Effects of data distribution and granularity on color semantics for colormap data visualizations
Clementine Zimnicki, Chin Tseng, Danielle Albers Szafir, Karen Schloss
Room: 104
2023-10-24T22:09:00ZGMT-0600Change your timezone on the schedule page
2023-10-24T22:09:00Z
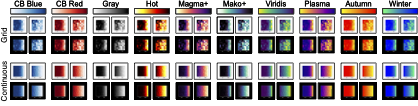
Fast forward
Full Video
Keywords
Visual reasoning, information visualization, colormap data visualizations, color cognition
Abstract
To create effective data visualizations, it helps to represent data using visual features in intuitive ways. When visualization designs match observer expectations, visualizations are easier to interpret. Prior work suggests that several factors influence such expectations. For example, the dark-is-more bias leads observers to infer that darker colors map to larger quantities, and the opaque-is-more bias leads them to infer that regions appearing more opaque (given the background color) map to larger quantities. Previous work suggested that the background color only plays a role if visualizations appear to vary in opacity. The present study challenges this claim. We hypothesized that the background color would modulate inferred mappings for colormaps that should not appear to vary in opacity (by previous measures) if the visualization appeared to have a “hole” that revealed the background behind the map (hole hypothesis). We found that spatial aspects of the map contributed to inferred mappings, though the effects were inconsistent with the hole hypothesis. Our work raises new questions about how spatial distributions of data influence color semantics in colormap data visualizations.