CoordNet: Data Generation and Visualization Generation for Time-Varying Volumes via a Coordinate-Based Neural Network
Jun Han, Chaoli Wang
DOI: 10.1109/TVCG.2022.3197203
Room: 106
2023-10-25T23:45:00ZGMT-0600Change your timezone on the schedule page
2023-10-25T23:45:00Z
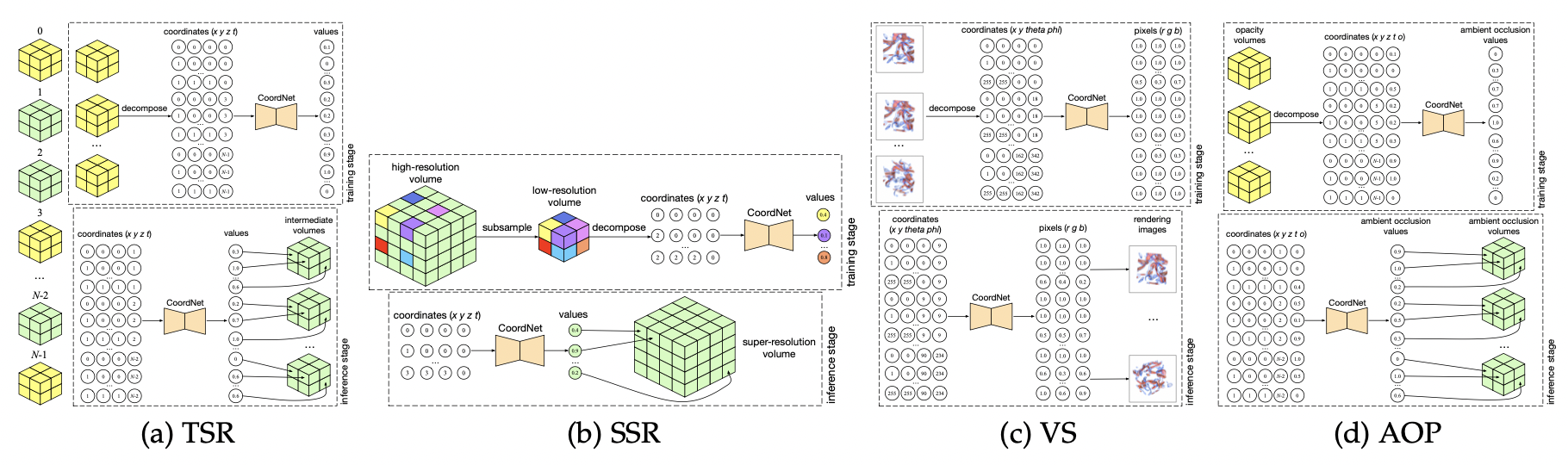
Fast forward
Full Video
Keywords
Volume visualization;implicit neural representation;data generation;visualization generation
Abstract
Although deep learning has demonstrated its capability in solving diverse scientific visualization problems, it still lacks generalization power across different tasks. To address this challenge, we propose CoordNet, a single coordinate-based framework that tackles various tasks relevant to time-varying volumetric data visualization without modifying the network architecture. The core idea of our approach is to decompose diverse task inputs and outputs into a unified representation (i.e., coordinates and values) and learn a function from coordinates to their corresponding values. We achieve this goal using a residual block-based implicit neural representation architecture with periodic activation functions. We evaluate CoordNet on data generation (i.e., temporal super-resolution and spatial super-resolution) and visualization generation (i.e., view synthesis and ambient occlusion prediction) tasks using time-varying volumetric data sets of various characteristics. The experimental results indicate that CoordNet achieves better quantitative and qualitative results than the state-of-the-art approaches across all the evaluated tasks.