An Image-based Exploration and Query System for Large Visualization Collections via Neural Image Embedding
Yilin Ye, Rong Huang, Wei Zeng
DOI: 10.1109/TVCG.2022.3229023
Room: 103
2023-10-24T22:12:00ZGMT-0600Change your timezone on the schedule page
2023-10-24T22:12:00Z
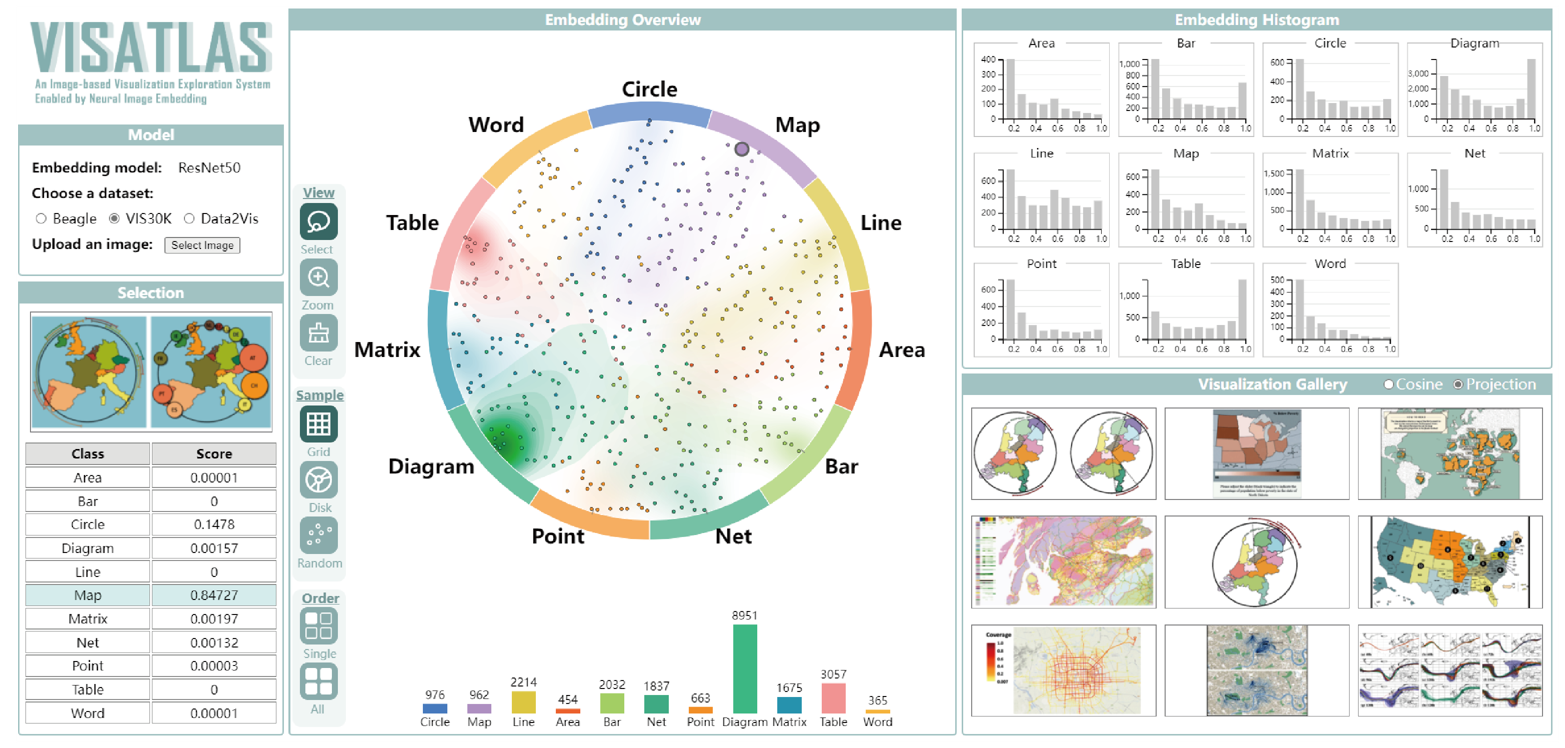
Fast forward
Full Video
Keywords
Visualization collection;image embedding;visual query;image visualization;design pattern
Abstract
High-quality visualization collections are beneficial for a variety of applications including visualization reference and data-driven visualization design. The visualization community has created many visualization collections, and developed interactive exploration systems for the collections. However, the systems are mainly based on extrinsic attributes like authors and publication years, whilst neglect intrinsic property ( i.e ., visual appearance) of visualizations, hindering visual comparison and query of visualization designs. This paper presents VISAtlas , an image-based approach empowered by neural image embedding, to facilitate exploration and query for visualization collections. To improve embedding accuracy, we create a comprehensive collection of synthetic and real-world visualizations, and use it to train a convolutional neural network (CNN) model with a triplet loss for taxonomical classification of visualizations. Next, we design a coordinated multiple view (CMV) system that enables multi-perspective exploration and design retrieval based on visualization embeddings. Specifically, we design a novel embedding overview that leverages contextual layout framework to preserve the context of the embedding vectors with the associated visualization taxonomies, and density plot and sampling techniques to address the overdrawing problem. We demonstrate in three case studies and one user study the effectiveness of VISAtlas in supporting comparative analysis of visualization collections, exploration of composite visualizations, and image-based retrieval of visualization designs. The studies reveal that real-world visualization collections ( e.g ., Beagle and VIS30K) better accord with the richness and diversity of visualization designs than synthetic collections ( e.g ., Data2Vis), inspiring composite visualizations are identified in real-world collections, and distinct design patterns exist in visualizations from different sources.