Visual Explanation for Open-domain Question Answering with BERT
Zekai Shao, Shuran Sun, Yuheng Zhao, Siyuan Wang, Zhongyu Wei, Tao Gui, Cagatay Turkay, Siming Chen
DOI: 10.1109/TVCG.2023.3243676
Room: 106
2023-10-25T00:09:00ZGMT-0600Change your timezone on the schedule page
2023-10-25T00:09:00Z
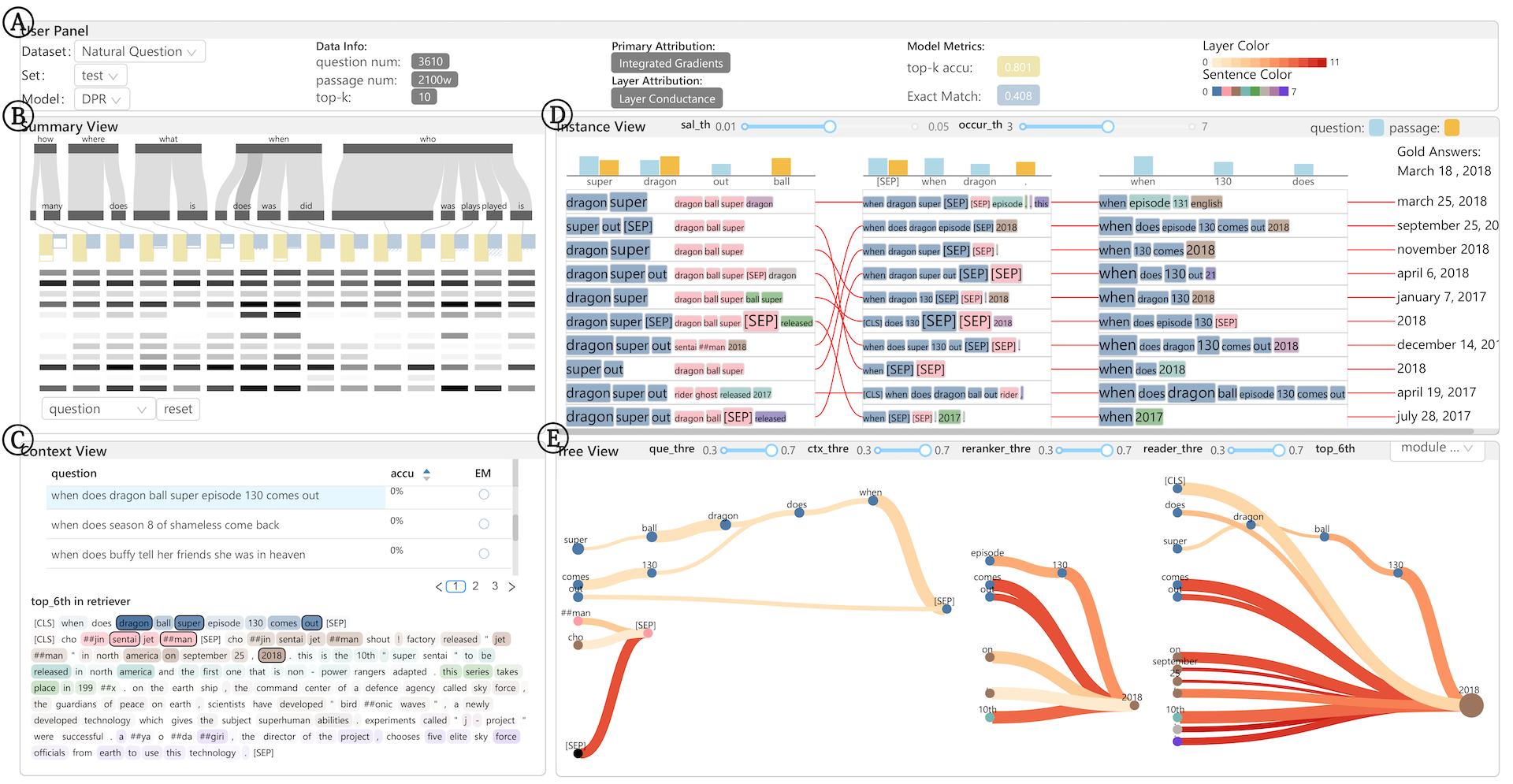
Fast forward
Full Video
Keywords
Open-domain Question Answering;Explainable Machine Learning;Visual Analytics
Abstract
Open-domain question answering (OpenQA) is an essential but challenging task in natural language processing that aims to answer questions in natural language formats on the basis of large-scale unstructured passages. Recent research has taken the performance of benchmark datasets to new heights, especially when these datasets are combined with techniques for machine reading comprehension based on Transformer models. However, as identified through our ongoing collaboration with domain experts and our review of literature, three key challenges limit their further improvement: (i) complex data with multiple long texts, (ii) complex model architecture with multiple modules, and (iii) semantically complex decision process. In this paper, we present VEQA, a visual analytics system that helps experts understand the decision reasons of OpenQA and provides insights into model improvement. The system summarizes the data flow within and between modules in the OpenQA model as the decision process takes place at the summary, instance and candidate levels. Specifically, it guides users through a summary visualization of dataset and module response to explore individual instances with a ranking visualization that incorporates context. Furthermore, VEQA supports fine-grained exploration of the decision flow within a single module through a comparative tree visualization. We demonstrate the effectiveness of VEQA in promoting interpretability and providing insights into model enhancement through a case study and expert evaluation.