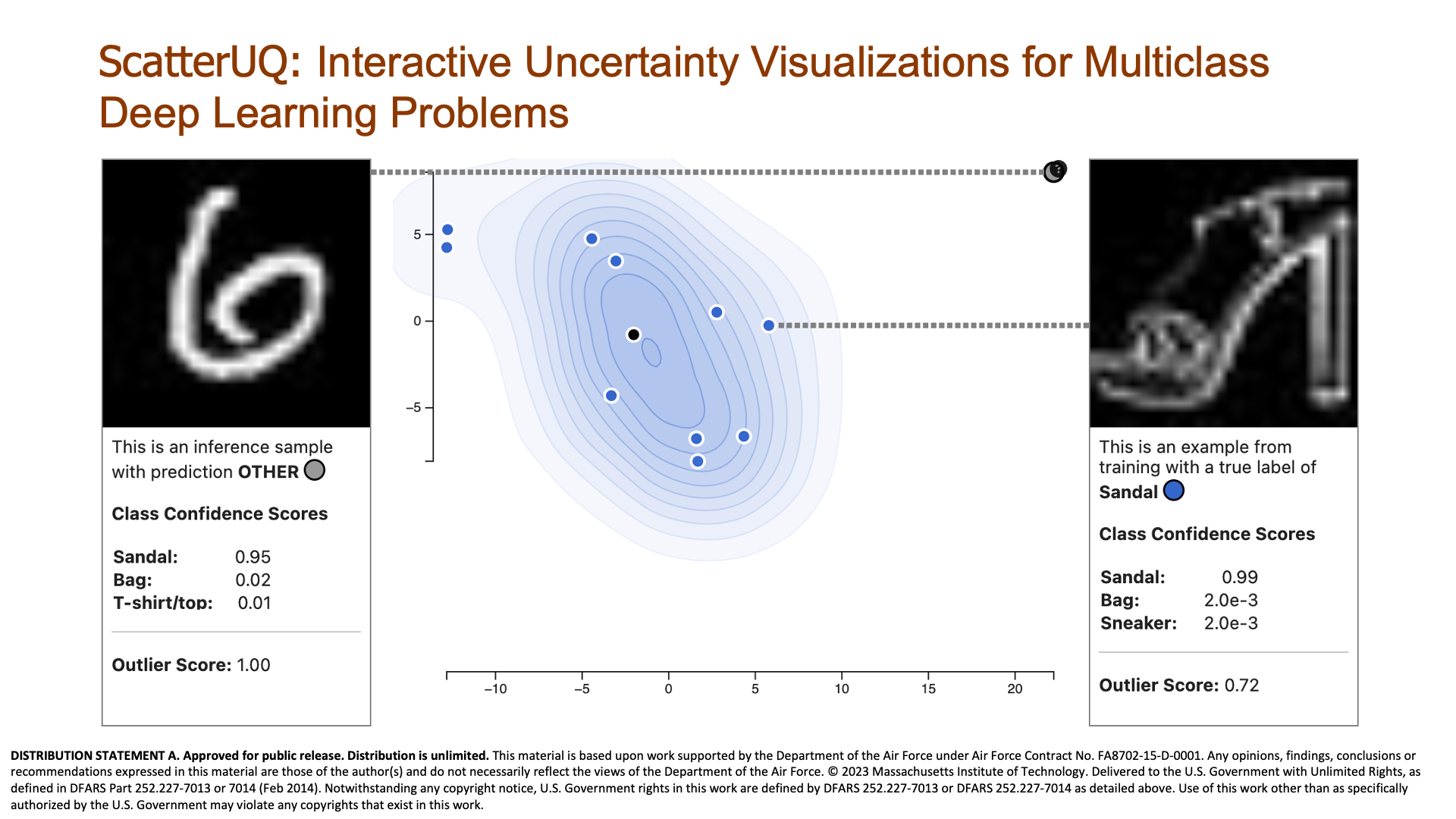
Fast forward
Full Video
Keywords
Uncertainty quantification - Machine learning -Dimensionality reduction - Visualization - Explainable AI
Abstract
Recently, uncertainty-aware deep learning methods for multiclass labeling problems have been developed that provide calibrated class prediction probabilities and out-of-distribution (OOD) indicators, letting machine learning (ML) consumers and engineers gauge a model's confidence in its predictions. However, this extra neural network prediction information is challenging to scalably convey visually for arbitrary data sources under multiple uncertainty contexts. To address these challenges, we present ScatterUQ, an interactive system that provides targeted visualizations to allow users to better understand model performance in context-driven uncertainty settings. ScatterUQ leverages recent advances in distance-aware neural networks, together with dimensionality reduction techniques, to construct robust, 2-D scatter plots explaining why a model predicts a test example to be (1) in-distribution and of a particular class, (2) in-distribution but unsure of the class, and (3) out-of-distribution. ML consumers and engineers can visually compare the salient features of test samples with training examples through the use of a ``hover callback'' to understand model uncertainty performance and decide follow up courses of action. We demonstrate the effectiveness of ScatterUQ to explain model uncertainty for a multiclass image classification on a distance-aware neural network trained on Fashion-MNIST and tested on Fashion-MNIST (in distribution) and MNIST digits (out of distribution), as well as a deep learning model for a cyber dataset. We quantitatively evaluate dimensionality reduction techniques to optimize our contextually driven UQ visualizations. Our results indicate that the ScatterUQ system should scale to arbitrary, multiclass datasets. Our code is available at https://github.com/mit-ll-responsible-ai/equine-webapp.