Principal Geodesic Analysis of Merge Trees (and Persistence Diagrams)
Mathieu Pont, Jules Vidal, Julien Tierny
DOI: 10.1109/TVCG.2022.3215001
Room: 106
2023-10-26T03:24:00ZGMT-0600Change your timezone on the schedule page
2023-10-26T03:24:00Z
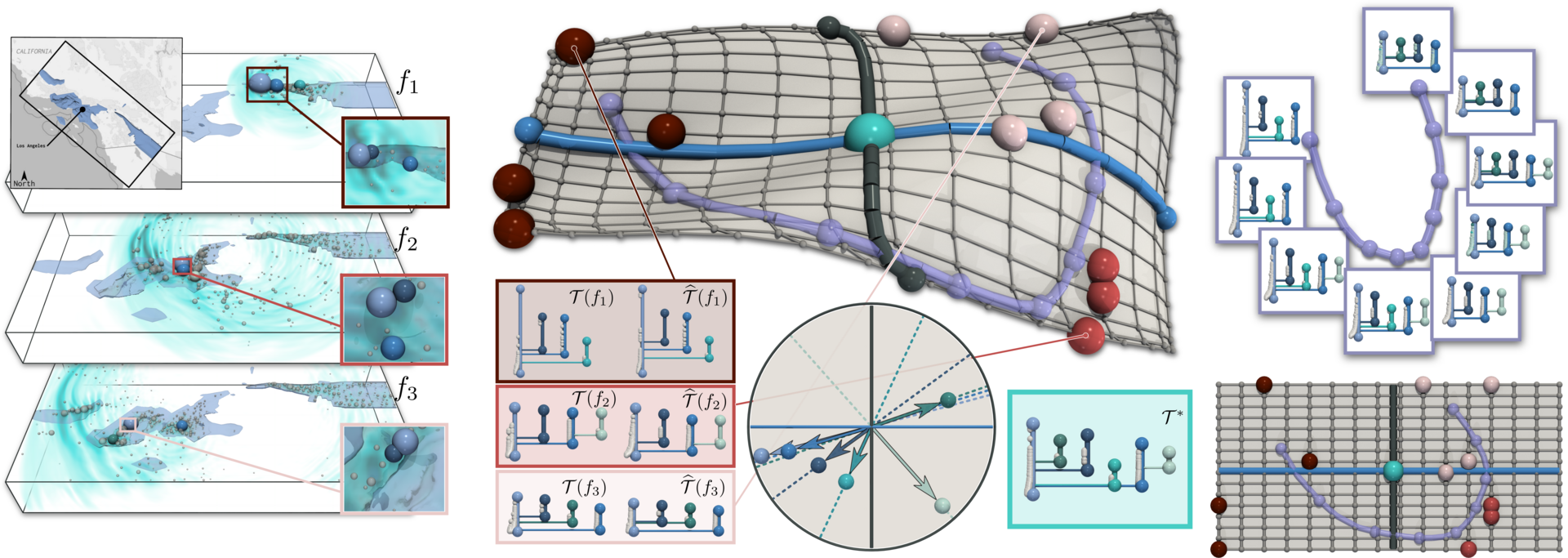
Fast forward
Full Video
Keywords
Topological data analysis;ensemble data;merge trees;persistence diagrams
Abstract
This paper presents a computational framework for the Principal Geodesic Analysis of merge trees (MT-PGA), a novel adaptation of the celebrated Principal Component Analysis (PCA) framework [87] to the Wasserstein metric space of merge trees [92]. We formulate MT-PGA computation as a constrained optimization problem, aiming at adjusting a basis of orthogonal geodesic axes, while minimizing a fitting energy. We introduce an efficient, iterative algorithm which exploits shared-memory parallelism, as well as an analytic expression of the fitting energy gradient, to ensure fast iterations. Our approach also trivially extends to extremum persistence diagrams. Extensive experiments on public ensembles demonstrate the efficiency of our approach - with MT-PGA computations in the orders of minutes for the largest examples. We show the utility of our contributions by extending to merge trees two typical PCA applications. First, we apply MT-PGA to data reduction and reliably compress merge trees by concisely representing them by their first coordinates in the MT-PGA basis. Second, we present a dimensionality reduction framework exploiting the first two directions of the MT-PGA basis to generate two-dimensional layouts of the ensemble. We augment these layouts with persistence correlation views, enabling global and local visual inspections of the feature variability in the ensemble. In both applications, quantitative experiments assess the relevance of our framework. Finally, we provide a C++ implementation that can be used to reproduce our results.