FS/DS: A Theoretical Framework for the Dual Analysis of Feature Space and Data Space
Frederik L. Dennig, Matthias Miller, Daniel A. Keim, Mennatallah El-Assady
DOI: 10.1109/TVCG.2023.3288356
Room: 106
Tuesday, October 24th, 2023 @ 22:36GMT+00:00Change your timezone on the schedule page
2 years agoYour current time: Sunday, Jun 22nd @ 22:14
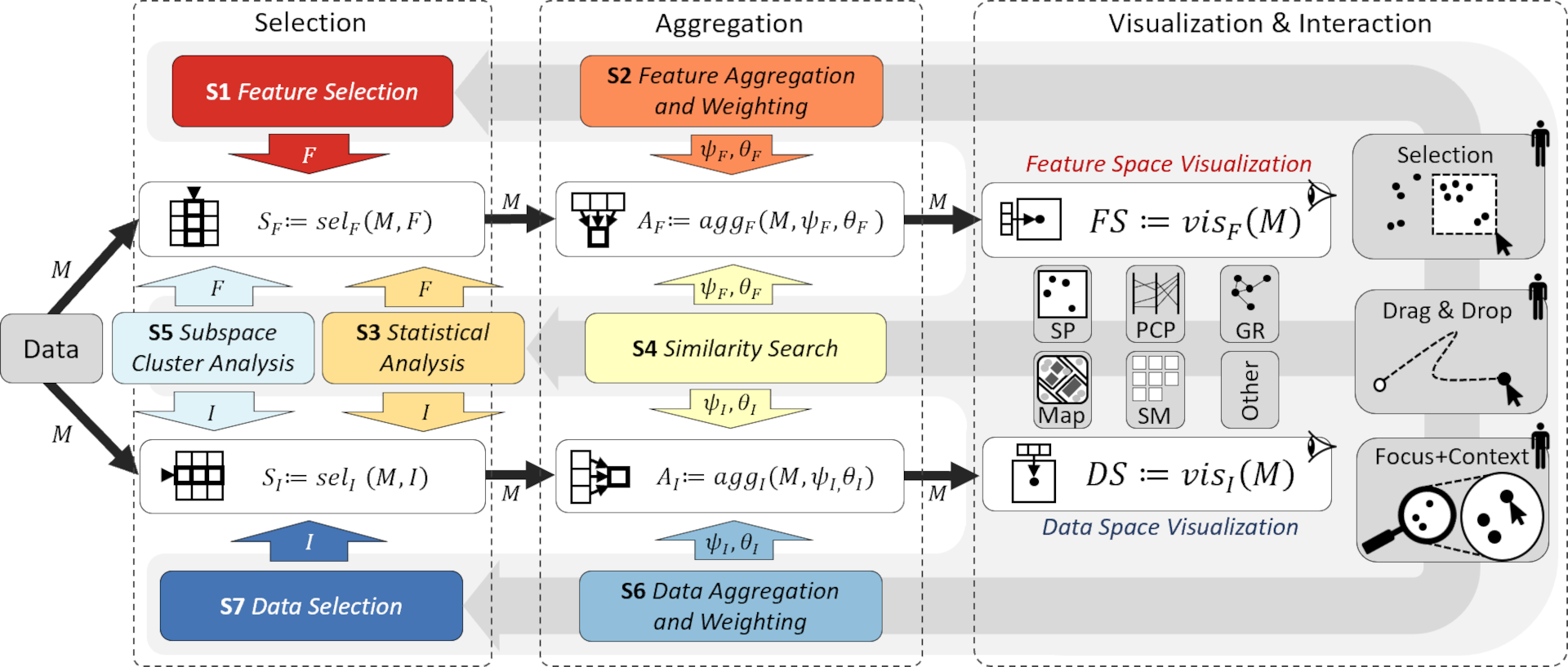
Fast forward
Full Video
Keywords
Visual analytics;dual analysis;feature space;data space;feature exploration;mixed data;high-dimensional data
Abstract
With the surge of data-driven analysis techniques, there is a rising demand for enhancing the exploration of large high-dimensional data by enabling interactions for the joint analysis of features (i.e., dimensions). Such a dual analysis of the feature space and data space is characterized by three components, (1) a view visualizing feature summaries, (2) a view that visualizes the data records, and (3) a bidirectional linking of both plots triggered by human interaction in one of both visualizations, e.g., Linking & Brushing. Dual analysis approaches span many domains, e.g., medicine, crime analysis, and biology. The proposed solutions encapsulate various techniques, such as feature selection or statistical analysis. However, each approach establishes a new definition of dual analysis. To address this gap, we systematically reviewed published dual analysis methods to investigate and formalize the key elements, such as the techniques used to visualize the feature space and data space, as well as the interaction between both spaces. From the information elicited during our review, we propose a unified theoretical framework for dual analysis, encompassing all existing approaches extending the field. We apply our proposed formalization describing the interactions between each component and relate them to the addressed tasks. Additionally, we categorize the existing approaches using our framework and derive future research directions to advance dual analysis by including state-of-the-art visual analysis techniques to improve data exploration.