GraphDecoder: Recovering Diverse Network Graphs from Visualization Images via Attention-Aware Learning
Sicheng Song, Chenhui Li, Dong Li, Jaunting Chen, Changbo Wang
DOI: 10.1109/TVCG.2022.3225554
Room: 105
2023-10-25T05:09:00ZGMT-0600Change your timezone on the schedule page
2023-10-25T05:09:00Z
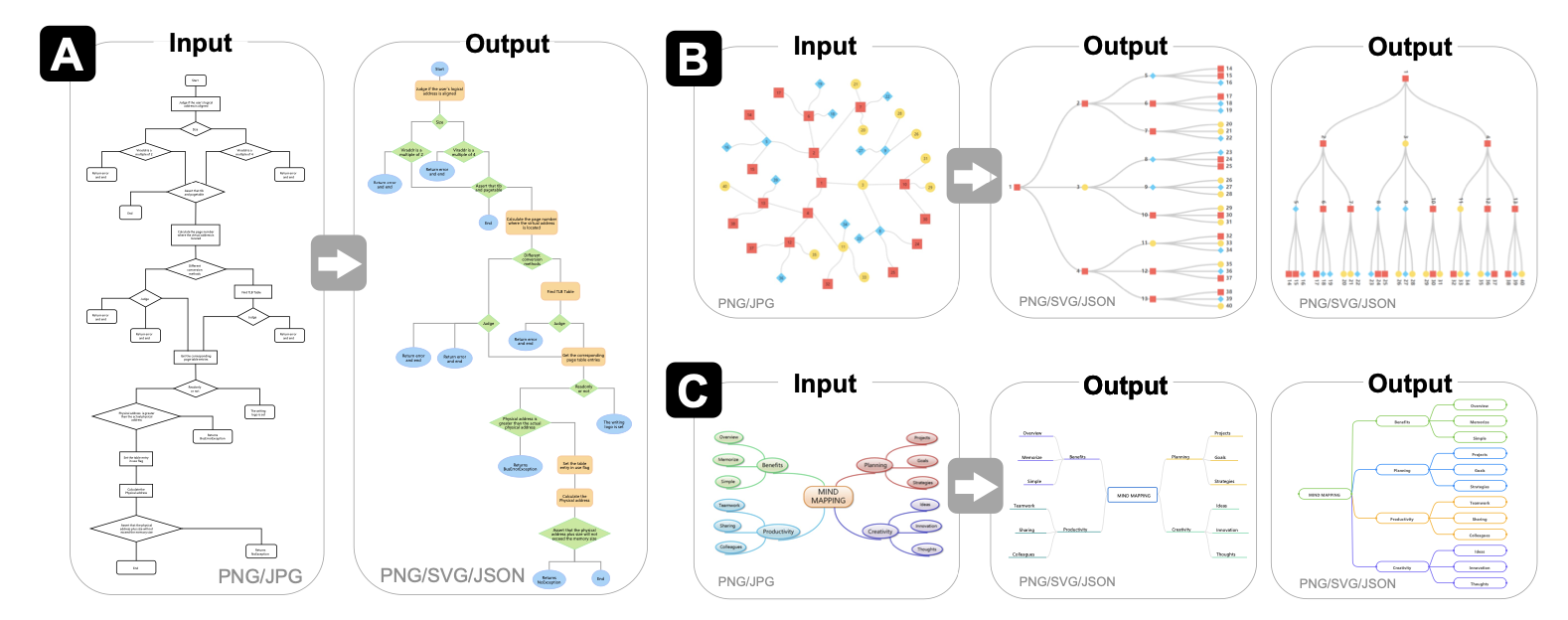
Fast forward
Full Video
Keywords
Information visualization;Chart mining;Semantic segmentation;Network graph;Attention mechanism
Abstract
DNGs are diverse network graphs with texts and different styles of nodes and edges, including mind maps, modeling graphs, and flowcharts. They are high-level visualizations that are easy for humans to understand but difficult for machines. Inspired by the process of human perception of graphs, we propose a method called GraphDecoder to extract data from raster images. Given a raster image, we extract the content based on a neural network. We built a semantic segmentation network based on U-Net. We increase the attention mechanism module, simplify the network model, and design a specific loss function to improve the model's ability to extract graph data. After this semantic segmentation network, we can extract the data of all nodes and edges. We then combine these data to obtain the topological relationship of the entire DNG. We also provide an interactive interface for users to redesign the DNGs. We verify the effectiveness of our method by evaluations and user studies on datasets collected on the Internet and generated datasets.