A Vega-Lite Dataset and Natural Language Generation Pipeline with Large Language Models
Hyung-Kwon Ko, Hyeon Jeon, Gwanmo Park, Dae Hyun Kim, Nam Wook Kim, Juho Kim, Jinwook Seo
Room: 110
2023-10-22T03:00:00ZGMT-0600Change your timezone on the schedule page
2023-10-22T03:00:00Z
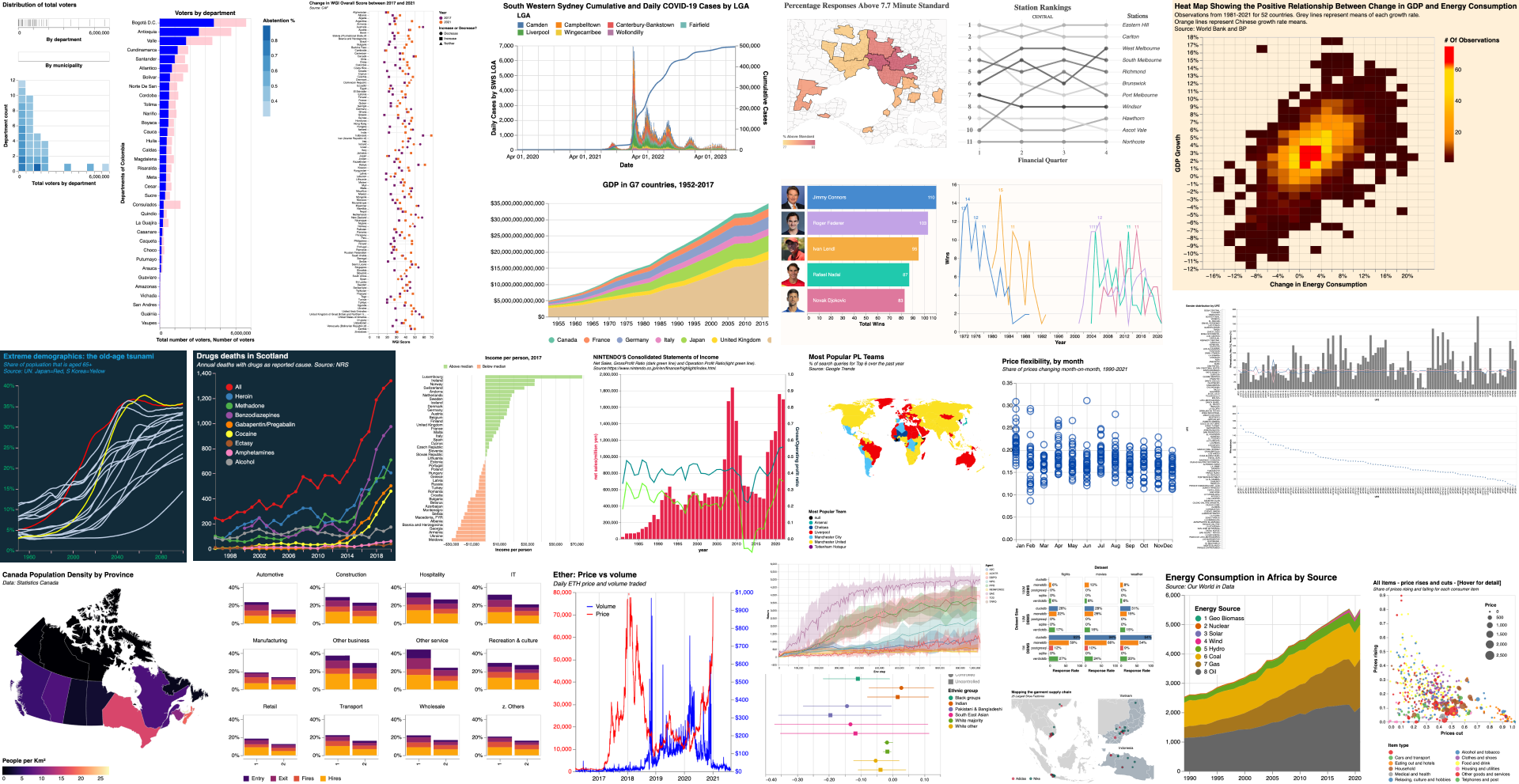
Fast forward
Full Video
Abstract
There is a growing trend of utilizing Visualization-oriented Natural Language Interfaces (V-NLIs) to author charts. However, researchers consistently highlight the lack of high-quality chart and natural language datasets, which impedes the development of more sophisticated and data-driven systems using V-NLIs. In this study, we present a meticulously curated collection of human-generated 1,981 Vega-Lite specifications, derived from real-world data, and use Large Language Models (LLMs) for generating natural language queries for chart generation tasks. Unlike previous datasets that relied on relatively simple and homogeneous templates, our Vega-Lite dataset contains more complex and diverse (i.e., varying interactions, multiple plots/views, and different chart types). Using this dataset, we demonstrate generating natural language queries for chart generation, and how the results can be different when different input types are used (e.g., Vega-Lite, Image, both Vega-Lite and Image).