DMiner: Dashboard Design Mining and Recommendation
Yanna Lin, Haotian Li, Aoyu Wu, Yong Wang, Huamin Qu
DOI: 10.1109/TVCG.2023.3251344
Room: 103
2023-10-25T03:00:00ZGMT-0600Change your timezone on the schedule page
2023-10-25T03:00:00Z
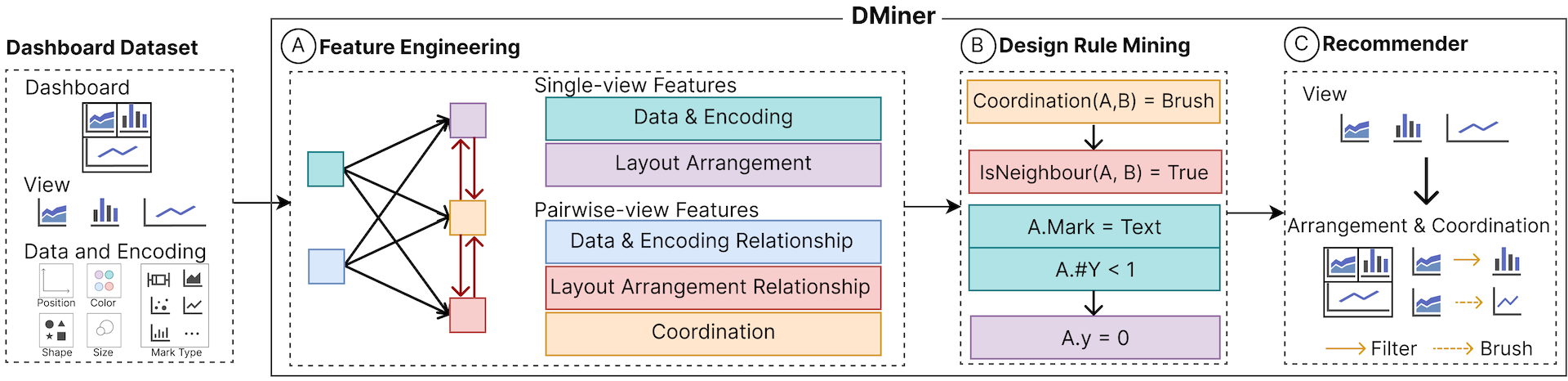
Fast forward
Full Video
Keywords
Design Mining;Visualization Recommendation;Multiple-view Visualization;Dashboards
Abstract
Dashboards, which comprise multiple views on a single display, help analyze and communicate multiple perspectives of data simultaneously. However, creating effective and elegant dashboards is challenging since it requires careful and logical arrangement and coordination of multiple visualizations. To solve the problem, we propose a data-driven approach for mining design rules from dashboards and automating dashboard organization. Specifically, we focus on two prominent aspects of the organization: arrangement, which describes the position, size, and layout of each view in the display space; and coordination, which indicates the interaction between pairwise views. We build a new dataset containing 854 dashboards crawled online, and develop feature engineering methods for describing the single views and view-wise relationships in terms of data, encoding, layout, and interactions. Further, we identify design rules among those features and develop a recommender for dashboard design. We demonstrate the usefulness of DMiner through an expert study and a user study. The expert study shows that our extracted design rules are reasonable and conform to the design practice of experts. Moreover, a comparative user study shows that our recommender could help automate dashboard organization and reach human-level performance. In summary, our work offers a promising starting point for design mining visualizations to build recommenders.