ShortcutLens: A Visual Analytics Approach for Exploring Shortcuts in Natural Language Understanding Dataset
Zhihua Jin, Xingbo Wang, Furui Cheng, Chunhui Sun, Qun Liu, Huamin Qu
DOI: 10.1109/TVCG.2023.3236380
Room: 109
2023-10-25T22:36:00ZGMT-0600Change your timezone on the schedule page
2023-10-25T22:36:00Z
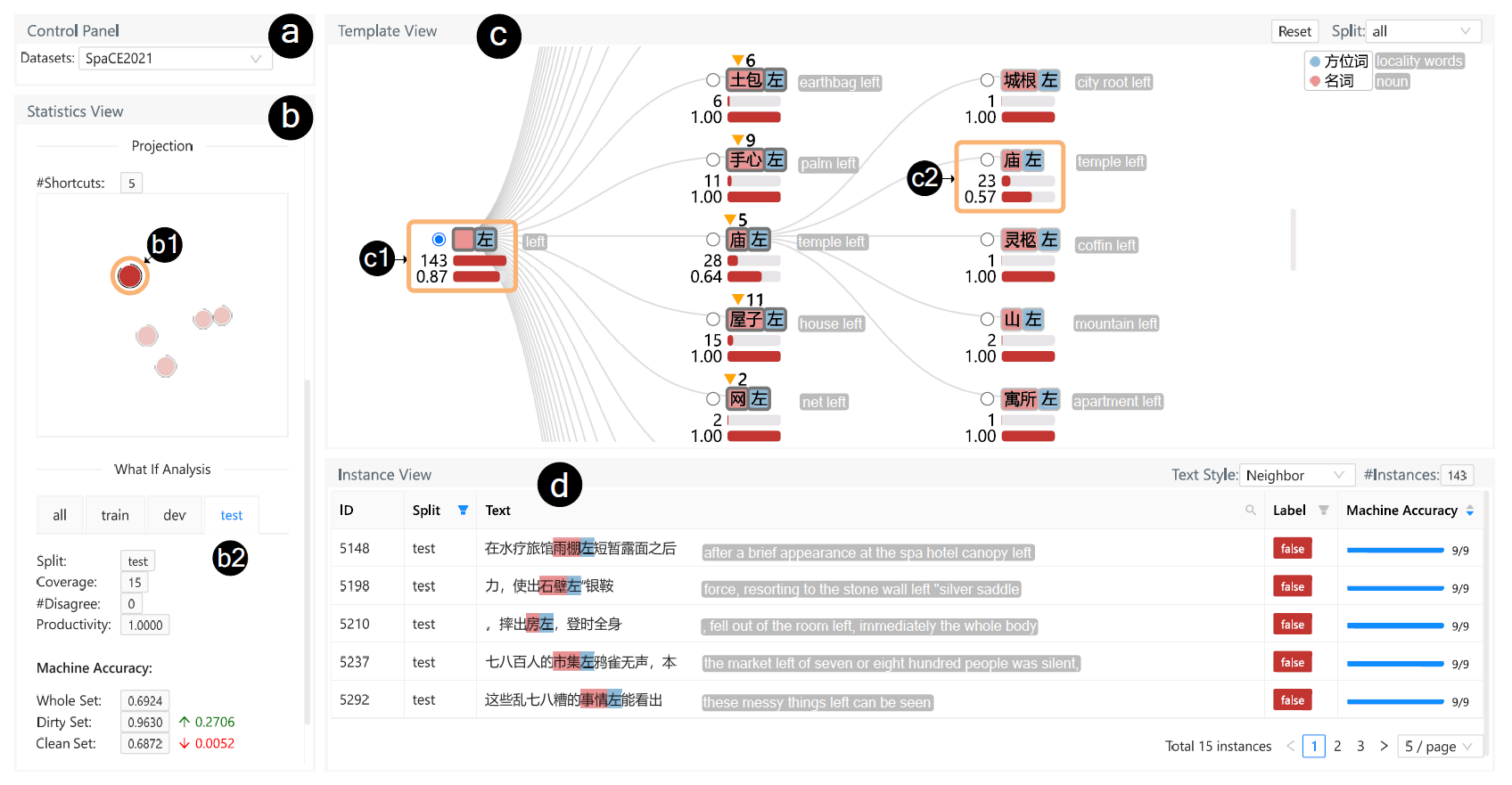
Fast forward
Full Video
Keywords
Visual Analytics;Natural Language Understanding;Shortcut
Abstract
Benchmark datasets play an important role in evaluating Natural Language Understanding (NLU) models. However, shortcuts—unwanted biases in the benchmark datasets—can damage the effectiveness of benchmark datasets in revealing models’ real capabilities. Since shortcuts vary in coverage, productivity, and semantic meaning, it is challenging for NLU experts to systematically understand and avoid them when creating benchmark datasets. In this paper, we develop a visual analytics system, ShortcutLens, to help NLU experts explore shortcuts in NLU benchmark datasets. The system allows users to conduct multi-level exploration of shortcuts. Specifically, Statistics View helps users grasp the statistics such as coverage and productivity of shortcuts in the benchmark dataset. Template View employs hierarchical and interpretable templates to summarize different types of shortcuts. Instance View allows users to check the corresponding instances covered by the shortcuts. We conduct case studies and expert interviews to evaluate the effectiveness and usability of the system. The results demonstrate that ShortcutLens supports users in gaining a better understanding of benchmark dataset issues through shortcuts, inspiring them to create challenging and pertinent benchmark datasets.