DataTales: Investigating the Use of Large Language Models for Authoring Data-Driven Articles
Nicole Sultanum, Arjun Srinivasan
Room: 104
2023-10-26T22:27:00ZGMT-0600Change your timezone on the schedule page
2023-10-26T22:27:00Z
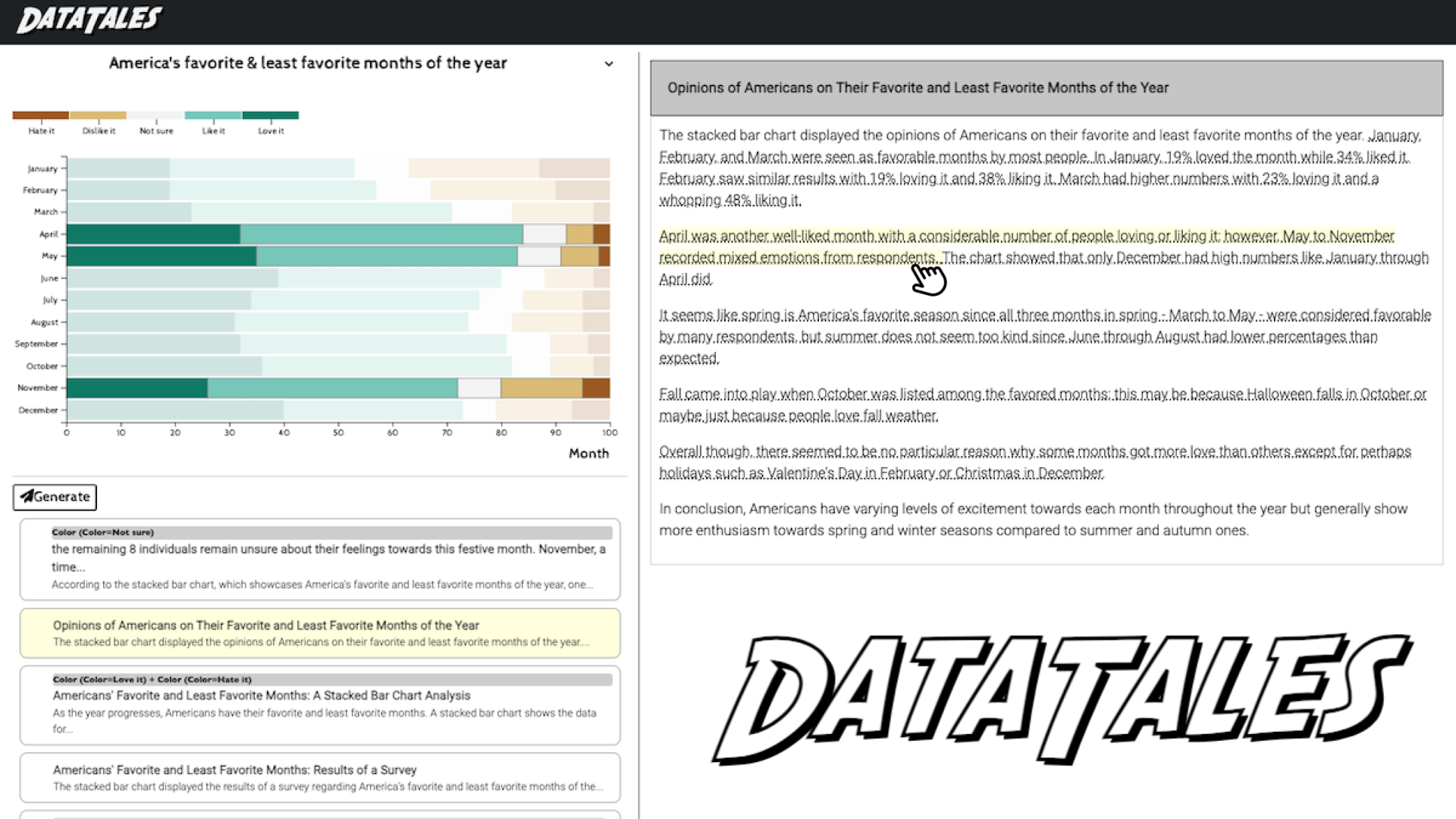
Fast forward
Full Video
Keywords
large language models; data-driven articles; data-driven authoring; data stories
Abstract
Authoring data-driven articles is a complex process requiring authors to not only analyze data for insights but also craft a cohesive narrative that effectively communicates the insights. Text generation capabilities of contemporary large language models (LLMs) present an opportunity to assist the authoring of data-driven articles and expedite the writing process. In this work, we investigate the feasibility and perceived value of leveraging LLMs to support authors of data-driven articles. We designed a prototype system, DataTales, that leverages a LLM to generate textual narratives accompanying a given chart. Using DataTales as a design probe, we conducted a qualitative study with 11 professionals to evaluate the concept, from which we distilled affordances and opportunities to further integrate LLMs as valuable data-driven article authoring assistants.