VisRecall: Quantifying Information Visualisation Recallability via Question Answering
Yao Wang, Chuhan Jiao, Mihai Bâce, Andreas Bulling
DOI: 10.1109/TVCG.2022.3198163
Room: 105
2023-10-25T23:45:00ZGMT-0600Change your timezone on the schedule page
2023-10-25T23:45:00Z
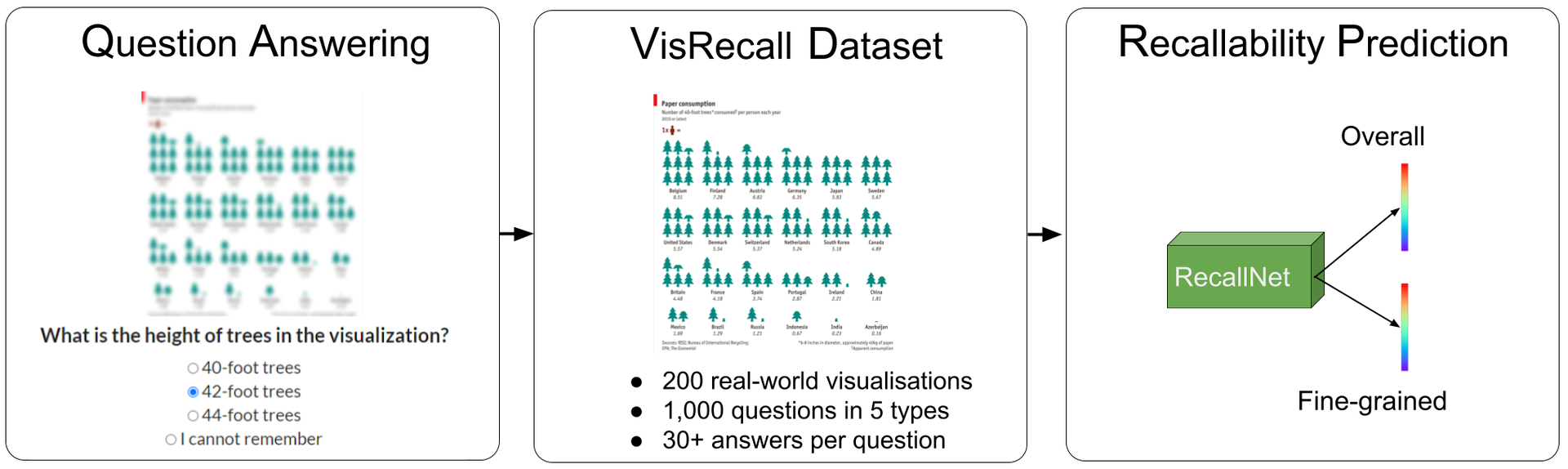
Fast forward
Full Video
Keywords
Information visualisation;machine learning;memorability;recallability
Abstract
Despite its importance for assessing the effectiveness of communicating information visually, fine-grained recallability of information visualisations has not been studied quantitatively so far. In this work, we propose a question-answering paradigm to study visualisation recallability and present VisRecall - a novel dataset consisting of 200 visualisations that are annotated with crowd-sourced human (N = 305) recallability scores obtained from 1,000 questions of five question types. Furthermore, we present the first computational method to predict recallability of different visualisation elements, such as the title or specific data values. We report detailed analyses of our method on VisRecall and demonstrate that it outperforms several baselines in overall recallability and FE-, F-, RV-, and U-question recallability. Our work makes fundamental contributions towards a new generation of methods to assist designers in optimising visualisations.