Concept Lens: Visually Analyzing the Consistency of Semantic Manipulation in GANs
Sangwon Jeong, Mingwei Li, Matthew Berger, Shusen Liu
Room: 104
2023-10-26T22:09:00ZGMT-0600Change your timezone on the schedule page
2023-10-26T22:09:00Z
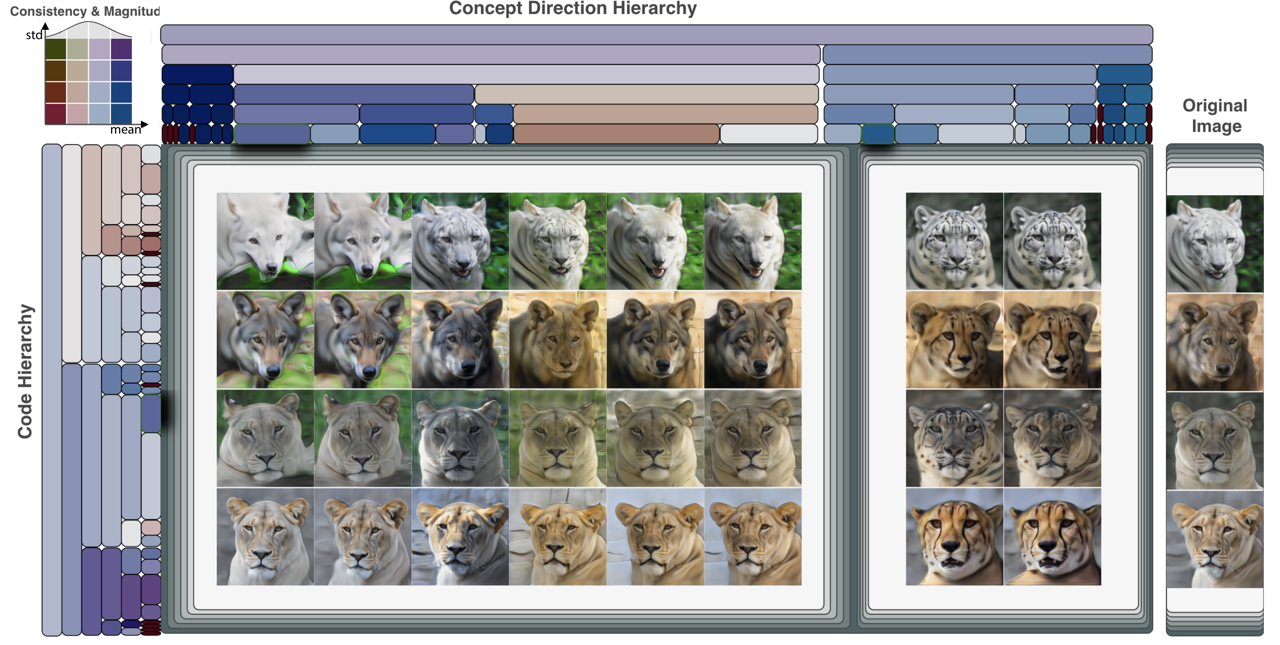
Fast forward
Full Video
Keywords
Generative model, Explainable AI, Latent space, Clustering, Bivariate color
Abstract
As applications of generative AI become mainstream, it is important to understand what generative models are capable of producing, and the extent to which one can predictably control their outputs. In this paper, we propose a visualization design, named Concept Lens, for jointly navigating the data distribution of a generative model, and concept manipulations supported by the model. Our work is focused on modern vision-based generative adversarial networks (GAN), and their learned latent spaces, wherein concept discovery has gained significant interest as a means of image manipulation. Concept Lens is designed to support users in understanding the diversity of a pro- vided set of concepts, the relationship between concepts, and the suitability of concepts to give semantic controls for image genera- tion. Key to our approach is the hierarchical grouping of concepts, generated images, and the associated joint exploration. We show how Concept Lens can reveal consistent semantic manipulations for editing images, while also serving as a diagnostic tool for studying the limitations and trade-offs of concept discovery methods.